AI 简报 -GAN 和 CGAN
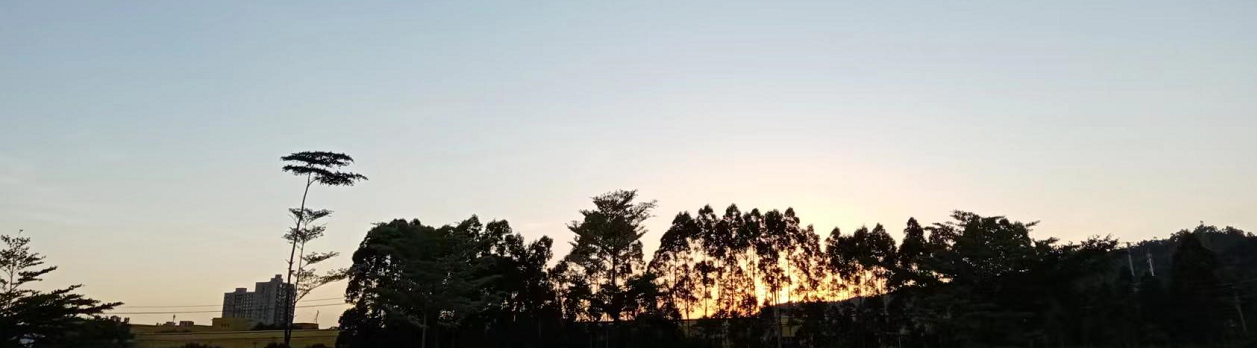
1.GAN 基础
Generative Adversarial Nets 是 GAN 的开山之作,通过对抗的方式,去学习数据分布的生成式模型。GAN 是无监督的过程,能够捕捉数据集的分布,以便于可以从随机噪声中生成同样分布的数据。
GAN 涉及两个模型,一个是 D 判别式模型和 G 生成式模型
D 判别式模型:学习真假边界,判断数据是真的还是假的
G 生成式模型:学习数据分布并生成数据
所谓对抗是指生成器 G 不断的生成数据让判别器 D 进行判断,一开始生成器 G 生成的数据很容易就被判别器揪出来,但是随着生成器 G 学会了判别器 D 的判别一些标准或者特征,也学习了这些特征生成新的数据,再次让判别器判断。如此迭代,直到生成器 G 生成的数据,判别器无法判断真假,以假乱真的情况下,生成器 G 就出师了。
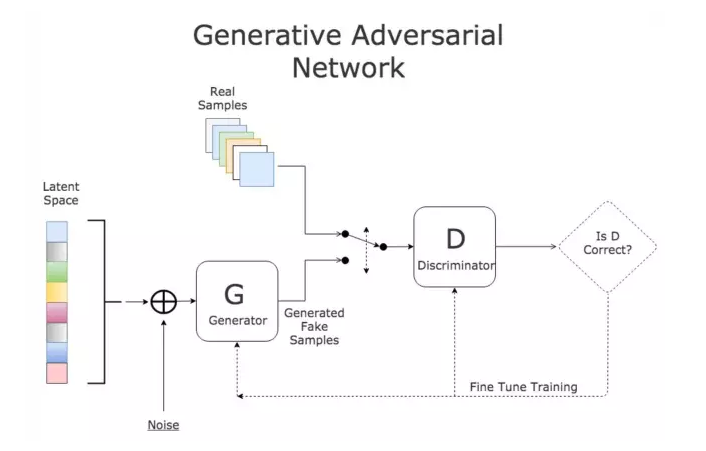
GAN 经典的 loss 如下(minmax 体现的就是对抗)

训练过程:
首先先训练多轮的判别器 D:生成器 G 不变,使得判别器 D,能够正确区分真假(真的数据是要学习分布的数据,假的数据是随机分布通常是正态分布的数据),从 loss 看就是最大化真的数据和最大化 1-假的数据
在训练生成器 G:判别器不变,原文是最大化假的 loss
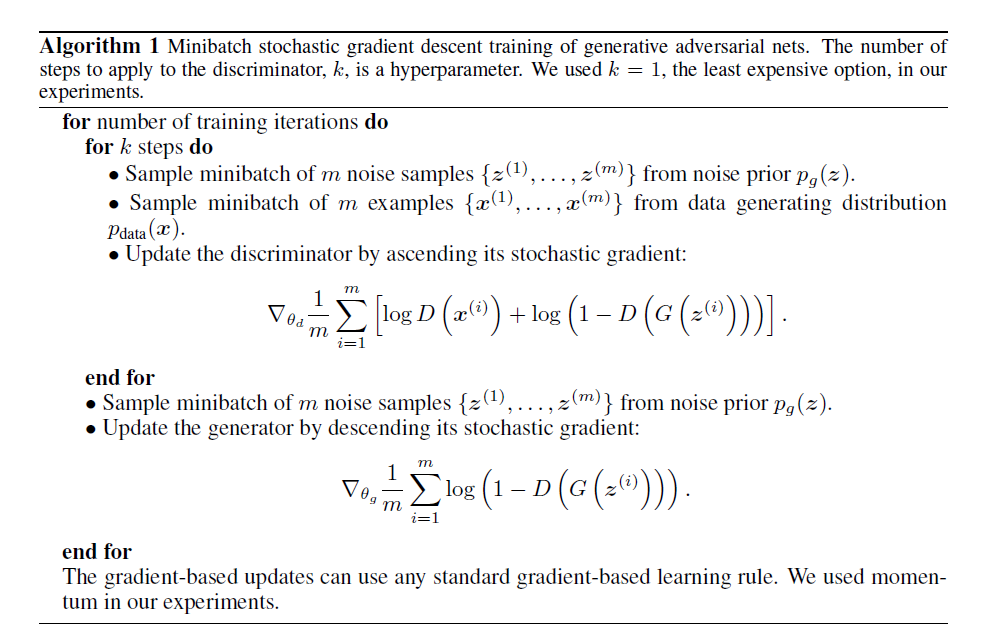
结果展示:
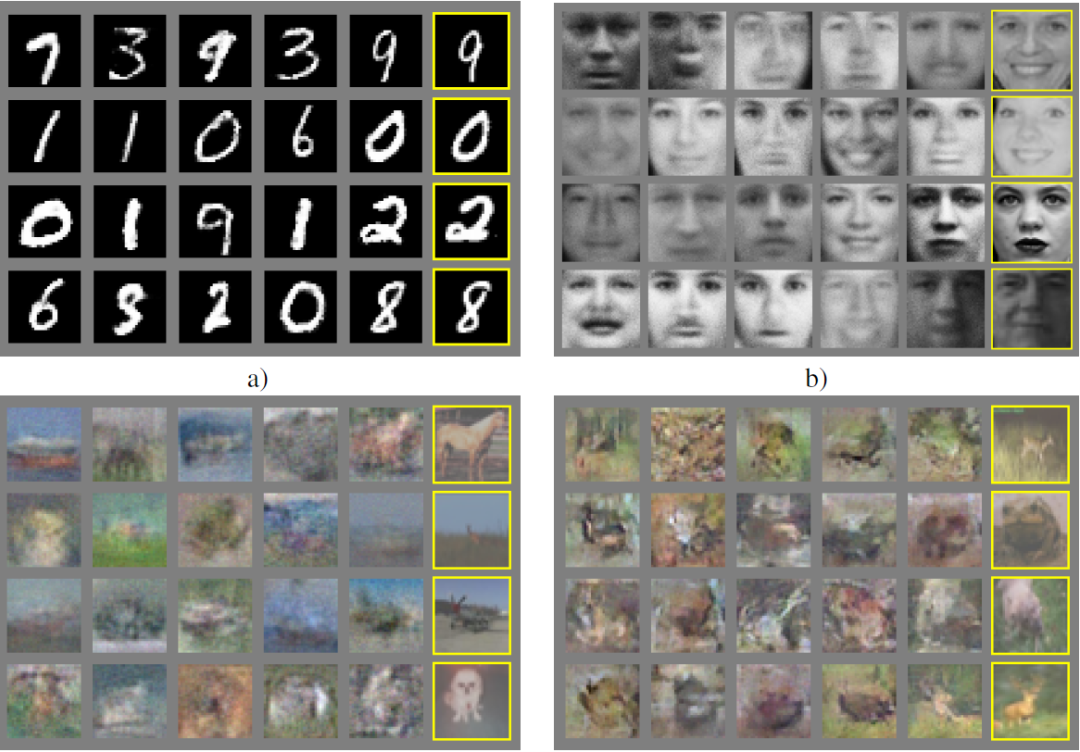
2.CGAN 介绍
GAN 的目的是学习输入数据的分布特征,然后再根据这个特征根据随机噪声输入生成相同分布的数据。理想情况下,GAN 可以生成与训练数据相似的数据,但是每次生成的图像是不受控制的。CGAN 是指在 GAN 上加一个条件,让 GAN 的生成受这个输入条件的影响。比如在论文中Conditional Generative Adversarial Nets
加入的条件是希望生成的类别的标签; 当然这个这个条件可以是文本,图片等。
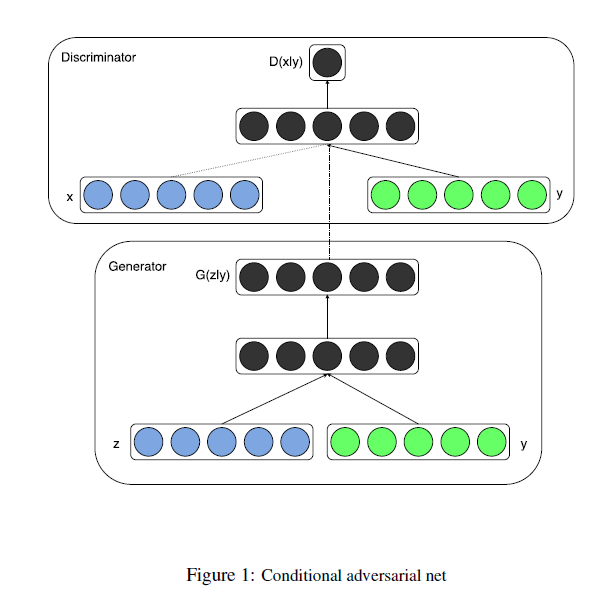
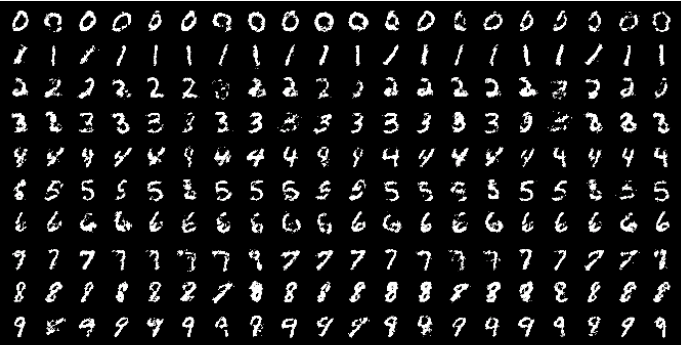
条件文本:Text-to-Image 根据文本来生成对应的图片
条件是图片:Image-to-Image (pix2pix: Image-to-Image Translation with Conditional Adversarial Networks):如下:
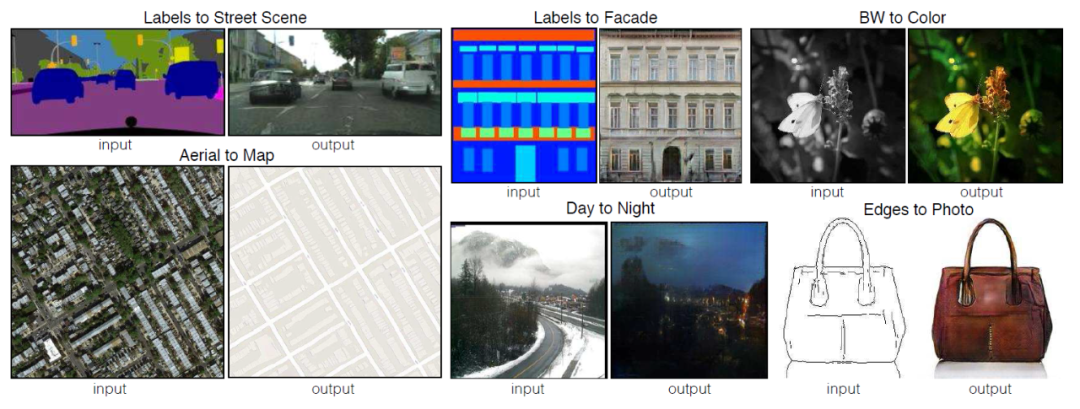
可见,CGAN 是属于监督学习的过程,需要 paired data。
2.1 CGAN 实现说明
CGAN 要求 paired 数据, 判别器和生成器都比 GAN 多一个 condition。实现的时候只要把这个 condition 以 concat 的方式加到原来噪声输入即可。见下面李宏毅先生的课件:
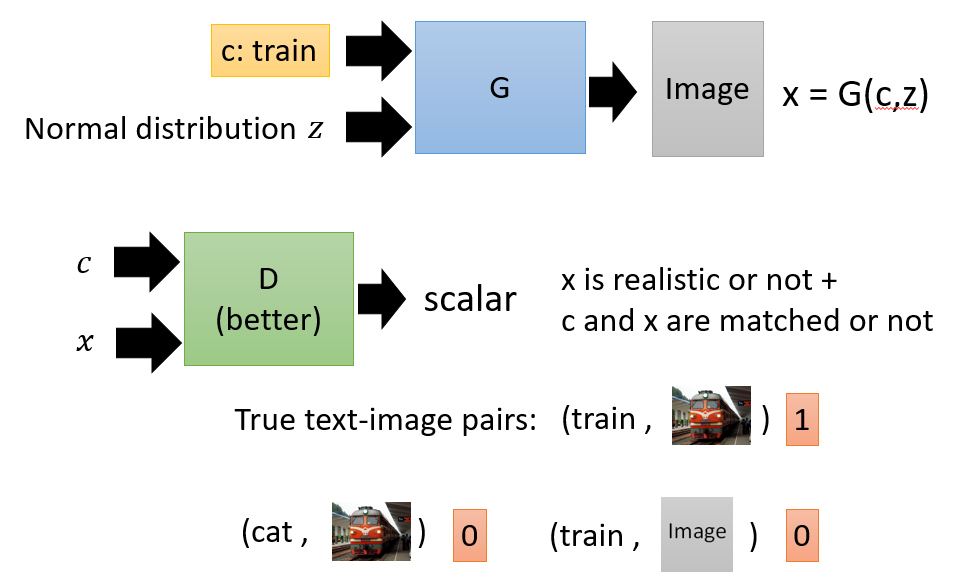
生成器
判别器
train
2.2 CGAN 的应用
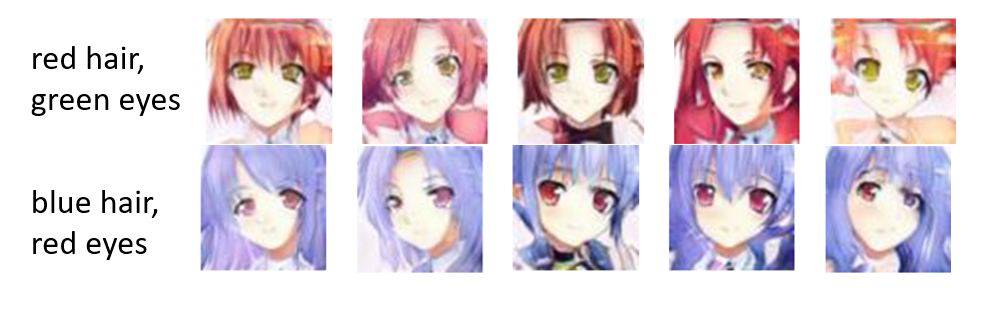
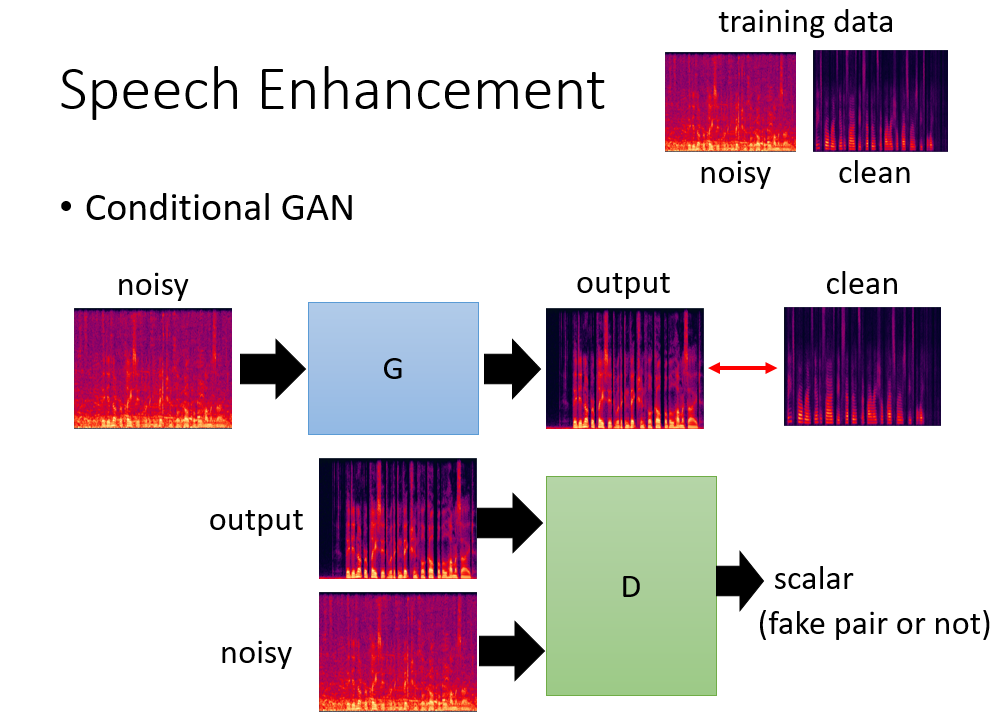
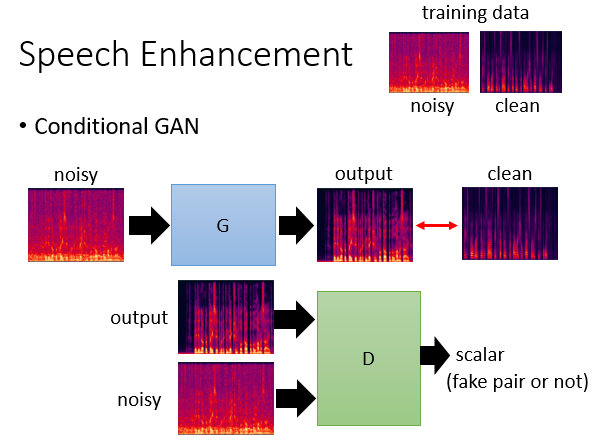
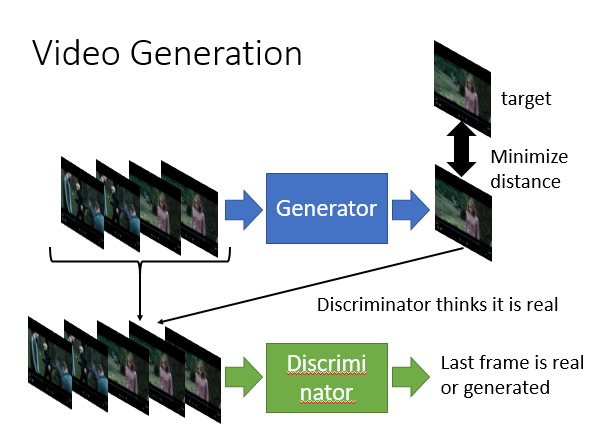
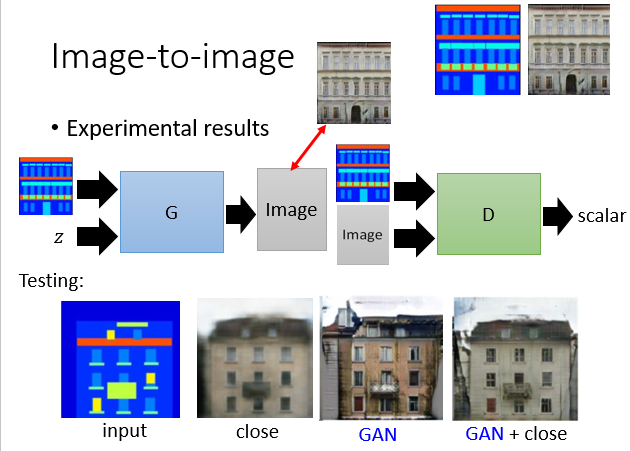
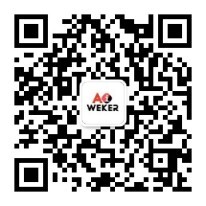
版权声明: 本文为 InfoQ 作者【AIWeker-人工智能微客】的原创文章。
原文链接:【http://xie.infoq.cn/article/9a78b053d4fd80361f6d592e1】。文章转载请联系作者。
评论