Google I/O 2021 移动和 Web 应用上的设备端机器学习
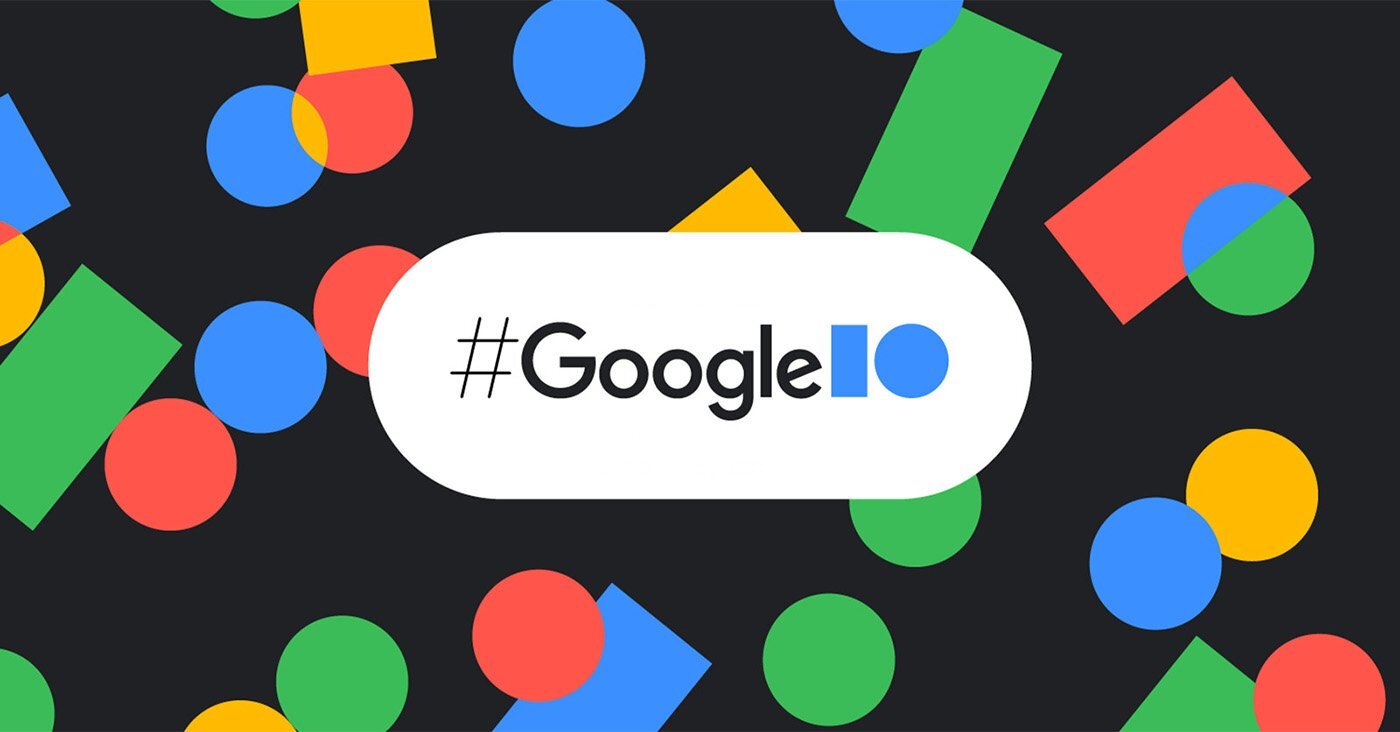
视频地址: https://events.google.com/io/session/187aed3d-2768-4b1a-8047-48c025b7d0bd?lng=zh-CN, 时长 29min34s
本文是 Google I/O 2021,关于《移动和 Web 应用上的设备端机器学习》相关主题的问答视频文字解读,主要是进展性和概念性问题,可参考信息不多
问答内容
Q:What is “on-device machine learning” and why should i care about it
A: 介绍了机器学习在设备端的发展历史,TensorFlow Lite;设备端机器学习在应用场景中的优势:低延时、低功耗、隐私数据...比如本地处理音频帧、视频帧、扫描条形码、离线服务等等
Q: What are some common use cases where ML is being used on-device?
A: 常见应用列举,TikTock、Instgram、Sapchat、文本翻译、语义理解等等
Q:On device ML, we have seen in android devices, but what about web apps? Like on device ML will work as usual in PWAs?
A: 介绍了 TensorFlow.js,提到了 Google I/O 上演示的一个 Demo
Q: I believe that on-device machine learning means having the model on the device or loading it beforehand. So how can we prevent other people that uses our product to extract that model?
(既然模型部署在端上,怎么防止模型被人为提取)
A: 首先,我认为有有一些细节,你不需要打包您的模型作为您的 Android 或 IOS 应用程序的一部分可以准备它,但你不必这样做。你可以做的一件事之一 考虑将您的模型打包为模型的一部分或托管您的模型 和 Firebase 托管,然后使用五个基础 SDK 以便 安装后在设备上下载和管理您的模型 应用程序,这也可以帮助您保持应用程序的大小较小。另一种选择是使用 Android 应用程序包,它可以让您部署 安装后应用程序的工件,因此它再次帮助您保持 你的应用程序。它可以帮助你在安装时保持你的应用程序很小,但是 我认为你问题的第二部分很重要,所以 如何保护我的模型不被盗。我们正在积极思考这个问题。我们是加密和其他机制的一部分 我们正在考虑的部分事情是让它变得更多其他人更难访问您的模型。你能想到的一些事情 的只是你知道也许在设备上运行你的模型的一部分 发送专有部分。也许到服务器端,因为然后 单独的模型可能不是您的应用程序中的秘密武器 它们可能是其他东西等等。
Q: Besides processing power, are there any other disadvantages to using on-device ML? When should you implement on-device ML instead of cloud ML?
A:老问题
Q:ML Kit and TensorFlow Lite appear to have some similarities. Can you provide some guidance around when to use ML Kit vs TensorFlow Lite?
Q: Will concepts like edge computing affect On-device ML? Will it be a positive or negative impact?(边缘计算对设备机器学习的影响)
A:go over to the device ML is just a form of which computing right When we think about HT devices. We think about anything that does not run in the server side. This includes mobile devices. Raspberry Pi is microcontrollers web Linux, devices, desktop, etc etc So so I think they both are the same on device. There is a few terms here that are conflated with each other but I think for the most part. It's computing is running things that are very don't make a server set call and get back response but run it on device Sunday this one
(这里的边缘计算跟设备端机器学习还是两个不同的概念,注意区分)
转到设备 ML 只是其中一种计算方式,我们考虑智能设备、和其他一些任何不会运行在服务器端的东西。这包括移动设备、树莓派、微控制器 web Linux、设备、桌面等 所以我认为它们在设备上这个概念上都是相同的。这里有几个术语是,彼此混为一谈,但我认为在大多数情况下。边缘计算它在计算正在运行的东西本身不需要让服务器设置调用并获取回复响应。但它始终在自己运行。
Q: Adding to the previous question about models getting stolen. how one should handle this in perspective of user's privacy in on device models on the web, since on web it's pretty much accessible easily?
Q: My model is huge and I would like to reduce its size before deploying it on my mobile app. How do I do it?
A:一定要看看 Tensorflow Hub,Tensorflow Hub 是网站上的我们策划的重新选择的模型列表 由 Google 员工和其他开源贡献者贡献,然后你几乎可以在那里找到很多模型,可以满足您的需求,并且已经经过大量优化。另外,我想我们在上面谈到了一些优化技术,作为模型优化工具包的一部分,所以值得一试。
版权声明: 本文为 InfoQ 作者【CatTalk】的原创文章。
原文链接:【http://xie.infoq.cn/article/3f282ea815724743629153abb】。文章转载请联系作者。
评论