Kafka 源码阅读笔记(1)
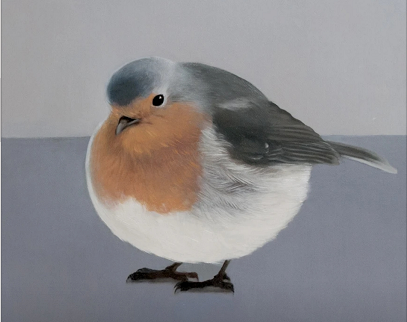
Kafka 源码笔记
1. Server 启动流程
/**
* Start up API for bringing up a single instance of the Kafka server.
* Instantiates the LogManager, the SocketServer and the request handlers - KafkaRequestHandlers
*/
def startup(): Unit = {
try {
info("starting")
if (isShuttingDown.get)
throw new IllegalStateException("Kafka server is still shutting down, cannot re-start!")
if (startupComplete.get)
return
val canStartup = isStartingUp.compareAndSet(false, true)
if (canStartup) {
brokerState.newState(Starting)
/* setup zookeeper */
initZkClient(time)
/* initialize features */
_featureChangeListener = new FinalizedFeatureChangeListener(featureCache, _zkClient)
if (config.isFeatureVersioningSupported) {
_featureChangeListener.initOrThrow(config.zkConnectionTimeoutMs)
}
/* Get or create cluster_id */
_clusterId = getOrGenerateClusterId(zkClient)
info(s"Cluster ID = $clusterId")
/* load metadata */
val (preloadedBrokerMetadataCheckpoint, initialOfflineDirs) = getBrokerMetadataAndOfflineDirs
/* check cluster id */
if (preloadedBrokerMetadataCheckpoint.clusterId.isDefined && preloadedBrokerMetadataCheckpoint.clusterId.get != clusterId)
throw new InconsistentClusterIdException(
s"The Cluster ID ${clusterId} doesn't match stored clusterId ${preloadedBrokerMetadataCheckpoint.clusterId} in meta.properties. " +
s"The broker is trying to join the wrong cluster. Configured zookeeper.connect may be wrong.")
/* generate brokerId */
config.brokerId = getOrGenerateBrokerId(preloadedBrokerMetadataCheckpoint)
logContext = new LogContext(s"[KafkaServer id=${config.brokerId}] ")
this.logIdent = logContext.logPrefix
// initialize dynamic broker configs from ZooKeeper. Any updates made after this will be
// applied after DynamicConfigManager starts.
config.dynamicConfig.initialize(zkClient)
/* start scheduler */
kafkaScheduler = new KafkaScheduler(config.backgroundThreads)
kafkaScheduler.startup()
/* create and configure metrics */
kafkaYammerMetrics = KafkaYammerMetrics.INSTANCE
kafkaYammerMetrics.configure(config.originals)
val jmxReporter = new JmxReporter()
jmxReporter.configure(config.originals)
val reporters = new util.ArrayList[MetricsReporter]
reporters.add(jmxReporter)
val metricConfig = KafkaServer.metricConfig(config)
val metricsContext = createKafkaMetricsContext()
metrics = new Metrics(metricConfig, reporters, time, true, metricsContext)
/* register broker metrics */
_brokerTopicStats = new BrokerTopicStats
quotaManagers = QuotaFactory.instantiate(config, metrics, time, threadNamePrefix.getOrElse(""))
notifyClusterListeners(kafkaMetricsReporters ++ metrics.reporters.asScala)
logDirFailureChannel = new LogDirFailureChannel(config.logDirs.size)
/* start log manager */
logManager = LogManager(config, initialOfflineDirs, zkClient, brokerState, kafkaScheduler, time, brokerTopicStats, logDirFailureChannel)
logManager.startup()
metadataCache = new MetadataCache(config.brokerId)
// Enable delegation token cache for all SCRAM mechanisms to simplify dynamic update.
// This keeps the cache up-to-date if new SCRAM mechanisms are enabled dynamically.
tokenCache = new DelegationTokenCache(ScramMechanism.mechanismNames)
credentialProvider = new CredentialProvider(ScramMechanism.mechanismNames, tokenCache)
// Create and start the socket server acceptor threads so that the bound port is known.
// Delay starting processors until the end of the initialization sequence to ensure
// that credentials have been loaded before processing authentications.
socketServer = new SocketServer(config, metrics, time, credentialProvider)
socketServer.startup(startProcessingRequests = false)
/* start replica manager */
brokerToControllerChannelManager = new BrokerToControllerChannelManagerImpl(metadataCache, time, metrics, config, threadNamePrefix)
replicaManager = createReplicaManager(isShuttingDown)
replicaManager.startup()
brokerToControllerChannelManager.start()
val brokerInfo = createBrokerInfo
val brokerEpoch = zkClient.registerBroker(brokerInfo)
// Now that the broker is successfully registered, checkpoint its metadata
checkpointBrokerMetadata(BrokerMetadata(config.brokerId, Some(clusterId)))
/* start token manager */
tokenManager = new DelegationTokenManager(config, tokenCache, time , zkClient)
tokenManager.startup()
/* start kafka controller */
kafkaController = new KafkaController(config, zkClient, time, metrics, brokerInfo, brokerEpoch, tokenManager, brokerFeatures, featureCache, threadNamePrefix)
kafkaController.startup()
adminManager = new AdminManager(config, metrics, metadataCache, zkClient)
/* start group coordinator */
// Hardcode Time.SYSTEM for now as some Streams tests fail otherwise, it would be good to fix the underlying issue
groupCoordinator = GroupCoordinator(config, zkClient, replicaManager, Time.SYSTEM, metrics)
groupCoordinator.startup()
/* start transaction coordinator, with a separate background thread scheduler for transaction expiration and log loading */
// Hardcode Time.SYSTEM for now as some Streams tests fail otherwise, it would be good to fix the underlying issue
transactionCoordinator = TransactionCoordinator(config, replicaManager, new KafkaScheduler(threads = 1, threadNamePrefix = "transaction-log-manager-"), zkClient, metrics, metadataCache, Time.SYSTEM)
transactionCoordinator.startup()
/* Get the authorizer and initialize it if one is specified.*/
authorizer = config.authorizer
authorizer.foreach(_.configure(config.originals))
val authorizerFutures: Map[Endpoint, CompletableFuture[Void]] = authorizer match {
case Some(authZ) =>
authZ.start(brokerInfo.broker.toServerInfo(clusterId, config)).asScala.map { case (ep, cs) =>
ep -> cs.toCompletableFuture
}
case None =>
brokerInfo.broker.endPoints.map { ep =>
ep.toJava -> CompletableFuture.completedFuture[Void](null)
}.toMap
}
val fetchManager = new FetchManager(Time.SYSTEM,
new FetchSessionCache(config.maxIncrementalFetchSessionCacheSlots,
KafkaServer.MIN_INCREMENTAL_FETCH_SESSION_EVICTION_MS))
/* start processing requests */
dataPlaneRequestProcessor = new KafkaApis(socketServer.dataPlaneRequestChannel, replicaManager, adminManager, groupCoordinator, transactionCoordinator,
kafkaController, zkClient, config.brokerId, config, metadataCache, metrics, authorizer, quotaManagers,
fetchManager, brokerTopicStats, clusterId, time, tokenManager, brokerFeatures, featureCache)
dataPlaneRequestHandlerPool = new KafkaRequestHandlerPool(config.brokerId, socketServer.dataPlaneRequestChannel, dataPlaneRequestProcessor, time,
config.numIoThreads, s"${SocketServer.DataPlaneMetricPrefix}RequestHandlerAvgIdlePercent", SocketServer.DataPlaneThreadPrefix)
socketServer.controlPlaneRequestChannelOpt.foreach { controlPlaneRequestChannel =>
controlPlaneRequestProcessor = new KafkaApis(controlPlaneRequestChannel, replicaManager, adminManager, groupCoordinator, transactionCoordinator,
kafkaController, zkClient, config.brokerId, config, metadataCache, metrics, authorizer, quotaManagers,
fetchManager, brokerTopicStats, clusterId, time, tokenManager, brokerFeatures, featureCache)
controlPlaneRequestHandlerPool = new KafkaRequestHandlerPool(config.brokerId, socketServer.controlPlaneRequestChannelOpt.get, controlPlaneRequestProcessor, time,
1, s"${SocketServer.ControlPlaneMetricPrefix}RequestHandlerAvgIdlePercent", SocketServer.ControlPlaneThreadPrefix)
}
Mx4jLoader.maybeLoad()
/* Add all reconfigurables for config change notification before starting config handlers */
config.dynamicConfig.addReconfigurables(this)
/* start dynamic config manager */
dynamicConfigHandlers = Map[String, ConfigHandler](ConfigType.Topic -> new TopicConfigHandler(logManager, config, quotaManagers, kafkaController),
ConfigType.Client -> new ClientIdConfigHandler(quotaManagers),
ConfigType.User -> new UserConfigHandler(quotaManagers, credentialProvider),
ConfigType.Broker -> new BrokerConfigHandler(config, quotaManagers))
// Create the config manager. start listening to notifications
dynamicConfigManager = new DynamicConfigManager(zkClient, dynamicConfigHandlers)
dynamicConfigManager.startup()
socketServer.startProcessingRequests(authorizerFutures)
brokerState.newState(RunningAsBroker)
shutdownLatch = new CountDownLatch(1)
startupComplete.set(true)
isStartingUp.set(false)
AppInfoParser.registerAppInfo(metricsPrefix, config.brokerId.toString, metrics, time.milliseconds())
info("started")
}
}
catch {
case e: Throwable =>
fatal("Fatal error during KafkaServer startup. Prepare to shutdown", e)
isStartingUp.set(false)
shutdown()
throw e
}
}
KafkaController#startup 中为每一个 server 都会启动一个 eventManager
/**
* Invoked when the controller module of a Kafka server is started up. This does not assume that the current broker
* is the controller. It merely registers the session expiration listener and starts the controller leader
* elector
*/
def startup() = {
zkClient.registerStateChangeHandler(new StateChangeHandler {
override val name: String = StateChangeHandlers.ControllerHandler
override def afterInitializingSession(): Unit = {
eventManager.put(RegisterBrokerAndReelect)
}
override def beforeInitializingSession(): Unit = {
val queuedEvent = eventManager.clearAndPut(Expire)
// Block initialization of the new session until the expiration event is being handled,
// which ensures that all pending events have been processed before creating the new session
queuedEvent.awaitProcessing()
}
})
eventManager.put(Startup)
eventManager.start()
}
Startup 类型的 ControllerEvent 被放入到 eventmanager 中,被 KafkaController#process 方法调用
override def process(event: ControllerEvent): Unit = {
try {
event match {
case event: ……
case Startup =>
processStartup()
}
} catch {
case e: ControllerMovedException =>
info(s"Controller moved to another broker when processing $event.", e)
maybeResign()
case e: Throwable =>
error(s"Error processing event $event", e)
} finally {
updateMetrics()
}
}
private def processStartup(): Unit = {
zkClient.registerZNodeChangeHandlerAndCheckExistence(controllerChangeHandler)
elect()
}
elect 就是尝试竞选 controller,如果我们当前节点真的被选为 controller(onControllerFailover()--故障转移)
/*
1.把controller的epoch号码+1
2.启动controller的channel manager用于接收请求
3.启动replica的状态机,监测replica是OnlineReplica还是OfflineReplica的状态。这里的offline是指该replica的broker已经挂掉。
4.启动partition的状态机,监测partition是OnlinePartition还是OfflinePartition。这里的offline是指该partion的leader已经挂掉。
5.启动自动的leader分配rebalance(如果启动设置)
*/
replicaStateMachine.startup()
partitionStateMachine.startup()
2. 日志操作
kafka 后台抽象的 Log 由若干个 LogSegment 组成。
每一个 LogSegments 都有一个 baseOffset,是该 logsegment 第一条消息的偏移量。Server 根据时间或者大小限制来创建新的 LogSegment。
Log 是一个 partition 的一个 replica 的存储。
各个 server 的日志存储不一定相同,即使是相同的 topic 里面相同 partion 的副本,存储的起始 offset 也不相同。
后台维护线程
日志操作主要包含一下几个后台线程:
LogManager#startup
def startup(): Unit = {
/* Schedule the cleanup task to delete old logs */
if (scheduler != null) {
info("Starting log cleanup with a period of %d ms.".format(retentionCheckMs))
// 遍历所有Log。负责清理未压缩的日志,清除条件
// 1.日志超过保留时间 2.日志大小超过保留大小
scheduler.schedule("kafka-log-retention",
cleanupLogs _,
delay = InitialTaskDelayMs,
period = retentionCheckMs,
TimeUnit.MILLISECONDS)
info("Starting log flusher with a default period of %d ms.".format(flushCheckMs))
// 将超过写回限制时间且存在更新的Log写回磁盘。
// 调用JAVA NIO中的FileChannel中的force,该方法将负责将channel中的所有未未写入磁盘的内容写入磁盘。
scheduler.schedule("kafka-log-flusher",
flushDirtyLogs _,
delay = InitialTaskDelayMs,
period = flushCheckMs,
TimeUnit.MILLISECONDS)
// 向路径中写入当前的恢复点,避免在重启时需要重新恢复全部数据
scheduler.schedule("kafka-recovery-point-checkpoint",
checkpointLogRecoveryOffsets _,
delay = InitialTaskDelayMs,
period = flushRecoveryOffsetCheckpointMs,
TimeUnit.MILLISECONDS)
// 向日志目录写入当前存储的日志中的start offset。避免读到已经被删除的日志
scheduler.schedule("kafka-log-start-offset-checkpoint",
checkpointLogStartOffsets _,
delay = InitialTaskDelayMs,
period = flushStartOffsetCheckpointMs,
TimeUnit.MILLISECONDS)
// 清理已经被标记为删除的日志
scheduler.schedule("kafka-delete-logs", // will be rescheduled after each delete logs with a dynamic period
deleteLogs _,
delay = InitialTaskDelayMs,
unit = TimeUnit.MILLISECONDS)
}
if (cleanerConfig.enableCleaner)
cleaner.startup()
}
Log 的 flush 会具体到某个 segment 的 flush
/**
* Flush log segments for all offsets up to offset-1
*
* @param offset The offset to flush up to (non-inclusive); the new recovery point
*/
def flush(offset: Long): Unit = {
maybeHandleIOException(s"Error while flushing log for $topicPartition in dir ${dir.getParent} with offset $offset") {
if (offset <= this.recoveryPoint)
return
debug(s"Flushing log up to offset $offset, last flushed: $lastFlushTime, current time: ${time.milliseconds()}, " +
s"unflushed: $unflushedMessages")
for (segment <- logSegments(this.recoveryPoint, offset))
segment.flush()
lock synchronized {
checkIfMemoryMappedBufferClosed()
if (offset > this.recoveryPoint) {
this.recoveryPoint = offset
lastFlushedTime.set(time.milliseconds)
}
}
}
}
最终的操作会终止在 FileRecord.java 中实现,封装了 JAVA NIO 中 FILE CHANNEL 的常见操作
日志追加流程
最终 channel.write 是将 MemoryRecords 中的 bytebuffer 写入等到了磁盘
MemoryRecords 在 kafka 中的形态如下:
public class MemoryRecords extends AbstractRecords {
// 封装了NIO中的ByteBuffer
private final ByteBuffer buffer;
FileRecord#open 在打开文件的时候使用 java.io 的 File 类初始化该实例的 channel
public class FileRecords extends AbstractRecords implements Closeable {
// 访问该文件的channel
private final FileChannel channel;
private volatile File file;
//在打开文件的时候使用java.io的File类初始化该实例的channel
public static FileRecords open(File file,
boolean mutable,
boolean fileAlreadyExists,
int initFileSize,
boolean preallocate) throws IOException {
FileChannel channel = openChannel(file, mutable, fileAlreadyExists, initFileSize, preallocate);
int end = (!fileAlreadyExists && preallocate) ? 0 : Integer.MAX_VALUE;
return new FileRecords(file, channel, 0, end, false);
}
//把内存中的记录追加到文件中
public int append(MemoryRecords records) throws IOException {
if (records.sizeInBytes() > Integer.MAX_VALUE - size.get())
throw new IllegalArgumentException("Append of size " + records.sizeInBytes() +
" bytes is too large for segment with current file position at " + size.get());
//底层实现就是channel.write(buffer)
int written = records.writeFullyTo(channel);
size.getAndAdd(written);
return written;
}
##MemoryRecords
public int writeFullyTo(GatheringByteChannel channel) throws IOException {
buffer.mark();
int written = 0;
while (written < sizeInBytes())
written += channel.write(buffer);
buffer.reset();
return written;
}
日志读取流程
FileRecords 类提供了两种方式来读取日志文件:
采用 NIO 中的 channel.read 将内容读到 NIO 中的 ByteBuffer 里
采用 NIO 中 fileChannel.transferTo 将内容直接零拷贝到 socketchannel 中。注意,这一步,broker 端既不对数据进行解压缩,而是将压缩数据直接发给客户端,让客户端进行解压缩。
第一种方式:
//##FileRecords.java
public ByteBuffer readInto(ByteBuffer buffer, int position) throws IOException {
Utils.readFully(channel, buffer, position + this.start);
//buffer从写入模式切换到了读出模式,返回
buffer.flip();
return buffer;
}
//##Utils.java
public static void readFully(FileChannel channel, ByteBuffer destinationBuffer, long position) throws IOException {
if (position < 0) {
throw new IllegalArgumentException("The file channel position cannot be negative, but it is " + position);
}
long currentPosition = position;
int bytesRead;
do {
bytesRead = channel.read(destinationBuffer, currentPosition);
currentPosition += bytesRead;
} while (bytesRead != -1 && destinationBuffer.hasRemaining());
}
第二种方式:在操作系统支持的情况下,该数据并不需要将源数据从内核态拷贝到用户态,再从用户态拷贝到内核态。
// ##FileRecords.java
@Override
public long writeTo(GatheringByteChannel destChannel, long offset, int length) throws IOException {
final long bytesTransferred;
if (destChannel instanceof TransportLayer) {
//写入传输层的socket
TransportLayer tl = (TransportLayer) destChannel;
bytesTransferred = tl.transferFrom(channel, position, count);
} else {
bytesTransferred = channel.transferTo(position, count, destChannel);
}
return bytesTransferred;
}
// ##PlaintextTransportLayer.java
public class PlaintextTransportLayer implements TransportLayer {
//实例保存的具体socket
private final SocketChannel socketChannel;
@Override
public long transferFrom(FileChannel fileChannel, long position, long count) throws IOException {
//NIO包方法,从filechannel零拷贝到socketchannel。
return fileChannel.transferTo(position, count, socketChannel);
}
3. 网络链接
kafka 使用自己写的 socket server,使用标准的 reactor 多线程模式。
启动流程如下:KafkaServer#startup 下对 socketServer#startup
/**
* Starts the socket server and creates all the Acceptors and the Processors. The Acceptors
* start listening at this stage so that the bound port is known when this method completes
* even when ephemeral ports are used. Acceptors and Processors are started if `startProcessingRequests`
* is true. If not, acceptors and processors are only started when [[kafka.network.SocketServer#startProcessingRequests()]]
* is invoked. Delayed starting of acceptors and processors is used to delay processing client
* connections until server is fully initialized, e.g. to ensure that all credentials have been
* loaded before authentications are performed. Incoming connections on this server are processed
* when processors start up and invoke [[org.apache.kafka.common.network.Selector#poll]].
*
* @param startProcessingRequests Flag indicating whether `Processor`s must be started.
*/
def startup(startProcessingRequests: Boolean = true): Unit = {
this.synchronized {
connectionQuotas = new ConnectionQuotas(config, time, metrics)
// qi
createControlPlaneAcceptorAndProcessor(config.controlPlaneListener)
createDataPlaneAcceptorsAndProcessors(config.numNetworkThreads, config.dataPlaneListeners)
if (startProcessingRequests) {
this.startProcessingRequests()
}
}
}
// ## 创建控制层Acceptor和Processor
private def createControlPlaneAcceptorAndProcessor(endpointOpt: Option[EndPoint]): Unit = {
endpointOpt.foreach { endpoint =>
connectionQuotas.addListener(config, endpoint.listenerName)
val controlPlaneAcceptor = createAcceptor(endpoint, ControlPlaneMetricPrefix)
val controlPlaneProcessor = newProcessor(nextProcessorId, controlPlaneRequestChannelOpt.get, connectionQuotas, endpoint.listenerName, endpoint.securityProtocol, memoryPool)
controlPlaneAcceptorOpt = Some(controlPlaneAcceptor)
controlPlaneProcessorOpt = Some(controlPlaneProcessor)
val listenerProcessors = new ArrayBuffer[Processor]()
listenerProcessors += controlPlaneProcessor
controlPlaneRequestChannelOpt.foreach(_.addProcessor(controlPlaneProcessor))
nextProcessorId += 1
controlPlaneAcceptor.addProcessors(listenerProcessors, ControlPlaneThreadPrefix)
info(s"Created control-plane acceptor and processor for endpoint : ${endpoint.listenerName}")
}
}
// ## 数据数据层Acceptor和Processor
private def createDataPlaneAcceptorsAndProcessors(dataProcessorsPerListener: Int,
endpoints: Seq[EndPoint]): Unit = {
endpoints.foreach { endpoint =>
connectionQuotas.addListener(config, endpoint.listenerName)
val dataPlaneAcceptor = createAcceptor(endpoint, DataPlaneMetricPrefix)
addDataPlaneProcessors(dataPlaneAcceptor, endpoint, dataProcessorsPerListener)
dataPlaneAcceptors.put(endpoint, dataPlaneAcceptor)
info(s"Created data-plane acceptor and processors for endpoint : ${endpoint.listenerName}")
}
}
// ## 创建acceptor
private def createAcceptor(endPoint: EndPoint, metricPrefix: String) : Acceptor = {
val sendBufferSize = config.socketSendBufferBytes
val recvBufferSize = config.socketReceiveBufferBytes
val brokerId = config.brokerId
new Acceptor(endPoint, sendBufferSize, recvBufferSize, brokerId, connectionQuotas, metricPrefix)
}
// ## 创建并添加数据层Processers
private def addDataPlaneProcessors(acceptor: Acceptor, endpoint: EndPoint, newProcessorsPerListener: Int): Unit = {
val listenerName = endpoint.listenerName
val securityProtocol = endpoint.securityProtocol
val listenerProcessors = new ArrayBuffer[Processor]()
for (_ <- 0 until newProcessorsPerListener) {
val processor = newProcessor(nextProcessorId, dataPlaneRequestChannel, connectionQuotas, listenerName, securityProtocol, memoryPool)
listenerProcessors += processor
dataPlaneRequestChannel.addProcessor(processor)
nextProcessorId += 1
}
listenerProcessors.foreach(p => dataPlaneProcessors.put(p.id, p))
acceptor.addProcessors(listenerProcessors, DataPlaneThreadPrefix)
}
Aceptor 线程核心是一段循环代码,负责处理新加入的连接
while (isRunning) {
try {
// 阻塞查询需要处理的连接事件
val ready = nioSelector.select(500)
if (ready > 0) {
val keys = nioSelector.selectedKeys()
val iter = keys.iterator()
while (iter.hasNext && isRunning) {
try {
val key = iter.next
iter.remove()
if (key.isAcceptable) {
// 该方法调用processor.accept来接收新connection
accept(key).foreach { socketChannel =>
// Assign the channel to the next processor (using round-robin) to which the
// channel can be added without blocking. If newConnections queue is full on
// all processors, block until the last one is able to accept a connection.
var retriesLeft = synchronized(processors.length)
var processor: Processor = null
do {
retriesLeft -= 1
processor = synchronized {
// adjust the index (if necessary) and retrieve the processor atomically for
// correct behaviour in case the number of processors is reduced dynamically
currentProcessorIndex = currentProcessorIndex % processors.length
processors(currentProcessorIndex)
}
currentProcessorIndex += 1
} while (!assignNewConnection(socketChannel, processor, retriesLeft == 0))
}
} else
throw new IllegalStateException("Unrecognized key state for acceptor thread.")
} catch {
case e: Throwable => error("Error while accepting connection", e)
}
}
}
}
Processor 相当于 netty 中 workergroup 中的线程,链接建立以后,channel 被交给 Processor,负责相应之后需要处理的读写。Processor 主流程如下:
while (isRunning) {
try {
// setup any new connections that have been queued up
configureNewConnections()
// register any new responses for writing
processNewResponses()
poll()
processCompletedReceives()
processCompletedSends()
processDisconnected()
closeExcessConnections()
} catch { ……
处理接收的逻辑如下:
while (isRunning) {
try {
// setup any new connections that have been queued up
configureNewConnections()
// register any new responses for writing
processNewResponses()
poll()
processCompletedReceives() // 处理接收
processCompletedSends()
processDisconnected()
closeExcessConnections()
} catch {……
// ##processCompletedReceives()处理接收内部实际是将消息发给channel,requestChannel.sendRequest(req)
/** Send a request to be handled, potentially blocking until there is room in the queue for the request */
def sendRequest(request: RequestChannel.Request): Unit = {
requestQueue.put(request)
}
// ## KafkaRequestHandler线程主要方法#run中主要是从requestQueue中取请求
requestChannel.receiveRequest(300)
/** Get the next request or block until specified time has elapsed */
def receiveRequest(timeout: Long): RequestChannel.BaseRequest =
requestQueue.poll(timeout, TimeUnit.MILLISECONDS)
Clients 端底层使用 java 的 java.nio.channels 的 SocketChannel。分别封装了 PlaintextTransportLayer 和 SslTransportLayer.同样也使用了 nio 的 selector。
第一步:要找到所有准备就绪的 SelectionKey
第二步:对所有准备就绪的 key 按着情况处理
第一步:Selector#poll,中需要找到 key
Set<SelectionKey> readyKeys = this.nioSelector.selectedKeys();
第二步:处理 key
**
* handle any ready I/O on a set of selection keys
* @param selectionKeys set of keys to handle
* @param isImmediatelyConnected true if running over a set of keys for just-connected sockets
* @param currentTimeNanos time at which set of keys was determined
*/
void pollSelectionKeys(Set<SelectionKey> selectionKeys,
boolean isImmediatelyConnected,
long currentTimeNanos) {
for (SelectionKey key : determineHandlingOrder(selectionKeys)) {
KafkaChannel channel = channel(key);
try {
/* complete any connections that have finished their handshake (either normally or immediately) */
if (isImmediatelyConnected || key.isConnectable()) {
// 处理TCP链接完成事件
if (channel.finishConnect()) {
……
} else {
continue;
}
}
/* if channel is not ready finish prepare */
if (channel.isConnected() && !channel.ready()) {
channel.prepare();
if (channel.ready()) {
long readyTimeMs = time.milliseconds();
boolean isReauthentication = channel.successfulAuthentications() > 1;
if (isReauthentication) {
sensors.successfulReauthentication.record(1.0, readyTimeMs);
if (channel.reauthenticationLatencyMs() == null)
log.warn(
"Should never happen: re-authentication latency for a re-authenticated channel was null; continuing...");
else
sensors.reauthenticationLatency
.record(channel.reauthenticationLatencyMs().doubleValue(), readyTimeMs);
} else {
sensors.successfulAuthentication.record(1.0, readyTimeMs);
if (!channel.connectedClientSupportsReauthentication())
sensors.successfulAuthenticationNoReauth.record(1.0, readyTimeMs);
}
log.debug("Successfully {}authenticated with {}", isReauthentication ?
"re-" : "", channel.socketDescription());
}
}
if (channel.ready() && channel.state() == ChannelState.NOT_CONNECTED)
channel.state(ChannelState.READY);
Optional<NetworkReceive> responseReceivedDuringReauthentication = channel.pollResponseReceivedDuringReauthentication();
responseReceivedDuringReauthentication.ifPresent(receive -> {
long currentTimeMs = time.milliseconds();
addToCompletedReceives(channel, receive, currentTimeMs);
});
//if channel is ready and has bytes to read from socket or buffer, and has no
//previous completed receive then read from it
if (channel.ready() && (key.isReadable() || channel.hasBytesBuffered()) && !hasCompletedReceive(channel)
&& !explicitlyMutedChannels.contains(channel)) {
attemptRead(channel);
}
if (channel.hasBytesBuffered()) {
//this channel has bytes enqueued in intermediary buffers that we could not read
//(possibly because no memory). it may be the case that the underlying socket will
//not come up in the next poll() and so we need to remember this channel for the
//next poll call otherwise data may be stuck in said buffers forever. If we attempt
//to process buffered data and no progress is made, the channel buffered status is
//cleared to avoid the overhead of checking every time.
keysWithBufferedRead.add(key);
}
/* if channel is ready write to any sockets that have space in their buffer and for which we have data */
long nowNanos = channelStartTimeNanos != 0 ? channelStartTimeNanos : currentTimeNanos;
try {
// 处理发送事件
attemptWrite(key, channel, nowNanos);
} catch (Exception e) {
sendFailed = true;
throw e;
}
/* cancel any defunct sockets */
if (!key.isValid())
close(channel, CloseMode.GRACEFUL);
} catch (Exception e) {……
}
}
4. Producer
KafkaProducer 把消息放到本地消息队列,然后后台有一个发送线程 Sender 不停的循环,把消息发给 Kafka 集群
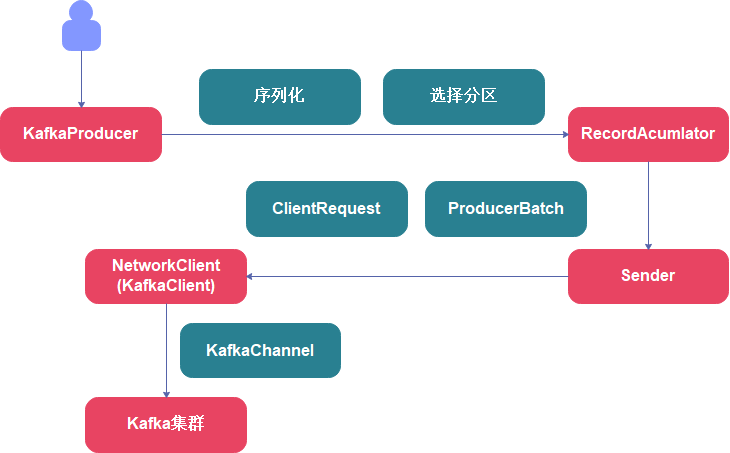
/**
* Implementation of asynchronously send a record to a topic.
*/
private Future<RecordMetadata> doSend(ProducerRecord<K, V> record, Callback callback) {
TopicPartition tp = null;
try {
throwIfProducerClosed();
// first make sure the metadata for the topic is available
long nowMs = time.milliseconds();
ClusterAndWaitTime clusterAndWaitTime;
try {
clusterAndWaitTime = waitOnMetadata(record.topic(), record.partition(), nowMs, maxBlockTimeMs);
} catch (KafkaException e) {
if (metadata.isClosed())
throw new KafkaException("Producer closed while send in progress", e);
throw e;
}
nowMs += clusterAndWaitTime.waitedOnMetadataMs;
long remainingWaitMs = Math.max(0, maxBlockTimeMs - clusterAndWaitTime.waitedOnMetadataMs);
Cluster cluster = clusterAndWaitTime.cluster;
byte[] serializedKey;
try {
// 序列化key
serializedKey = keySerializer.serialize(record.topic(), record.headers(), record.key());
} catch (ClassCastException cce) {
throw new SerializationException("Can't convert key of class " + record.key().getClass().getName() +
" to class " + producerConfig.getClass(ProducerConfig.KEY_SERIALIZER_CLASS_CONFIG).getName() +
" specified in key.serializer", cce);
}
byte[] serializedValue;
try {
// 序列化value
serializedValue = valueSerializer.serialize(record.topic(), record.headers(), record.value());
} catch (ClassCastException cce) {
throw new SerializationException("Can't convert value of class " + record.value().getClass().getName() +
" to class " + producerConfig.getClass(ProducerConfig.VALUE_SERIALIZER_CLASS_CONFIG).getName() +
" specified in value.serializer", cce);
}
// 构造分区
int partition = partition(record, serializedKey, serializedValue, cluster);
tp = new TopicPartition(record.topic(), partition);
setReadOnly(record.headers());
Header[] headers = record.headers().toArray();
int serializedSize = AbstractRecords.estimateSizeInBytesUpperBound(apiVersions.maxUsableProduceMagic(),
compressionType, serializedKey, serializedValue, headers);
ensureValidRecordSize(serializedSize);
long timestamp = record.timestamp() == null ? nowMs : record.timestamp();
if (log.isTraceEnabled()) {
log.trace("Attempting to append record {} with callback {} to topic {} partition {}", record, callback, record.topic(), partition);
}
// producer callback will make sure to call both 'callback' and interceptor callback
Callback interceptCallback = new InterceptorCallback<>(callback, this.interceptors, tp);
if (transactionManager != null && transactionManager.isTransactional()) {
transactionManager.failIfNotReadyForSend();
}
// 真正把本地消息发送给RecordAccumulator
RecordAccumulator.RecordAppendResult result = accumulator.append(tp, timestamp, serializedKey,
serializedValue, headers, interceptCallback, remainingWaitMs, true, nowMs);
if (result.abortForNewBatch) {
int prevPartition = partition;
partitioner.onNewBatch(record.topic(), cluster, prevPartition);
partition = partition(record, serializedKey, serializedValue, cluster);
tp = new TopicPartition(record.topic(), partition);
if (log.isTraceEnabled()) {
log.trace("Retrying append due to new batch creation for topic {} partition {}. The old partition was {}", record.topic(), partition, prevPartition);
}
// producer callback will make sure to call both 'callback' and interceptor callback
interceptCallback = new InterceptorCallback<>(callback, this.interceptors, tp);
result = accumulator.append(tp, timestamp, serializedKey,
serializedValue, headers, interceptCallback, remainingWaitMs, false, nowMs);
}
if (transactionManager != null && transactionManager.isTransactional())
transactionManager.maybeAddPartitionToTransaction(tp);
if (result.batchIsFull || result.newBatchCreated) {
log.trace("Waking up the sender since topic {} partition {} is either full or getting a new batch", record.topic(), partition);
this.sender.wakeup();
}
return result.future;
// handling exceptions and record the errors;
// for API exceptions return them in the future,
// for other exceptions throw directly
} catch (ApiException e) {……
5.Consumer
使用 KafkaConsumer 需要先订阅(substribe)topic,再通过 poll 方法进行消息拉取。Consumer,是一个单线程机制,包括和 coordinator 通讯,rebalance, heartbeat 等,都是在单线程的 poll 函数里面。也因此,在 consumer 的代码中不需要任何锁。
public class KafkaConsumer<K, V> implements Consumer<K, V> {
private final String clientId;
private final Optional<String> groupId;
private final ConsumerCoordinator coordinator; // 消费者协调器,负责和服务器端GroupCoodinator通信
private final Deserializer<K> keyDeserializer;
private final Deserializer<V> valueDeserializer;
private final Fetcher<K, V> fetcher; // 负责组织拉取消息的请求,以及处理返回。
private final ConsumerInterceptors<K, V> interceptors;
private final Time time;
private final ConsumerNetworkClient client; // 负责消费者的网络IO,在NetworkClient之上进行封装
private final SubscriptionState subscriptions; // 管理此消费者订阅的主题分区,记录消息的各种状态
private final ConsumerMetadata metadata;
private final long retryBackoffMs;
private final long requestTimeoutMs;
private final int defaultApiTimeoutMs;
private volatile boolean closed = false;
private List<ConsumerPartitionAssignor> assignors; // 分区分配策略
}
KafkaConsumer 内部的几个重要组件:
SubScriptionState 来保存消费的状态;
ConsumerCoordinator 负责和 GroupCoordinator 通讯,例如在 leader 选举,入组,分区分配等过程。
ConsumerNetworkClient 是对 NetworkClient 的封装,如果你是从 producer 看过来的,想必对 NetworkClient 十分了解,他对 nio 的组件进行封装,实现网络 IO。
PartitionAssignor,这是分区分配策略,在进行分区分配的时候会用到。
Fetcher 负责组织拉取消息的请求,以及处理返回。
不过需要注意它并不做网络 IO,网络 IO 还是由 ConsumerNetworkClient 完成。它其实对应生产者中的 Sender。
启动过程
consumer = new KafkaConsumer<>(props);//构造一个KafkaConsumer
consumer.subscribe(Arrays.asList(topicName));//提交之
ConsumerRecords<String, byte[]> records = consumer.poll(100);//接收之
构造过程,配置自己的 client.id 和 group.id 然后配置各种个样的参数
String clientId = config.getString("client.id");
if (clientId.isEmpty()) {//clientId如果找不到,则利用AtomicInteger自增
clientId = "consumer-" + CONSUMER_CLIENT_ID_SEQUENCE.getAndIncrement();
}
this.clientId = clientId;
String groupId = config.getString("group.id");
消息拉取
这是消息拉取的入口方法,他会从上次消费的位置拉取消息,也可以手动指定消费位置。入参是阻塞的时长,如果有消息将会立即返回,否则会阻塞到超时,如果没有数据则返回空的数据集合。
private ConsumerRecords<K, V> poll(final Timer timer, final boolean includeMetadataInTimeout) {
acquireAndEnsureOpen(); // 1. 确保本对象是单线程进入,这是因为KafkaConsumer非线程安全。
try {
this.kafkaConsumerMetrics.recordPollStart(timer.currentTimeMs());
// 2. 检查是否订阅了topic
if (this.subscriptions.hasNoSubscriptionOrUserAssignment()) {
throw new IllegalStateException("Consumer is not subscribed to any topics or assigned any partitions");
}
// 3. 进入主循环,条件是没有超时
do {
client.maybeTriggerWakeup();
if (includeMetadataInTimeout) {
// try to update assignment metadata BUT do not need to block on the timer for join group
updateAssignmentMetadataIfNeeded(timer, false);
} else {
while (!updateAssignmentMetadataIfNeeded(time.timer(Long.MAX_VALUE), true)) {
log.warn("Still waiting for metadata");
}
}
// 4. 拉取一次消息。pollForFetches
final Map<TopicPartition, List<ConsumerRecord<K, V>>> records = pollForFetches(timer);
if (!records.isEmpty()) {
// before returning the fetched records, we can send off the next round of fetches
// and avoid block waiting for their responses to enable pipelining while the user
// is handling the fetched records.
//
// NOTE: since the consumed position has already been updated, we must not allow
// wakeups or any other errors to be triggered prior to returning the fetched records.
if (fetcher.sendFetches() > 0 || client.hasPendingRequests()) {
client.transmitSends();
}
return this.interceptors.onConsume(new ConsumerRecords<>(records));
}
} while (timer.notExpired());
return ConsumerRecords.empty();
} finally {
release();
this.kafkaConsumerMetrics.recordPollEnd(timer.currentTimeMs());
}
}
通过 acquireAndEnsureOpen()确保本对象是单线程进入,这是因为 KafkaConsumer 非线程安全。
检查是否订阅了 topic
进入主循环,条件是没有超时
在主循环中通过 pollForFetches()拉取一次消息。这个方法中先检查是否经存在拉取过的未加工消息,这是因为上一轮次拉取做了提前拉取处理。有可能已经拉取回消息等待处理。如果没有已拉取未加工数据,则准备新的拉取请求,网络 IO 拉取消息,加工拉取回来的数据。
如果上一步拉取到消息,并不会立即返回,而是再一次触发消息拉取,并且使用的是非阻塞方式,调用 client.pollNoWakeup()。这样做的目的是,提前网络 IO,把消息拉取请求发出去。在网络 IO 的同时,消息数据返回给 consumer 的调用者进行业务处理。这样做到了并行处理,提高了效率。等下次调用 KafkaConsumer 进行 poll,当进行到第 4 步时,有可能直接返回了上轮次提前拉取到的消息,从而省去了网络 IO 时间。
pollForFetches()方法:
这个方法完成了从服务端拉取消息的动作,这个过程主要使用了 Fetcher 和 ConsumerNetworkClient 两个组件。Fetcher 负责准备好拉取消息的 request、处理 response,并且把消息转化为对调用者友好的格式。ConsumerNetworkClient 负责把请求发送出去,接收返回,也就是网络 IO 工作。
**
* @throws KafkaException if the rebalance callback throws exception
*/
private Map<TopicPartition, List<ConsumerRecord<K, V>>> pollForFetches(Timer timer) {
long pollTimeout = coordinator == null ? timer.remainingMs() :
Math.min(coordinator.timeToNextPoll(timer.currentTimeMs()), timer.remainingMs());
// if data is available already, return it immediately
final Map<TopicPartition, List<ConsumerRecord<K, V>>> records = fetcher.fetchedRecords();
if (!records.isEmpty()) {
return records;
}
// send any new fetches (won't resend pending fetches)
fetcher.sendFetches();
// We do not want to be stuck blocking in poll if we are missing some positions
// since the offset lookup may be backing off after a failure
// NOTE: the use of cachedSubscriptionHashAllFetchPositions means we MUST call
// updateAssignmentMetadataIfNeeded before this method.
if (!cachedSubscriptionHashAllFetchPositions && pollTimeout > retryBackoffMs) {
pollTimeout = retryBackoffMs;
}
log.trace("Polling for fetches with timeout {}", pollTimeout);
Timer pollTimer = time.timer(pollTimeout);
client.poll(pollTimer, () -> {
// since a fetch might be completed by the background thread, we need this poll condition
// to ensure that we do not block unnecessarily in poll()
return !fetcher.hasAvailableFetches();
});
timer.update(pollTimer.currentTimeMs());
return fetcher.fetchedRecords();
}
主要流程是如下四步:
1、查看是否已经存在拉取回来未加工的消息原始数据,有的话立即调用 fetcher.fetchedRecords()加工,然后返回。
2、如果没有未加工的原始数据,那么调用 fetcher.sendFetches()准备拉取请求。
3、通过 ConsumerNetworkClient 发送拉取请求。
4、加工拉取回的原始数据,返回。
版权声明: 本文为 InfoQ 作者【InfoQ_Springup】的原创文章。
原文链接:【http://xie.infoq.cn/article/5411b4f9dab5d7a7bf0d3a0ee】。文章转载请联系作者。
还未添加个人签名 2019.02.22 加入
还未添加个人简介
评论