YOLOP 多任务算法详解
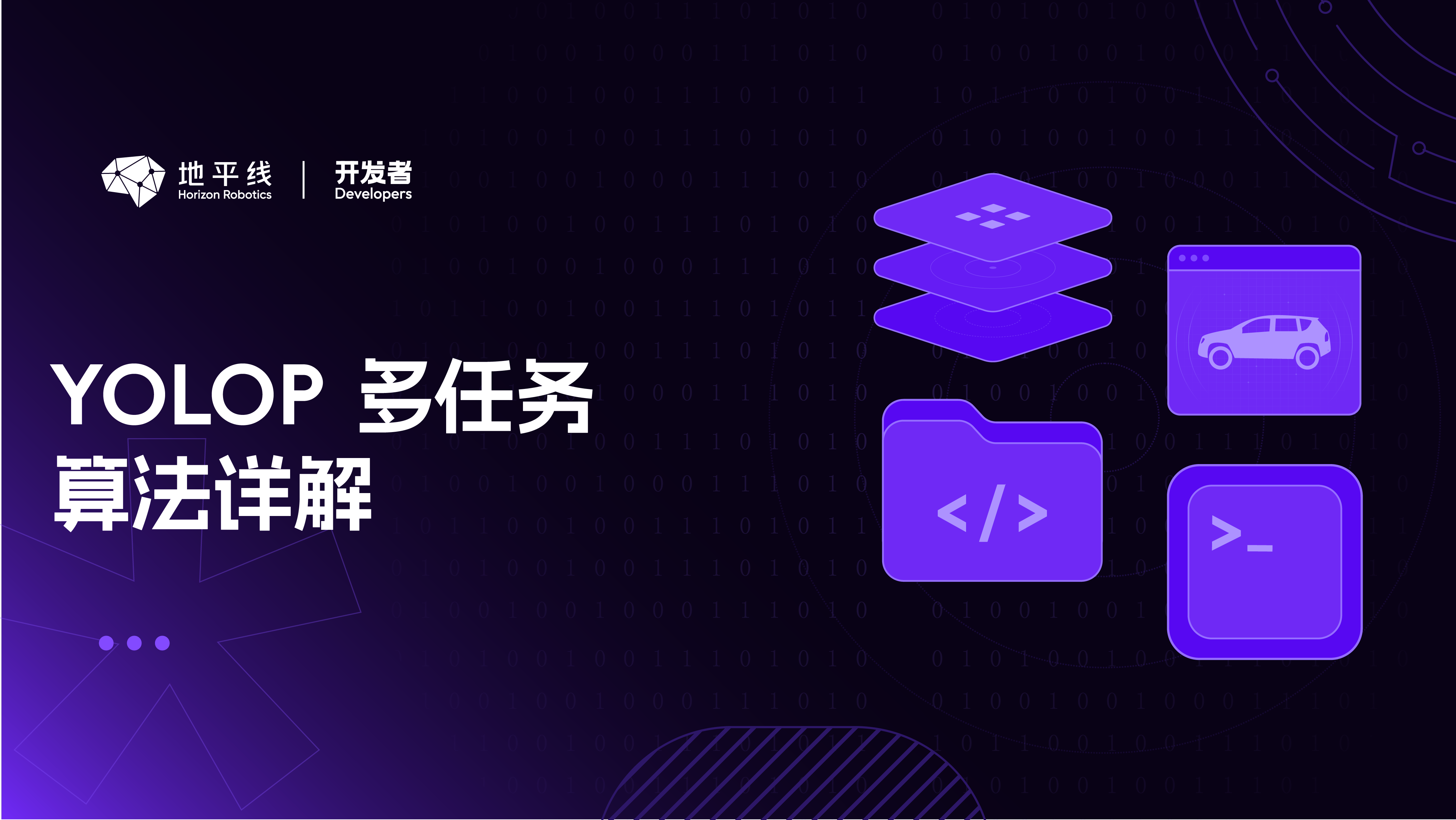
YOLOP 是华中科技大学研究团队在 2021 年开源的研究成果,其将目标检测/可行驶区域分割和车道线检测三大视觉任务同时放在一起处理,并且在 Jetson TX2 开发板子上能够达到 23FPS。
论文标题:YOLOP You Only Look Once for Panoptic Driving Perception
论文地址:https://arxiv.org/abs/2108.11250
官方代码:https://github.com/hustvl/YOLOP
01 网络结构
YOLOP 的核心亮点就是多任务学习,而各部分都是拿其它领域的成果进行缝合,其网络结构如下图所示
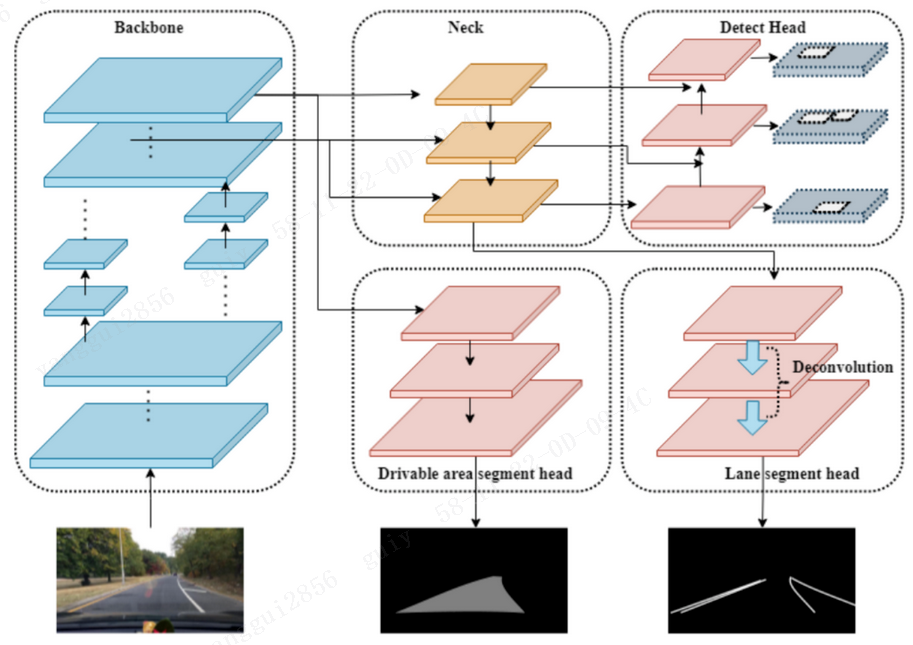
三个子任务共用一个 Backbone 和 Neck,然后分出来三个头来执行不同的任务。
1.1 Encoder
根据论文所述,整个网络可以分成一个 Encoder 和 3 个 Decoder。
Encoder 包含 Backbone 和 Neck,Backbone 照搬了 YOLOv4 所采用的 CSPDarknet,Neck 也和 YOLOv4 类似,使用了空间金字塔(SPP)模块和特征金字塔网络(FPN)模块。
1.2 Decoders
Decoders 即三个任务头:
Detect Head
目标检测头使用了 Path Aggregation Network (PAN)结构,这个结构可以将多个尺度特征图的特征图进行融合,其实还是 YOLOv4 那一套。
Drivable Area Segment Head & Lane Line Segment Head
可行驶区域分割头和车道线检测头都属于语义分割任务,因此 YOLOP 使用了相同的网络结构,经过三次上采样,将输出特征图恢复为(W, H, 2)的大小,再进行具体任务的处理。
1.3 Loss Function
损失函数包括三部分,即三个任务的损失。
目标检测损失
目标检测是直接照搬 YOLOv4 的,因此和 YOLOv4 采用的损失一样,经典的边界框损失、目标损失和类别损失,各自加了个权重。
2.语义分割损失
另外两个语义分割损失采用的均是交叉熵损失。
总体损失,总体损失为三部分损失之和:

02 代码结构
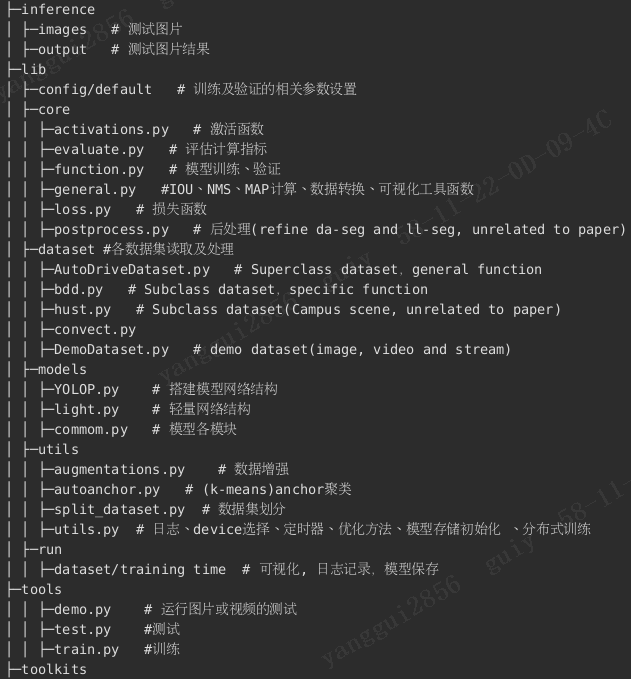
03 训练--tools/train.py
3.1 设置 DDP 参数
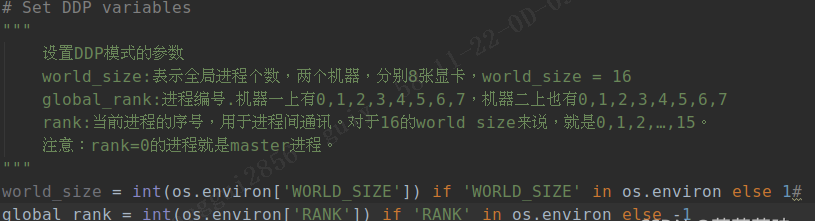
pytorch 中 DDP 使用:
(1)参数加载;
(2)模型转换成 DDP 模型;
(3)训练数据 sampler,来使得各个进程上的数据各不相同;
(4)分布式模型的保存。
3.2 读取网络结构

3.3 定义损失函数及优化器

3.4 网络结构划分
用于单任务训练固定其他网络部分层。

3.5 初始化学习率
后续在 train()中 warmup 会调整学习率。

首先定义一个优化器,定义好优化器以后,就可以给这个优化器绑定一个指数衰减学习率控制器。
参数:
04 dataset/bdd.py 文件
4.1 数据读取
1.该文件继承 AutoDriveDataset.py。

2、按比例缩放操作:letterbox()图像增加灰边
3、数据增强操作
4.2 数据增强
utils/utils.py 文件:
random_perspective()放射变换增强
augment_hsv()颜色 HSV 通道增强
cutout()
05 models/YOLOP.py 文件

YOLOP 包括三个检测任务,目标检测+可行驶区域检测+车道线检测。
06 损失函数
build_targets 思想:
build_targets 主要为了拿到所有 targets(扩充了周围 grids)对应的类别,框,batch 中图片数索引和 anchor 索引,以及具体的 anchors。
每个 gt 按照正样本选取策略,生成相应的 5 个框,再根据与默认 anchor 匹配,计算宽高的比例值,根据阈值过滤不相符的框,得到最终正样本。
6.1 目标检测损失
predictions[0] 目标检测分支[[4,3,48,80,6],[4,3,24,40,6],[4,3,12,20,6]]。
targets[0] 目标检测标签 [32,6],格式为[batch_num,class,x1,y1,x2,y2]。根据 build_targets 在每个检测层生成 相 应的正样本 tbox[]。
将每层的预测结果 tensor pi 根据正样本格式得到 ps = pi[b, a, gj, gi]。
计算每个检测层预测与正样本之间的 ciou 坐标损失。
obj 损失:
![]()
cls 类别损失:
![]()
6.2 可行驶区域损失
6.3 车道线损失
07 网络模型输出格式形式
7.1 网络模型检测输出格式
det_out:障碍物检测输出格式:[25200,6] 其中 6 表示[x1,y1,x2,y2,conf,cls],25200 :(80x80+40x40+20x20)x3。
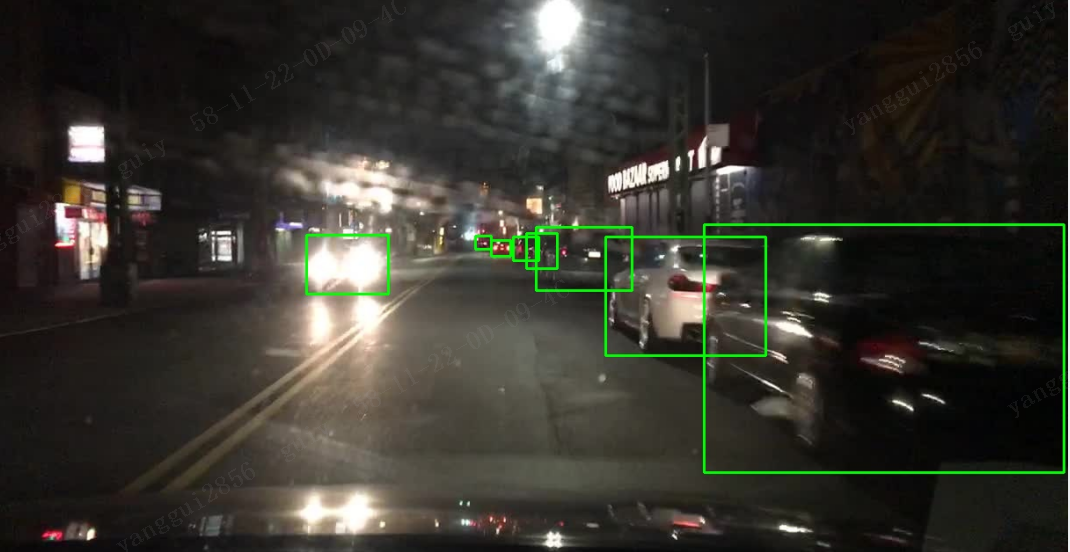
7.2 网络模型车道线输出格式
lane_line_seg : 车道线分割输出格式:1,2,640,640。
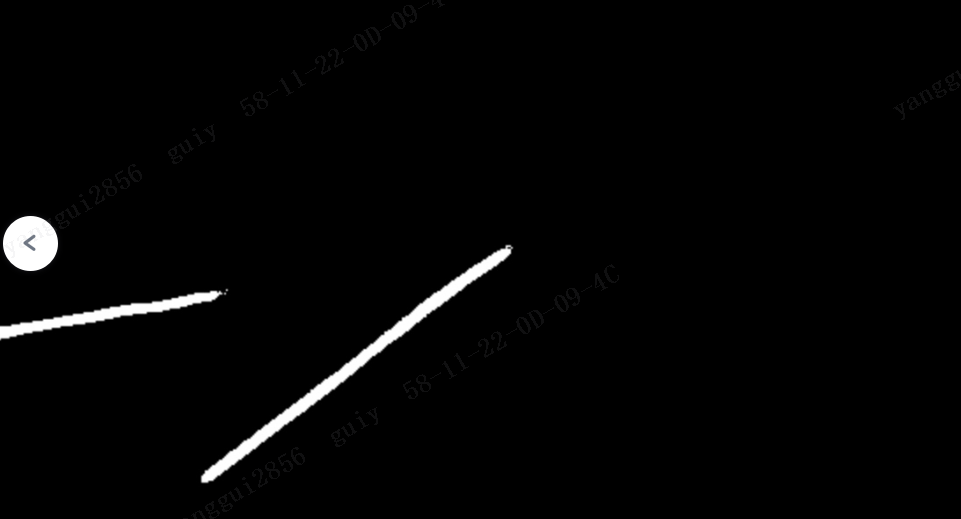
7.3 网络模型可行驶区域输出格式
drive_area_seg : 可行驶区域分割输出格式:1,2,640,640。
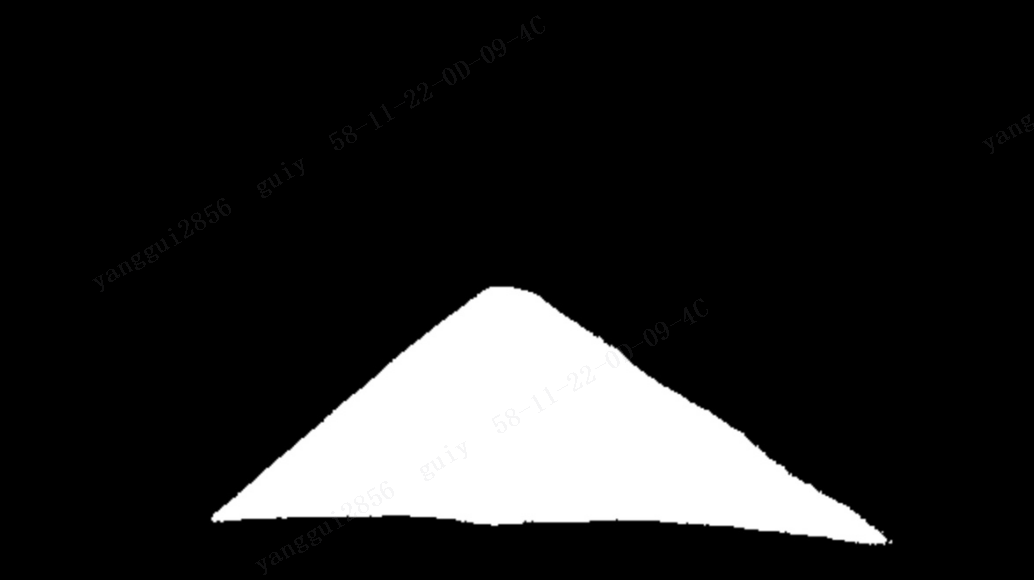
08 前视停车场数据集检测效果
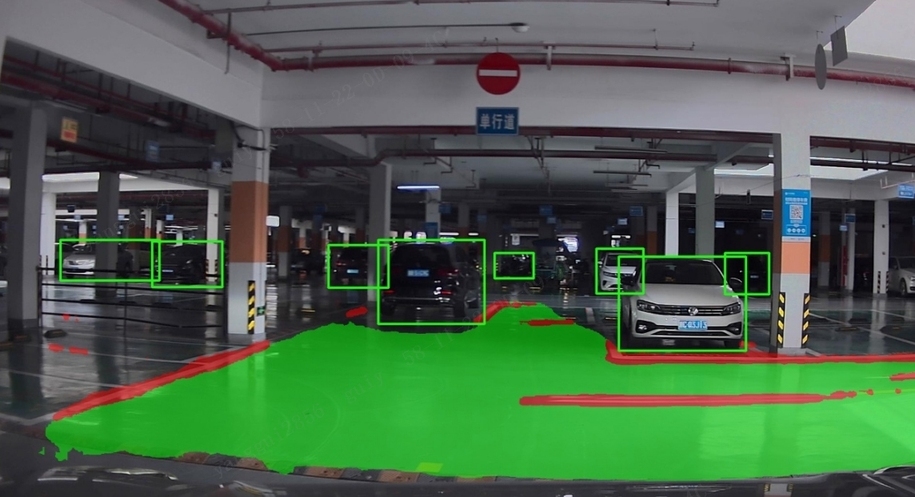
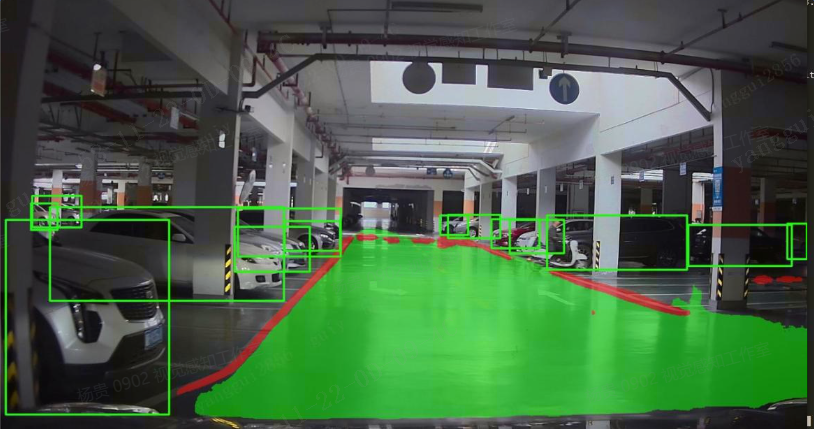
评论