通过 ModelScope 开源多模态 Embedding 模型进行向量生成
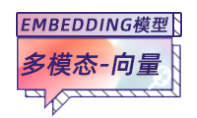
本文介绍如何通过ModelScope魔搭社区中的多模态表征开源模型进行 多模态向量生成 ,并入库至向量检索服务 DashVector 中进行向量检索。
ModelScope魔搭社区旨在打造下一代开源的模型即服务共享平台,为泛 AI 开发者提供灵活、易用、低成本的一站式模型服务产品,让模型应用更简单。
ModelScope 魔搭社区的愿景是汇集行业领先的预训练模型,减少开发者的重复研发成本,提供更加绿色环保、开源开放的 AI 开发环境和模型服务,助力绿色"数字经济"事业的建设。 ModelScope 魔搭社区将以开源的方式提供多类优质模型,开发者可在平台上免费体验与下载使用。
在 ModelScope 魔搭社区,您可以:
免费使用平台提供的预训练模型,支持免费下载运行
一行命令实现模型预测,简单快速验证模型效果
用自己的数据对模型进行调优,定制自己的个性化模型
学习系统性的知识,结合实训,有效提升模型研发能力
分享和贡献你的想法、评论与模型,让更多人认识你,在社区中成长
前提条件
DashVector:
已创建 Cluster
已获得 API-KEY
已安装最新版 SDK
ModelScope
已安装最新版 SDK:
pip install -U modelscope
CLIP 模型
简介
本项目为CLIP模型的中文版本,使用大规模中文数据进行训练( ~2 亿图文对 ),可用于图文检索和图像、文本的表征提取,应用于搜索、推荐等应用场景。
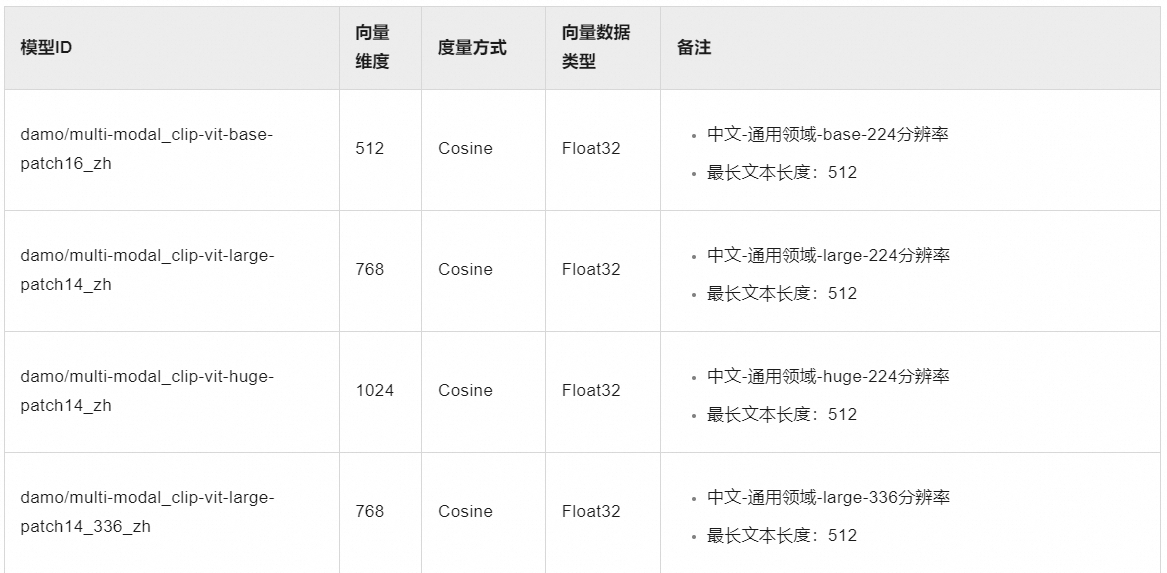
关于 CLIP 模型更多信息请参考:CLIP模型
使用示例
说明
需要进行如下替换代码才能正常运行:
DashVector api-key 替换示例中的{your-dashvector-api-key}
DashVector Cluster Endpoint 替换示例中的{your-dashvector-cluster-endpoint}
使用上表中 模型 ID 替换示例中的{model_id}
使用上表中 向量维度 替换示例中的{model_dim}
Python 示例:
版权声明: 本文为 InfoQ 作者【DashVector】的原创文章。
原文链接:【http://xie.infoq.cn/article/93b5b0c63bdf2298b5cb504ed】。文章转载请联系作者。
评论