AI 简报 -Image Colorization 调研
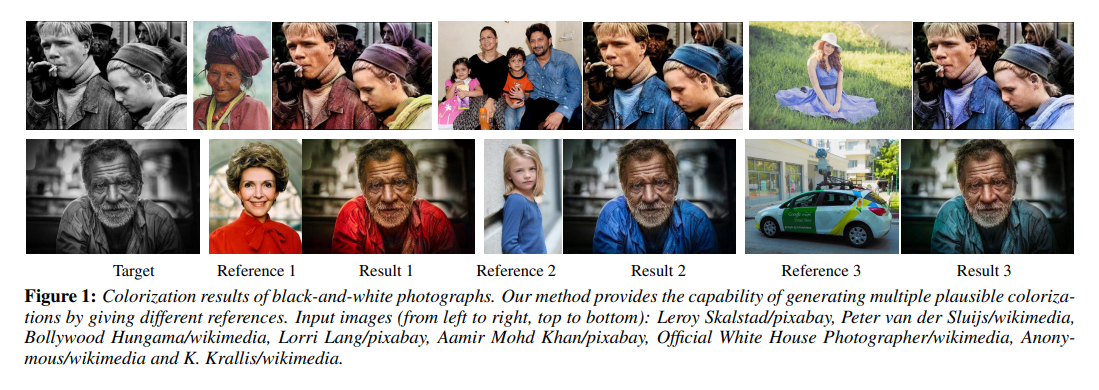
1.问题描述
估计一个灰度图(gray image)到彩色图(RGB image)的映射
一个灰度图的在彩色空间(Lab/YUV)中估计彩色的通道(ab or uv)
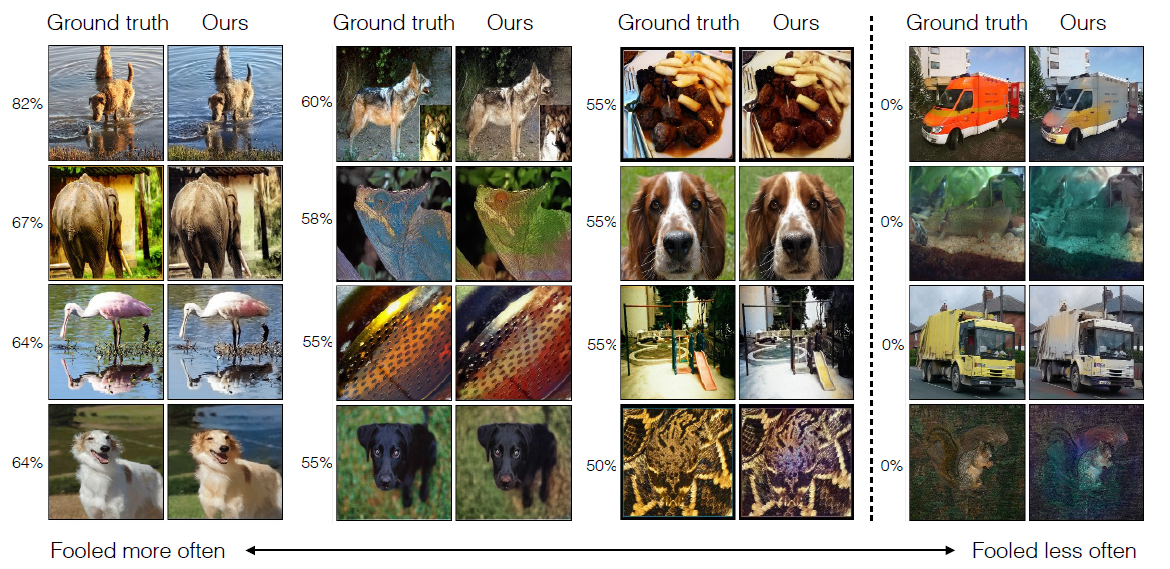
2.图像概述
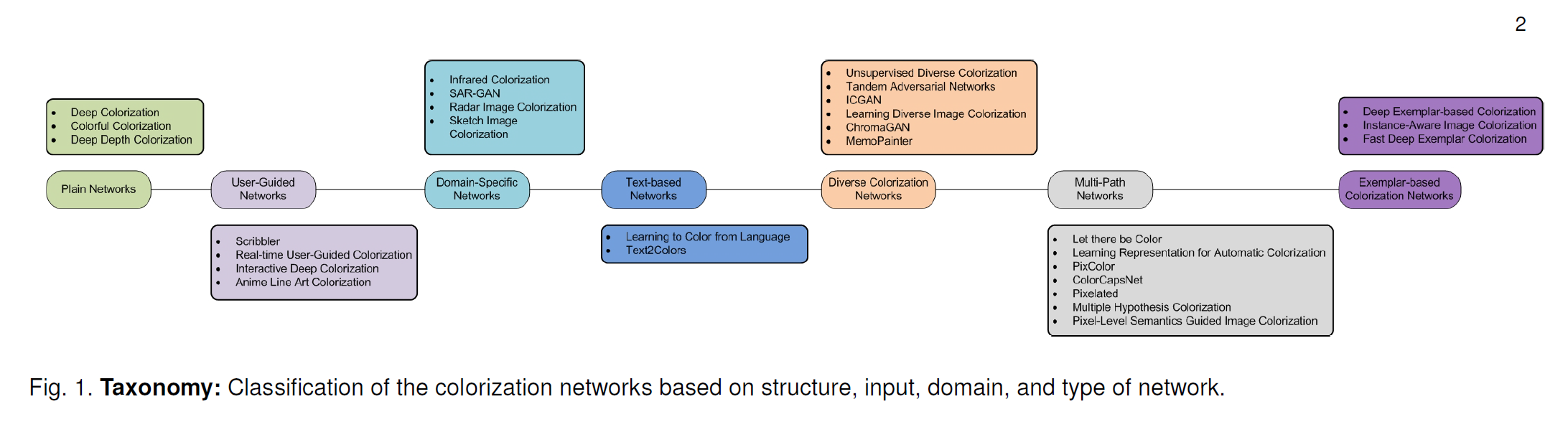
Plain Networks 无先验知识,CNN 架构,去估计彩色空间, 主要在于 loss 的设计
User-Guided Networks
需要用户输入额外的一些信息来指导或者需要用户的交互
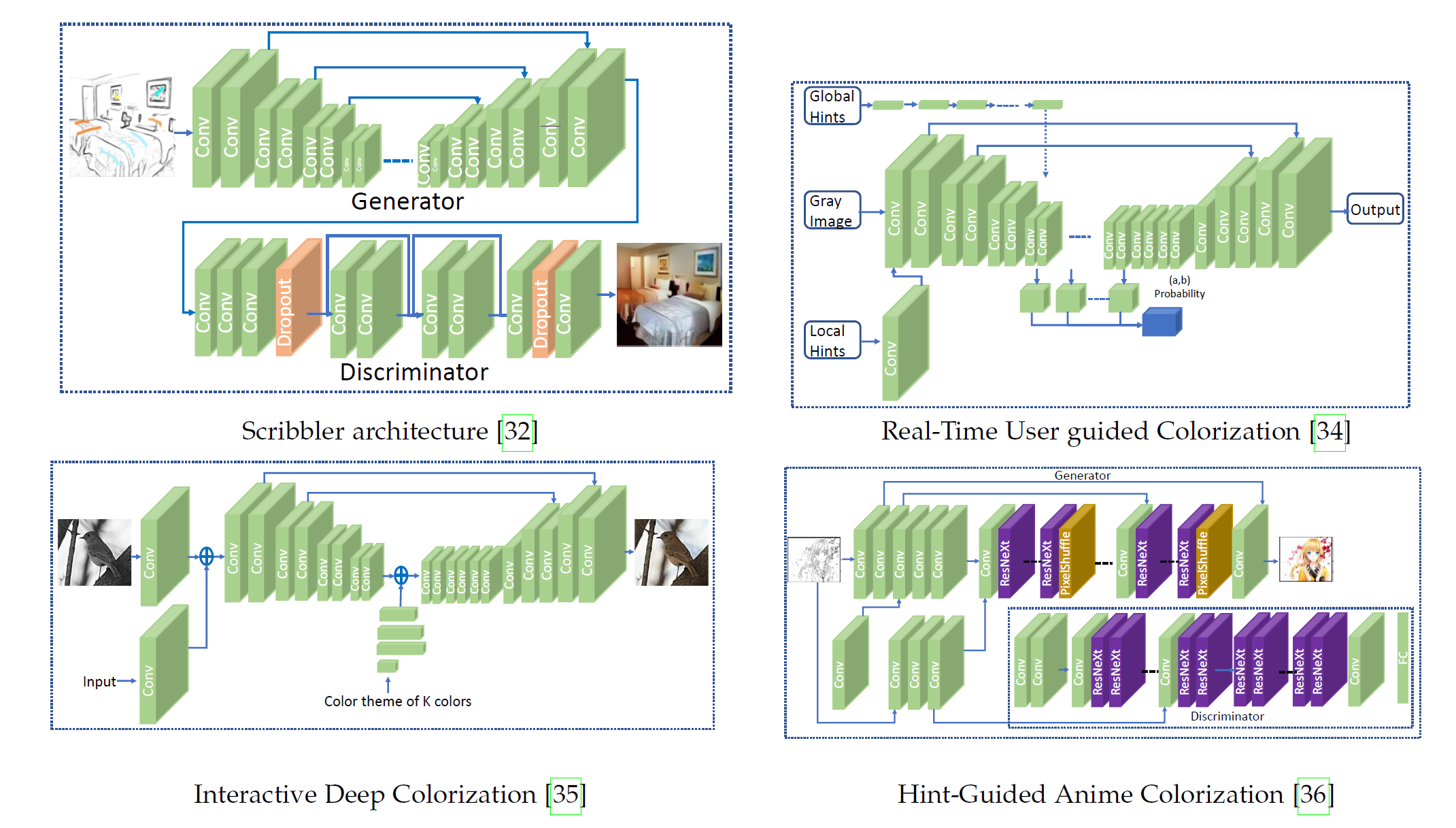
Domain-Specific Networks 有领域特性的图片,如红外线的黑白图,雷达图等
Text-Based Networks 参考的是文本,例如蓝色的车
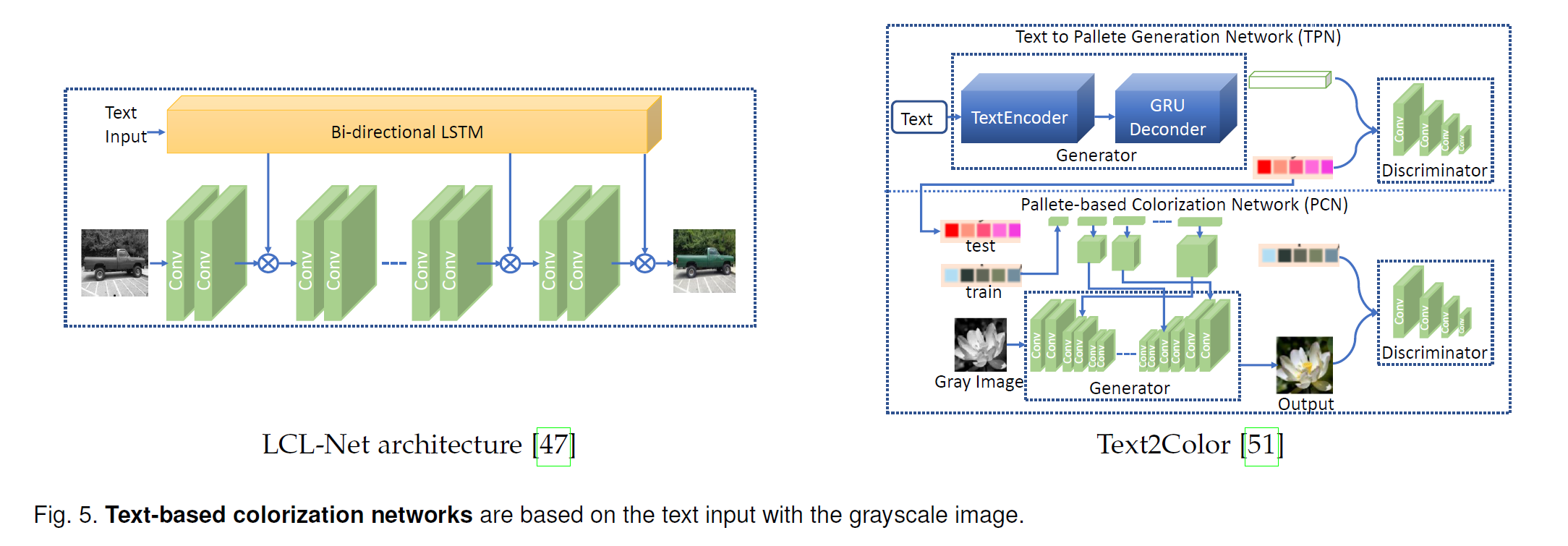
Diverse Colorization Networks 目标是生成多样性的彩色图片,车有不同的颜色。基本上 GAN 的方法,不要求和原图一样,只要看起来真实。
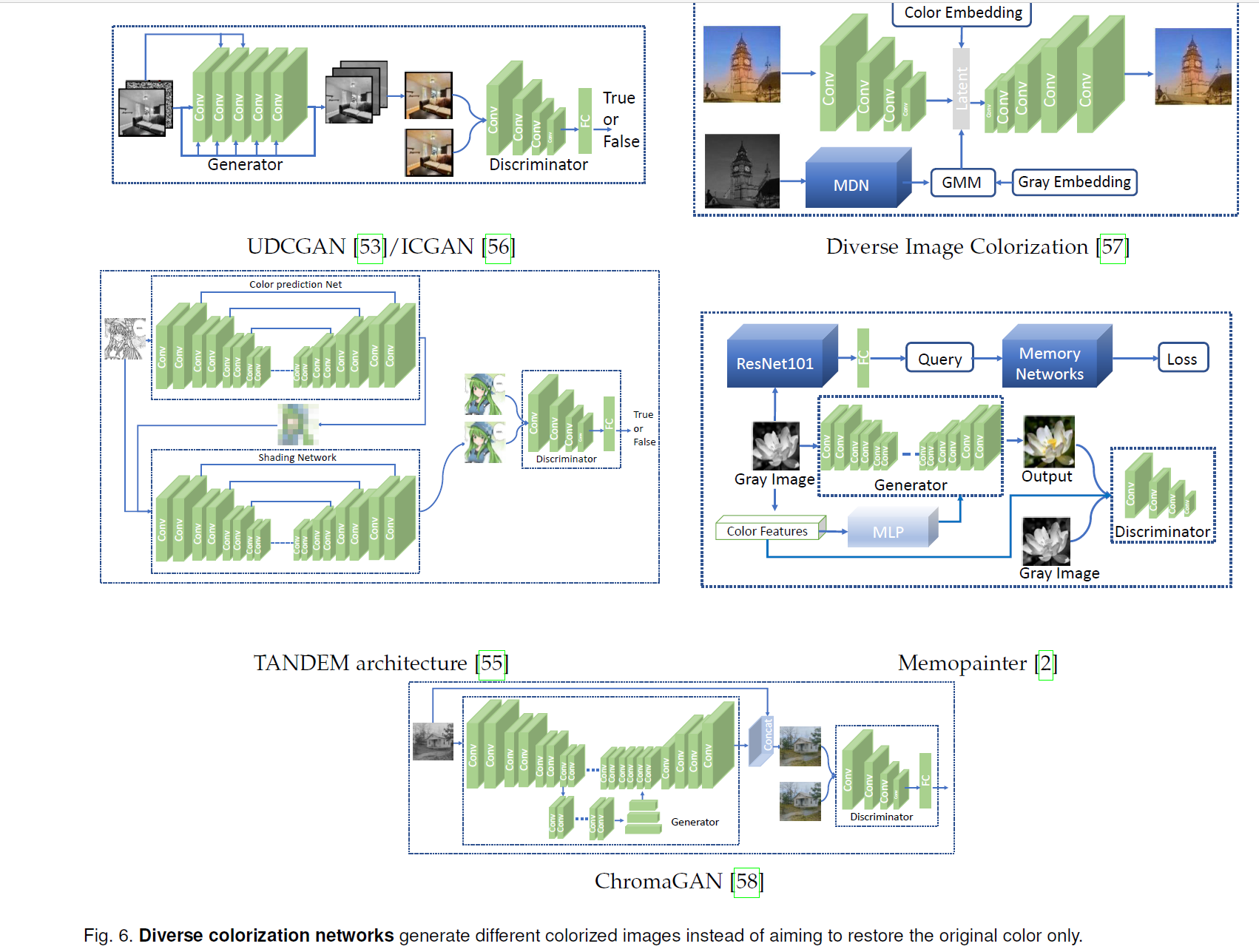
Multi-Path Networks 复杂网络,一般都有额外的指导:如类别信息,语义信息
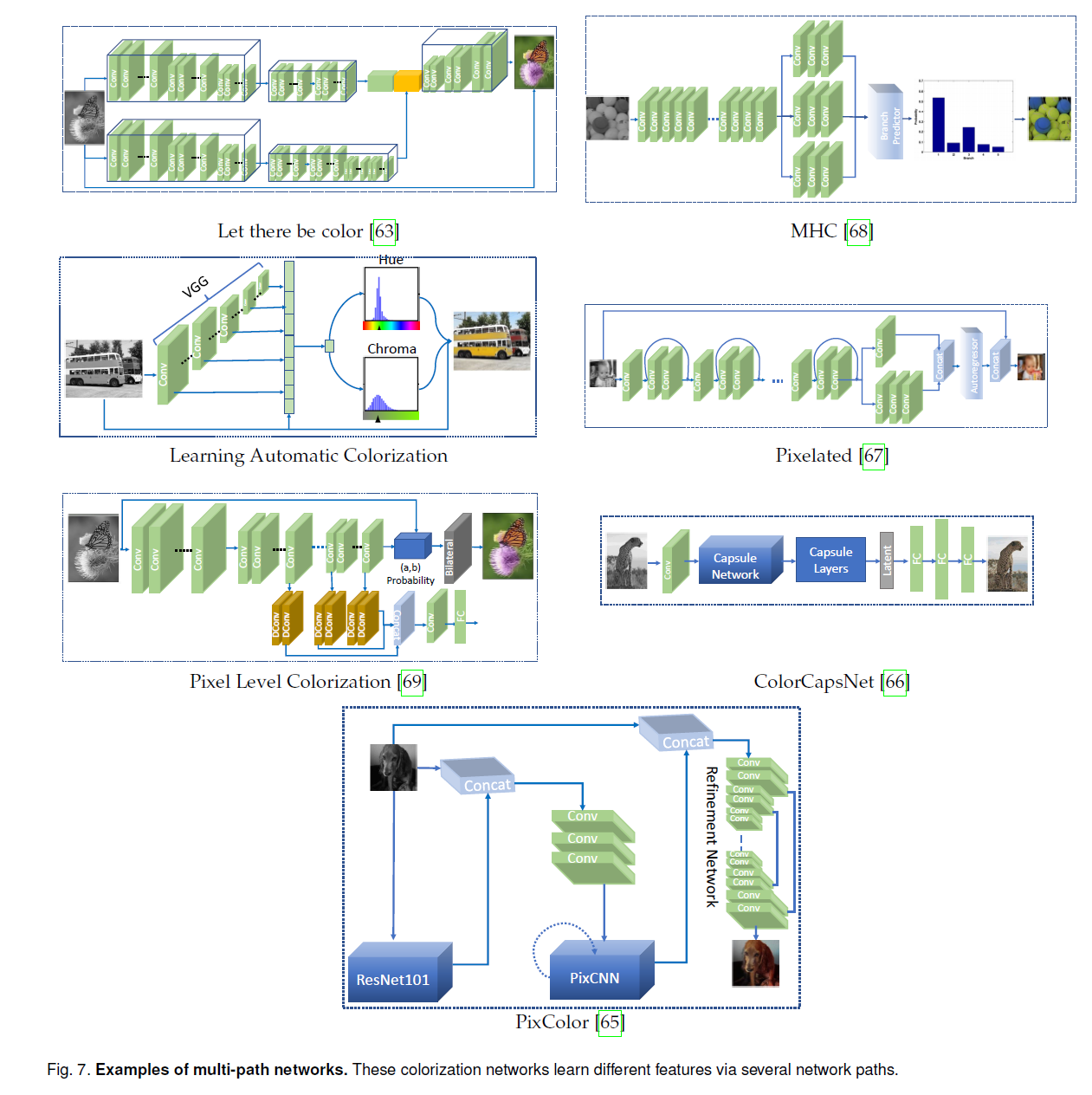
Examplar-Based Colorization Networks 样本指导的网络, 灰度图输入和亮度通道上最相似的彩色图输入作为指导颜色的走向
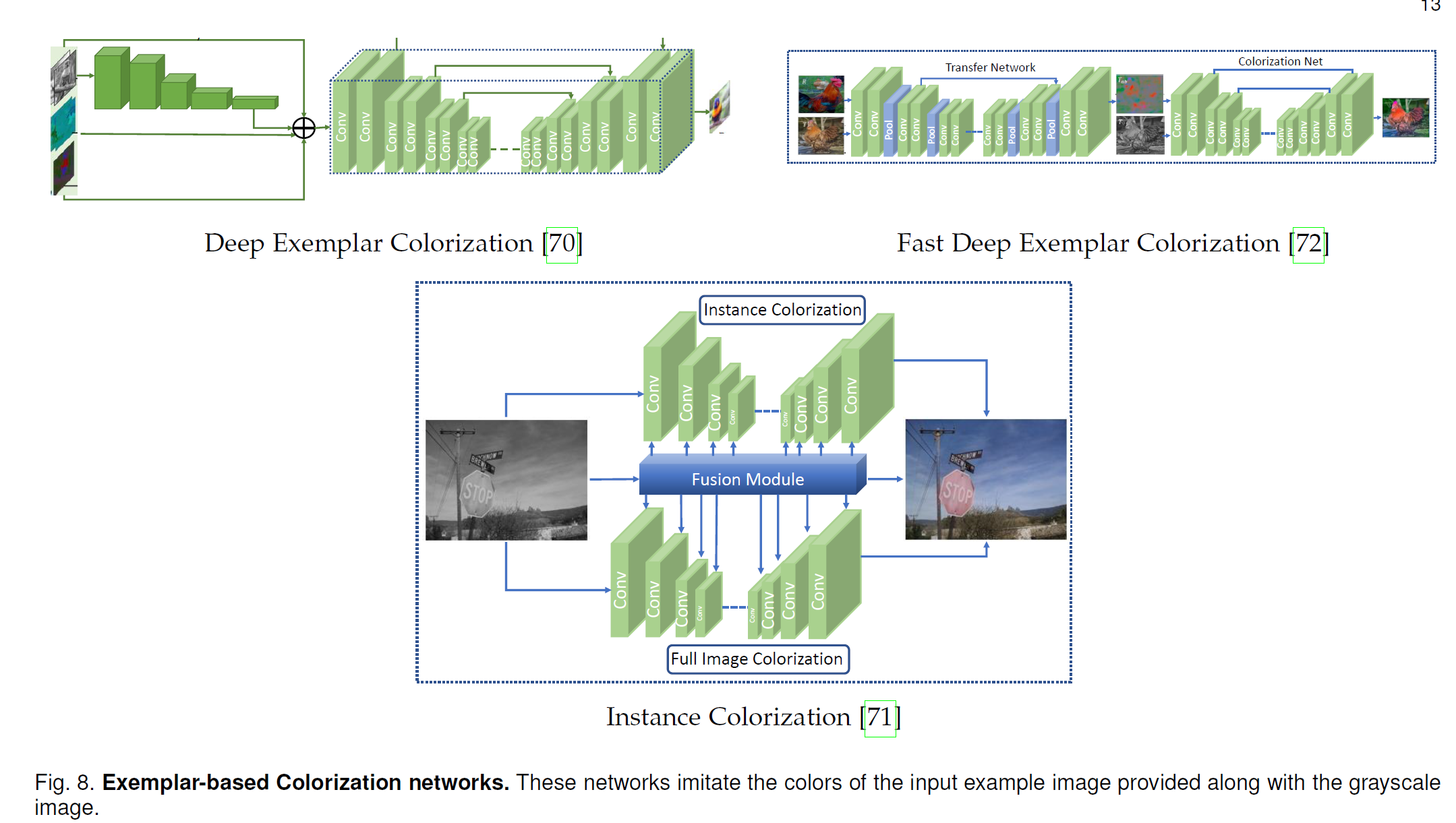
比较典型:Deep Exemplar-based Colorization:有两个步骤组成,一个是相似性网络(样式)一个上色网络, 根据不同的参考给出不同的上色结果
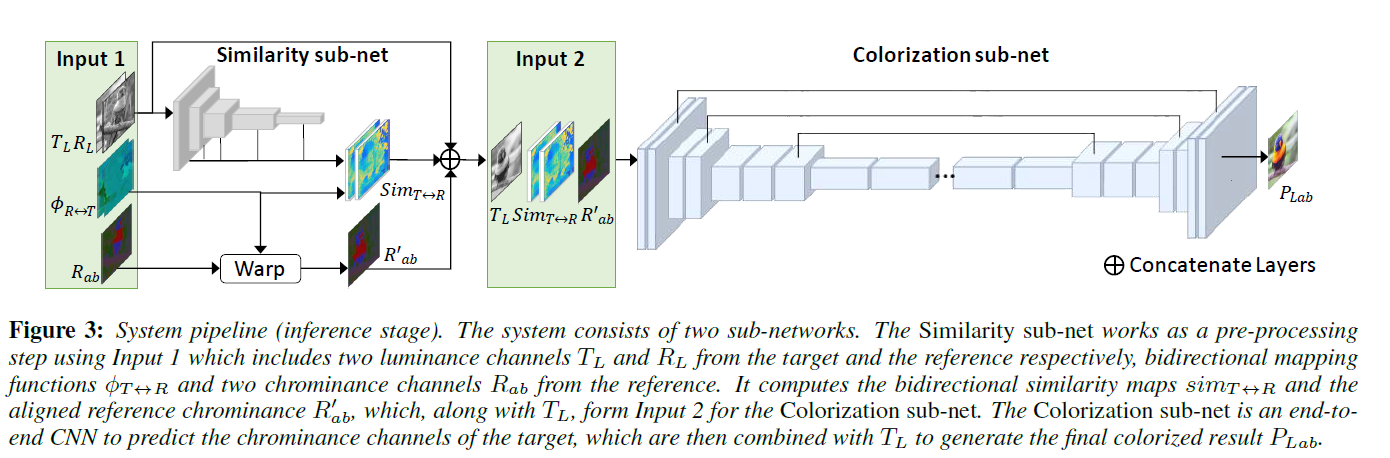
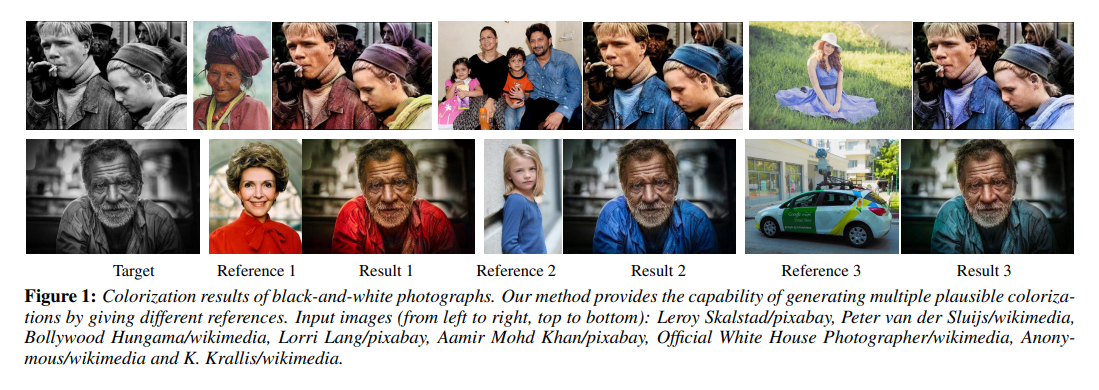
方法的优缺点
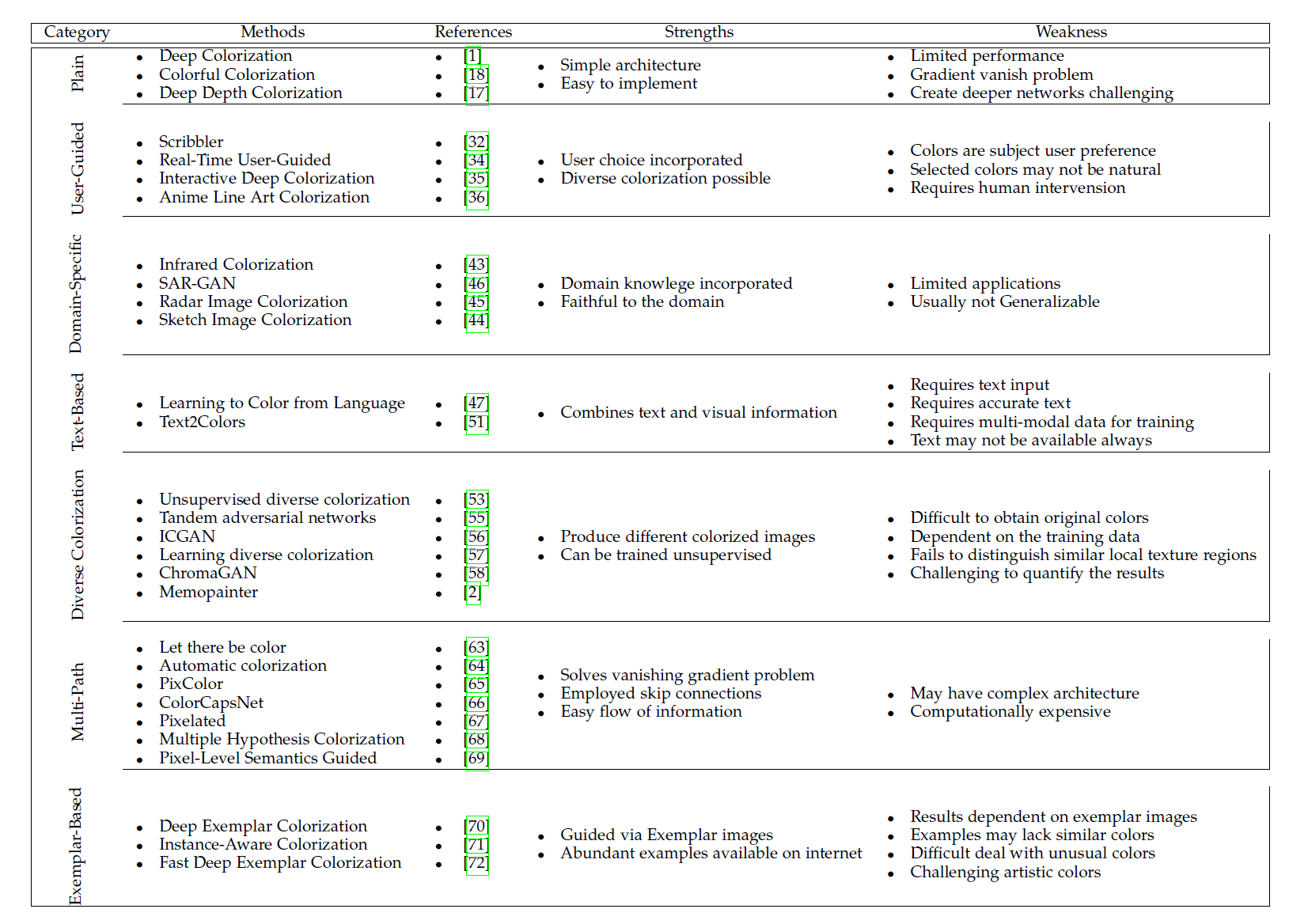
3.视频领域的
Deep Exemplar-based Video Colorization 增加了针对时间上一致性的 loss
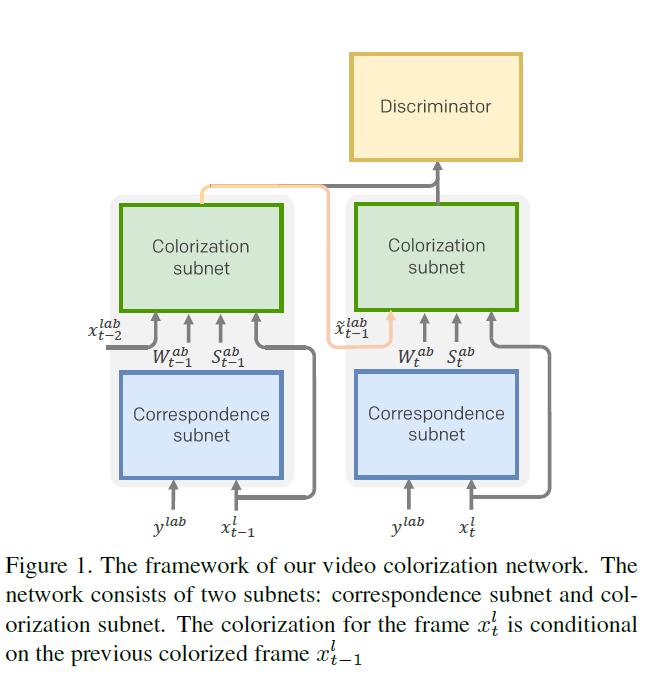
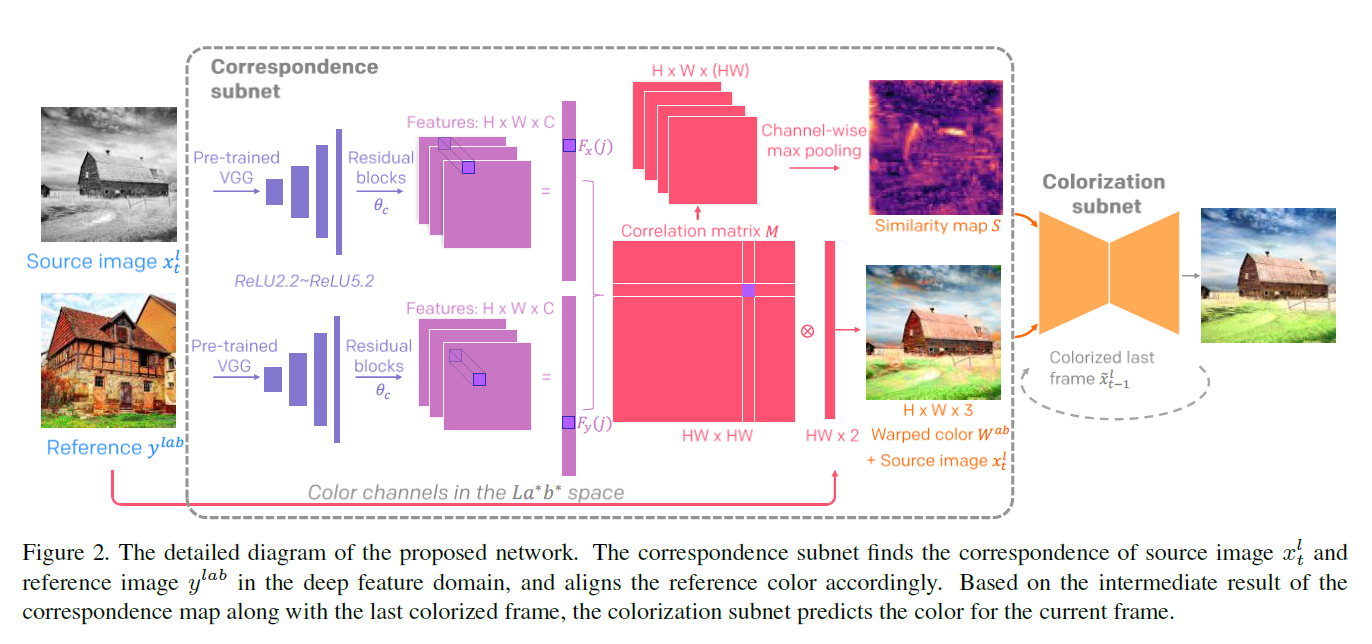
https://github.com/zhangmozhe/Deep-Exemplar-based-Video-Colorization
Automatic Temporally Coherent Video Colorization
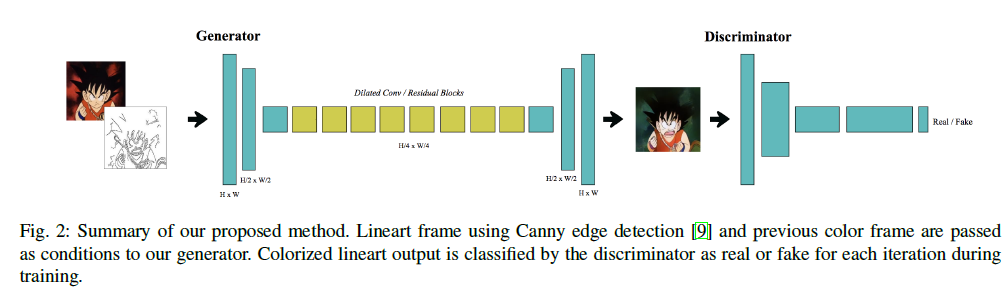
考虑不同镜头的问题
4.数据
COCO-stuff dataset
Places205
VOC
CIFAR
Imagenet ILSVR2012
Palette-and-Text
5.难点
上色有一定先验性,但是又有多样性:一个苹果可以是红色,红色,紫色,绿色,但不可能是蓝色
数据导向,数据的先验性
视频的一致性
评价比较难
上色问题实际上是病态的,其答案也是模棱两可,因为上色问题不止会有一种结果(比如人衣服的颜色)。由于没有一个定量的答案,所以人工干预在上色问题中是必要的。
如何干预?
6.友商结果
颜色不一致
颜色抖动
颜色和先验不一致
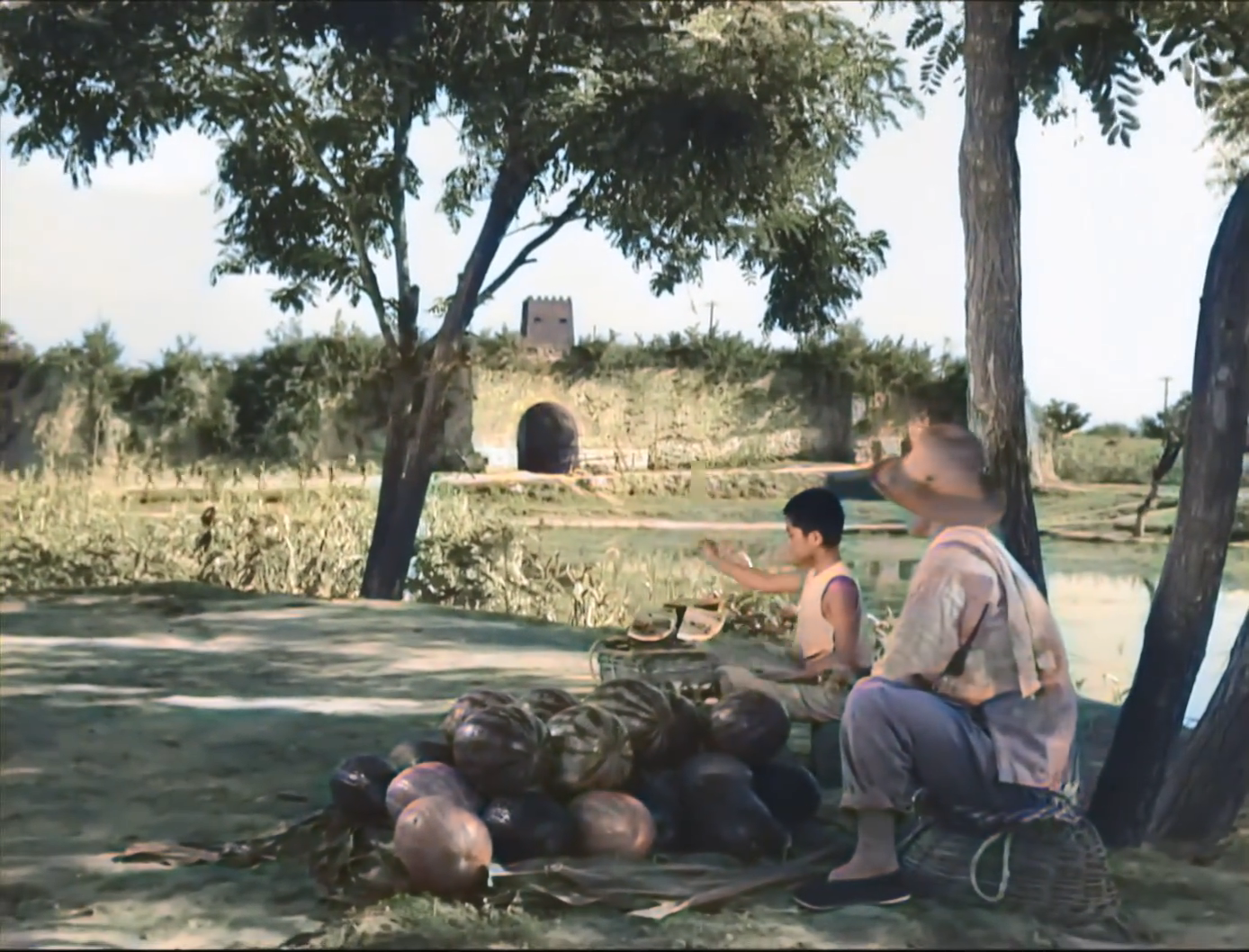
7.应用领域
黑白视频修复,老旧视频,特点课可能时代背景比较强,如革命题材
黑白照片着色
辅助设计如线稿上色
8.参考文档
Image Colorization: A Survey and Dataset
github: https://github.com/saeed-anwar/ColorSurvey
awesome https://github.com/MarkMoHR/Awesome-Image-Colorization
https://github.com/AlexSeongJu-sr/Auto-Colorization
Let there be Color!: Joint End-to-end Learning of Global and Local Image Priors for Automatic Image Colorization with Simultaneous Classification https://github.com/kainoj/colnet
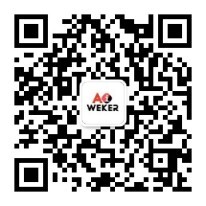
版权声明: 本文为 InfoQ 作者【AIWeker-人工智能微客】的原创文章。
原文链接:【http://xie.infoq.cn/article/e3d68a99aacc27dcd32fa87d8】。文章转载请联系作者。
评论