HiveSQL 优化方法
- 列裁剪和分区裁剪 -
最基本的操作。所谓列裁剪就是在查询时只读取需要的列,分区裁剪就是只读取需要的分区。以我们的日历记录表为例:
当列很多或者数据量很大时,如果 select *或者不指定分区,全列扫描和全表扫描效率都很低。Hive 中与列裁剪优化相关的配置项是hive.optimize.cp
,与分区裁剪优化相关的则是hive.optimize.pruner
,默认都是 true。在 HiveQL 解析阶段对应的则是 ColumnPruner 逻辑优化器。
- 谓语下推 -
在关系型数据库如 MySQL 中,也有谓词下推(Predicate Pushdown,PPD)的概念。它就是将 SQL 语句中的 where 谓词逻辑都尽可能提前执行,减少下游处理的数据量。例如以下 HiveQL 语句:
对 forum_topic 做过滤的 where 语句写在子查询内部,而不是外部。Hive 中有谓词下推优化的配置项hive.optimize.ppd
,默认值 true,与它对应的逻辑优化器是 PredicatePushDown。该优化器就是将 OperatorTree 中的 FilterOperator 向上提,见下图。
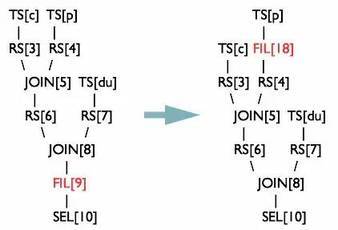
图来自https://tech.meituan.com/2014/02/12/hive-sql-to-mapreduce.html
上面的链接中是一篇讲解 HiveQL 解析与执行过程的好文章,前文提到的优化器、OperatorTree 等概念在其中也有详细的解释,非常推荐。
sort by 代替 order by
HiveQL 中的 order by 与其他 SQL 方言中的功能一样,就是将结果按某字段全局排序,这会导致所有 map 端数据都进入一个 reducer 中,在数据量大时可能会长时间计算不完。如果使用 sort by,那么还是会视情况启动多个 reducer 进行排序,并且保证每个 reducer 内局部有序。为了控制 map 端数据分配到 reducer 的 key,往往还要配合 distribute by 一同使用。如果不加 distribute by 的话,map 端数据就会随机分配到 reducer。举个例子,假如要以 UID 为 key,以上传时间倒序、记录类型倒序输出记录数据:
group by 代替 distinct
当要统计某一列的去重数时,如果数据量很大,count(distinct)就会非常慢,原因与 order by 类似,count(distinct)逻辑只会有很少的 reducer 来处理。这时可以用 group by 来改写:
但是这样写会启动两个 MR job(单纯 distinct 只会启动一个),所以要确保数据量大到启动 job 的 overhead 远小于计算耗时,才考虑这种方法。当数据集很小或者 key 的倾斜比较明显时,group by 还可能会比 distinct 慢。那么如何用 group by 方式同时统计多个列?下面是解决方法:
group by 配置调整
map 端预聚合
group by 时,如果先起一个 combiner 在 map 端做部分预聚合,可以有效减少 shuffle 数据量。预聚合的配置项是hive.map.aggr
,默认值 true,对应的优化器为 GroupByOptimizer,简单方便。通过hive.groupby.mapaggr.checkinterval
参数也可以设置 map 端预聚合的行数阈值,超过该值就会分拆 job,默认值 100000。
倾斜均衡配置项
group by 时如果某些 key 对应的数据量过大,就会发生数据倾斜。Hive 自带了一个均衡数据倾斜的配置项hive.groupby.skewindata
,默认值 false。其实现方法是在 group by 时启动两个 MR job。第一个 job 会将 map 端数据随机输入 reducer,每个 reducer 做部分聚合,相同的 key 就会分布在不同的 reducer 中。第二个 job 再将前面预处理过的数据按 key 聚合并输出结果,这样就起到了均衡的效果。但是,配置项毕竟是死的,单纯靠它有时不能根本上解决问题,因此还是建议自行了解数据倾斜的细节,并优化查询语句。
join 基础优化
join 优化是一个复杂的话题,下面先说 5 点最基本的注意事项。
build table(小表)前置
在最常见的 hash join 方法中,一般总有一张相对小的表和一张相对大的表,小表叫 build table,大表叫 probe table。如下图所示。
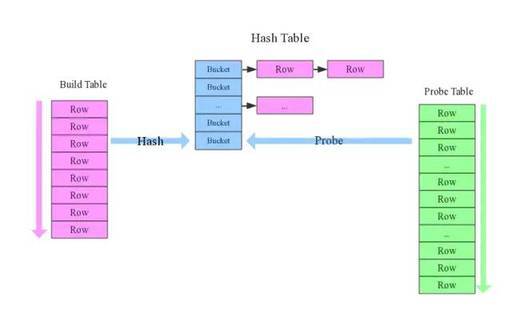
Hive 在解析带 join 的 SQL 语句时,会默认将最后一个表作为 probe table,将前面的表作为 build table 并试图将它们读进内存。如果表顺序写反,probe table 在前面,引发 OOM 的风险就高了。在维度建模数据仓库中,事实表就是 probe table,维度表就是 build table。假设现在要将日历记录事实表和记录项编码维度表来 join:
- 多表 join 时 key 相同 -
这种情况会将多个 join 合并为一个 MR job 来处理,例如:
如果上面两个 join 的条件不相同,比如改成a.event_code = c.event_code
,就会拆成两个 MR job 计算。负责这个的是相关性优化器 CorrelationOptimizer,它的功能除此之外还非常多,逻辑复杂,参考 Hive 官方的文档可以获得更多细节:https://cwiki.apache.org/confluence/display/Hive/Correlation+Optimizer。
- 利用 map join 特性 -
map join 特别适合大小表 join 的情况。Hive 会将 build table 和 probe table 在 map 端直接完成 join 过程,消灭了 reduce,效率很高。
上面的语句中加了一条 map join hint,以显式启用 map join 特性。早在 Hive 0.8 版本之后,就不需要写这条 hint 了。map join 还支持不等值连接,应用更加灵活。map join 的配置项是hive.auto.convert.join
,默认值 true,对应逻辑优化器是 MapJoinProcessor。还有一些参数用来控制 map join 的行为,比如hive.mapjoin.smalltable.filesize
,当 build table 大小小于该值就会启用 map join,默认值 25000000(25MB)。还有hive.mapjoin.cache.numrows
,表示缓存 build table 的多少行数据到内存,默认值 25000。
分桶表 map join
map join 对分桶表还有特别的优化。由于分桶表是基于一列进行 hash 存储的,因此非常适合抽样(按桶或按块抽样)。它对应的配置项是hive.optimize.bucketmapjoin
,优化器是 BucketMapJoinOptimizer。但我们的业务中用分桶表较少,所以就不班门弄斧了,只是提一句。
倾斜均衡配置项
这个配置与上面 group by 的倾斜均衡配置项异曲同工,通过hive.optimize.skewjoin
来配置,默认 false。如果开启了,在 join 过程中 Hive 会将计数超过阈值hive.skewjoin.key
(默认 100000)的倾斜 key 对应的行临时写进文件中,然后再启动另一个 job 做 map join 生成结果。通过hive.skewjoin.mapjoin.map.tasks
参数还可以控制第二个 job 的 mapper 数量,默认 10000。再重复一遍,通过自带的配置项经常不能解决数据倾斜问题。join 是数据倾斜的重灾区,后面还要介绍在 SQL 层面处理倾斜的各种方法。
优化 SQL 处理 join 数据倾斜
上面已经多次提到了数据倾斜,包括已经写过的 sort by 代替 order by,以及 group by 代替 distinct 方法,本质上也是为了解决它。join 操作更是数据倾斜的重灾区,需要多加注意。
空值或无意义值
这种情况很常见,比如当事实表是日志类数据时,往往会有一些项没有记录到,我们视情况会将它置为 null,或者空字符串、-1 等。如果缺失的项很多,在做 join 时这些空值就会非常集中,拖累进度。因此,若不需要空值数据,就提前写 where 语句过滤掉。需要保留的话,将空值 key 用随机方式打散,例如将用户 ID 为 null 的记录随机改为负值:
单独处理倾斜 key
这其实是上面处理空值方法的拓展,不过倾斜的 key 变成了有意义的。一般来讲倾斜的 key 都很少,我们可以将它们抽样出来,对应的行单独存入临时表中,然后打上一个较小的随机数前缀(比如 0~9),最后再进行聚合。SQL 语句与上面的相仿,不再赘述。
不同数据类型
这种情况不太常见,主要出现在相同业务含义的列发生过逻辑上的变化时。举个例子,假如我们有一旧一新两张日历记录表,旧表的记录类型字段是(event_type int),新表的是(event_type string)。为了兼容旧版记录,新表的 event_type 也会以字符串形式存储旧版的值,比如'17'。当这两张表 join 时,经常要耗费很长时间。其原因就是如果不转换类型,计算 key 的 hash 值时默认是以 int 型做的,这就导致所有“真正的”string 型 key 都分配到一个 reducer 上。所以要注意类型转换:
build table 过大
有时,build table 会大到无法直接使用 map join 的地步,比如全量用户维度表,而使用普通 join 又有数据分布不均的问题。这时就要充分利用 probe table 的限制条件,削减 build table 的数据量,再使用 map join 解决。代价就是需要进行两次 join。举个例子:
版权声明: 本文为 InfoQ 作者【大数据技术指南】的原创文章。
原文链接:【http://xie.infoq.cn/article/139ee7201afaf5075ed2d14bf】。
本文遵守【CC-BY 4.0】协议,转载请保留原文出处及本版权声明。
评论