LangChain 系列 -03. LLM 聊天模型
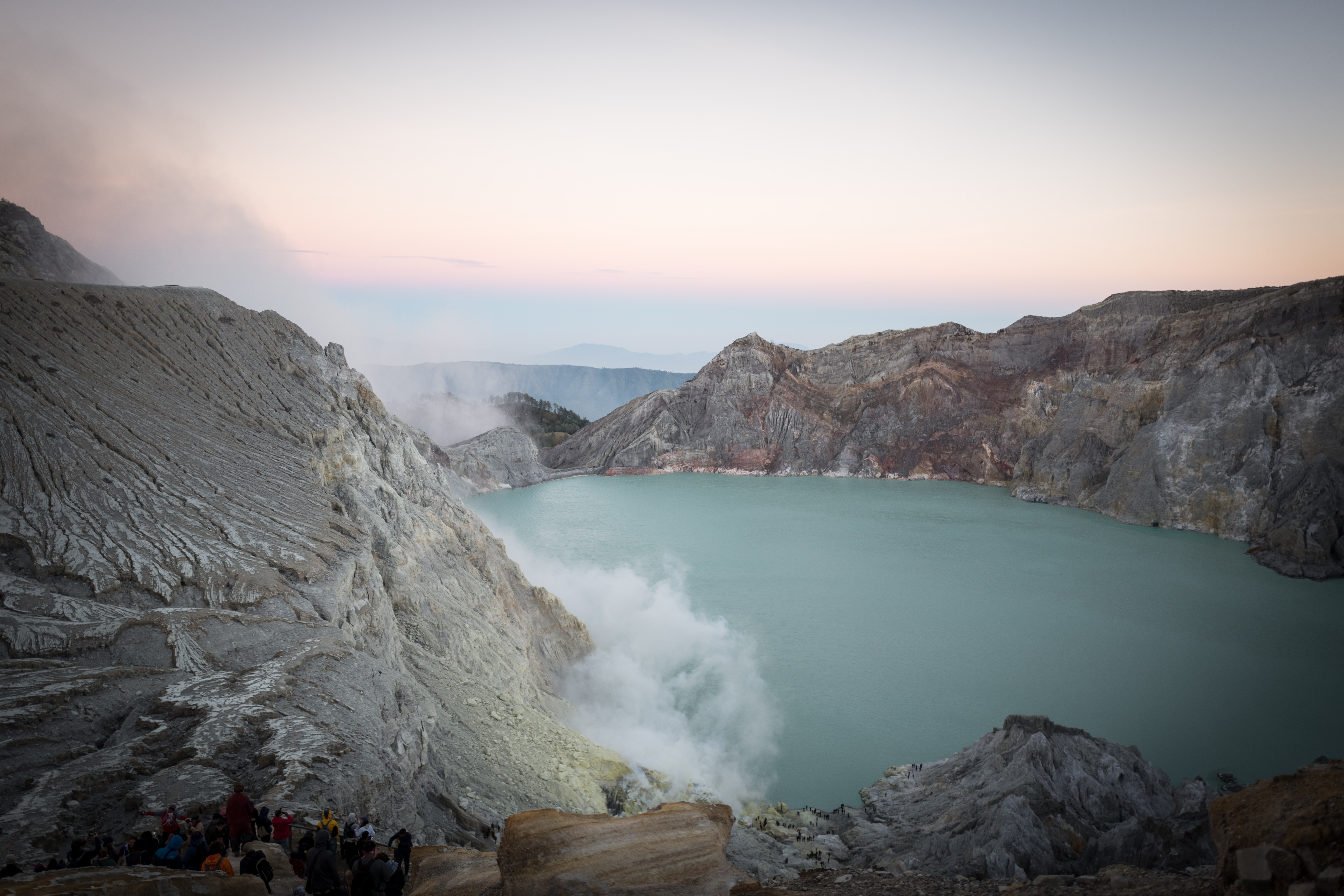
Chat Models
上面的 LLM 例子都是使用的 text in, text out 方式,直接向 LLM 输入 prompt text,让其返回 generation text.
此外,LangChain 还提供对话模型,它是 LLM 的一个变种,其底层还是 OpenAI 这样的 LLM,只是其编程接口与 text in, text out 的风格有所不同,它使用的是 chat messages 作为输入和输出。
我们先看一下原来的 OpenAI 模型:

注意上面的代码运行时会有一个 warning:
UserWarning: You are trying to use a chat model. This way of initializing it is no longer supported. Instead, please use: from langchain.chat_models import ChatOpenAI
也就是说 gpt-3.5-turbo 本身是一个对话模型,需要用 chat_models 的接口来调用。下面是对应的代码:
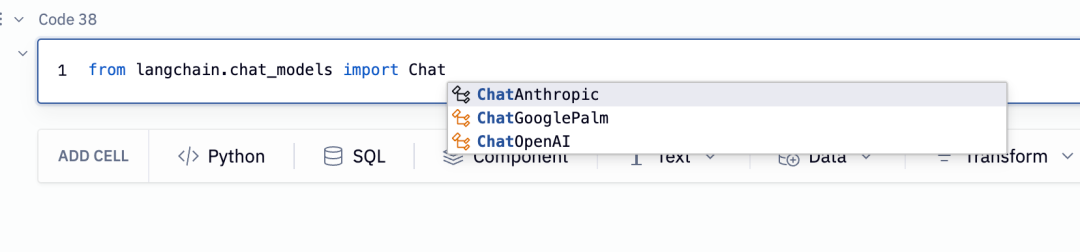
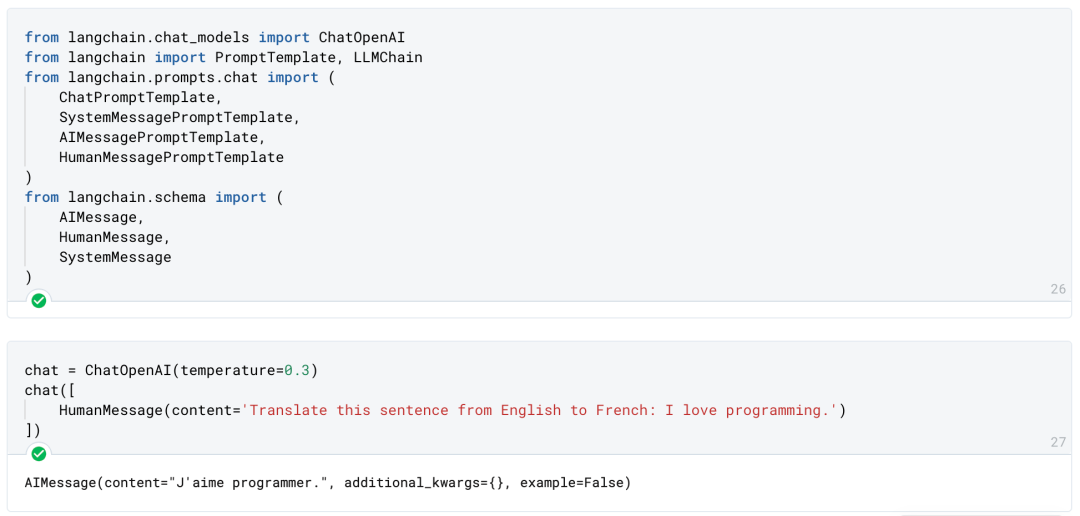
对话模型接受一个 Message 的数组作为输入,现在支持的 Message 类型包括:
AIMessage: AI 模型输出的 Message
HumanMessage: 用户输入的 Message,往往作为 in-context learning 的输入,给 AI 做 few-shot
SystemMessage: 设定 AI 模型的 role 用到的 Message
ChatMessage: 接受任意角色参数
一般只用到 AIMessage、HumanMessage 和 SystemMessage。下面是一个例子:

可以一次传多组信息
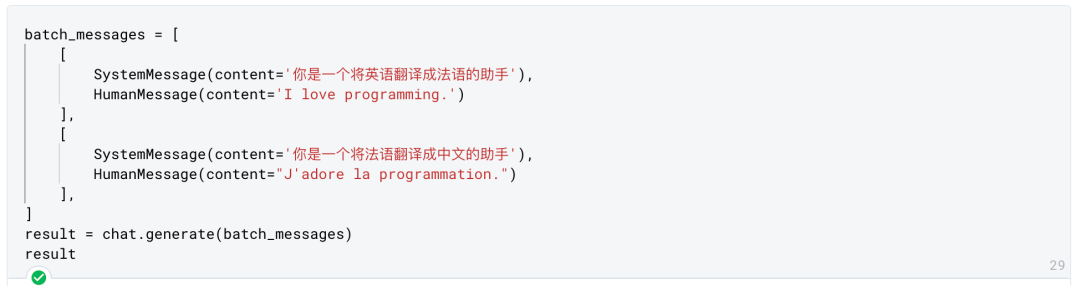


同样可以通过 llm_output 获取 token 使用情况:

用 PromptTemplate 来生成 Prompt
可以借助 PromptTemplate 来生成对话的 Prompt。下面是一个例子:想要将一种语言翻译成另一种语言。

将"I love programming." 从英语翻译成意大利语,得到:"Amo programmare."
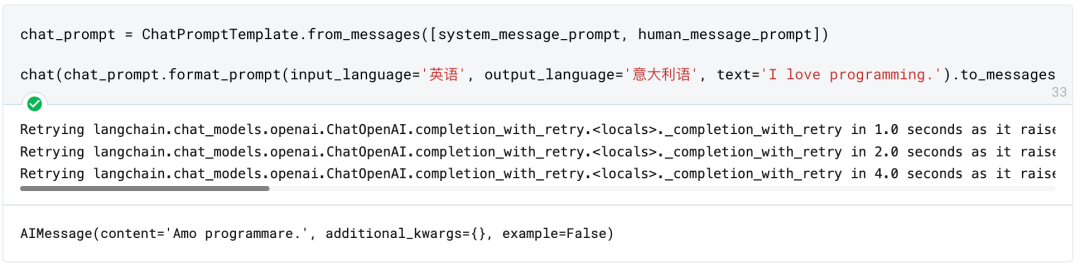
我也不知道是否正确,为了验证,将其从意大利语再翻译成中文,得到“我喜欢编程。”,这就对了!
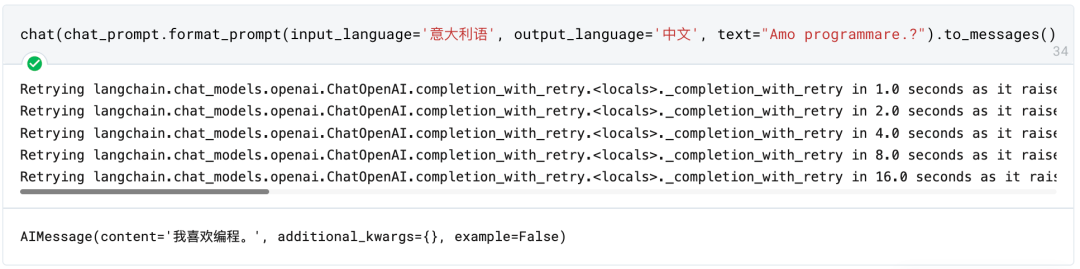
上面使用了 SystemMessagePromptTemplate、HumanMessagePromptTemplate、ChatPromptTemplate 来使用 PromptTemplate,还能更直接使用 PromptTemplate 来创建:

后续会有更详尽的教程来探讨 Prompt 的生成。
使用 LLMChain 来对话
可以直接使用 LLMChain 这个 Chain 来与 LLM 对话,它将 llm 与 prompt 串联起来。
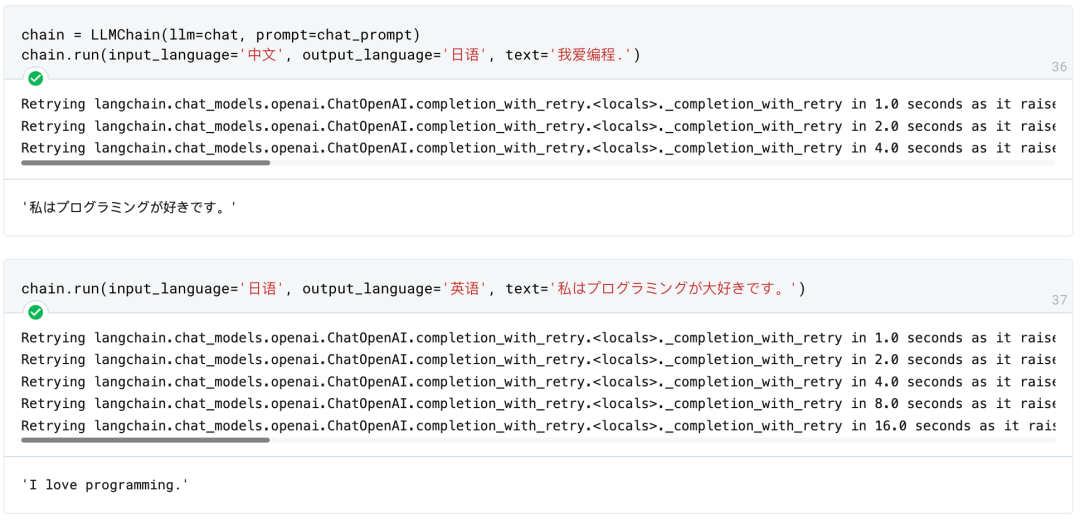
Streaming 返回
可以通过 ChatOpenAI 的回调出来实现对话流式输出,它会逐个字符输出结果:
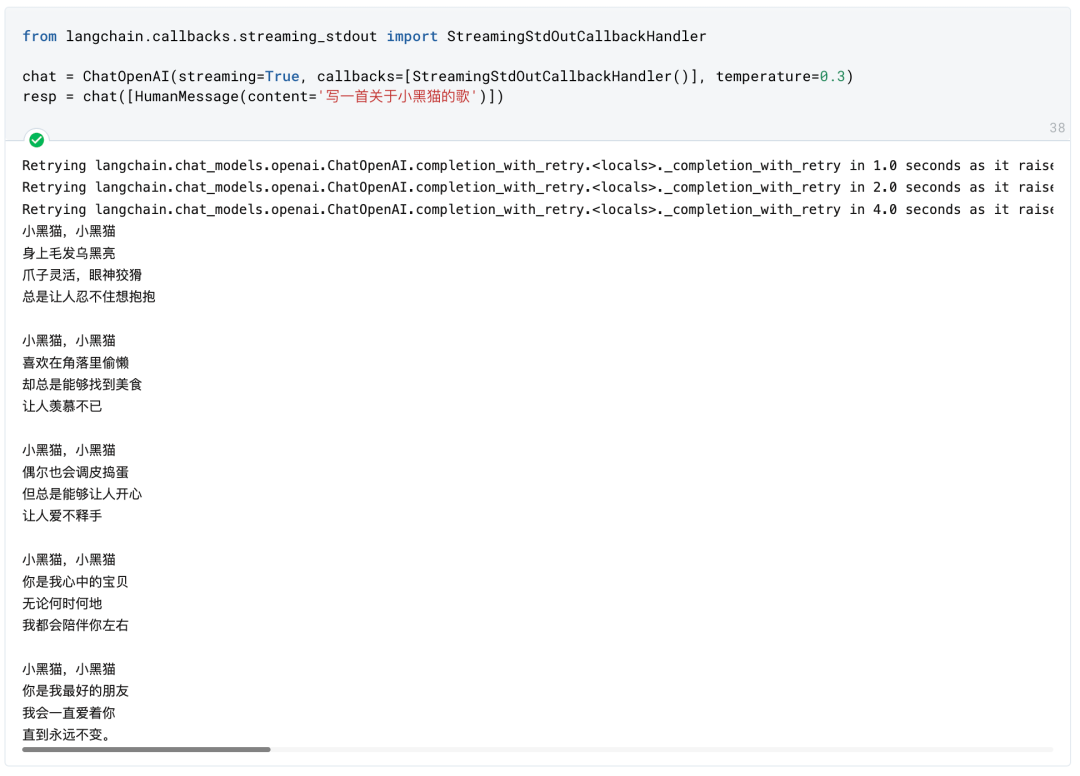
未完待续。。。
版权声明: 本文为 InfoQ 作者【无人之路】的原创文章。
原文链接:【http://xie.infoq.cn/article/6c6dfc65f7bc9ebf05484173c】。文章转载请联系作者。
评论