利用 Python 语言调用腾讯混元大模型接口实战指南
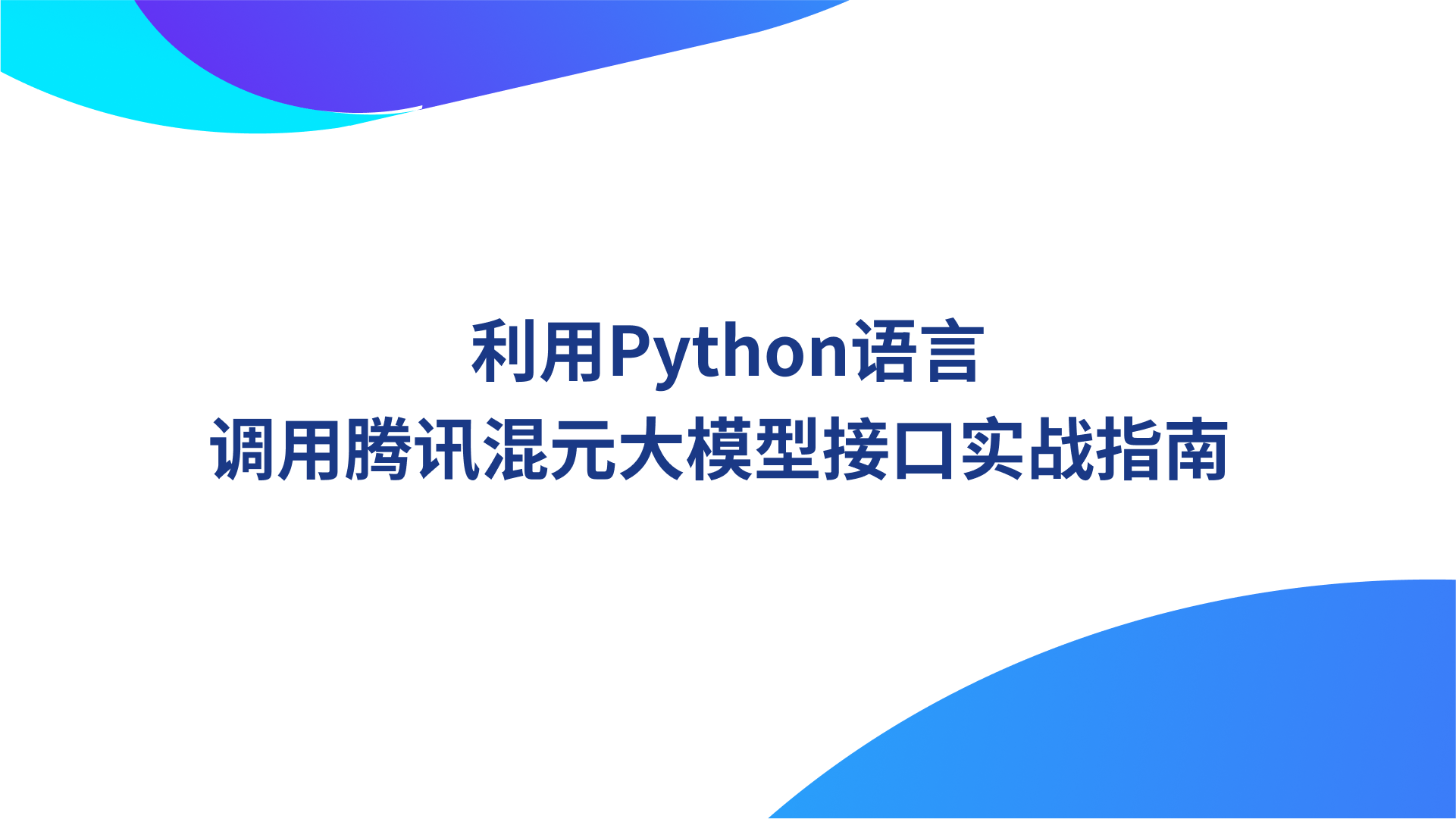
什么是混元大模型
“腾讯元器”是基于腾讯混元大模型的一站式智能体制作平台,支持通过下述能力对大模型进行增强:
提示词,包含详细设定(system prompt),开场白,建议引导问题。
插件(外部 API),目前支持勾选多个插件。官方插件包含微信搜一搜、PDF 摘要 &解析、混元图片生成,也支持用户自定义插件。
知识库,当前版本支持 doc 和 txt 两种格式。
工作流,一种“流程图”式的低代码编辑工具,可以用来做一个“高级版”插件。在工作流里,可以任意编排插件、知识库、大模型节点的工作顺序和调用传参,从而精确控制智能体中部分任务的运行逻辑。
通过元器平台制作的智能体,目前支持 32k token 上下文长度(某次回答过程中的提示词+机器回答的 token 长度,一个 token 约为 1.8 个中文字符)。工作流的超时运行时间为 5 分钟。智能体的回复上限时间是 90s。
什么是 API 接口
API是一个软件解决方案,作为中介,使两个应用程序能够相互交互。以下一些特征让 API 变得更加有用和有价值:
遵守 REST 和 HTTP 等易于访问、广泛理解和开发人员友好的标准。
API 不仅仅是几行代码;这些是为移动开发人员等特定受众创建的。
这些有清晰的文档和版本,以满足用户的期望。
更好的治理和安全性,以及监控以管理性能和可扩展性。
Python 编写 API 接口的主要步骤如下:
登录并注册账号
可以通过 幂简集成-API HUB 快速找到大量 AI 技术相关 API,心仪的 API 可以在登录后添加到个人书签,便于下次使用快速查找。
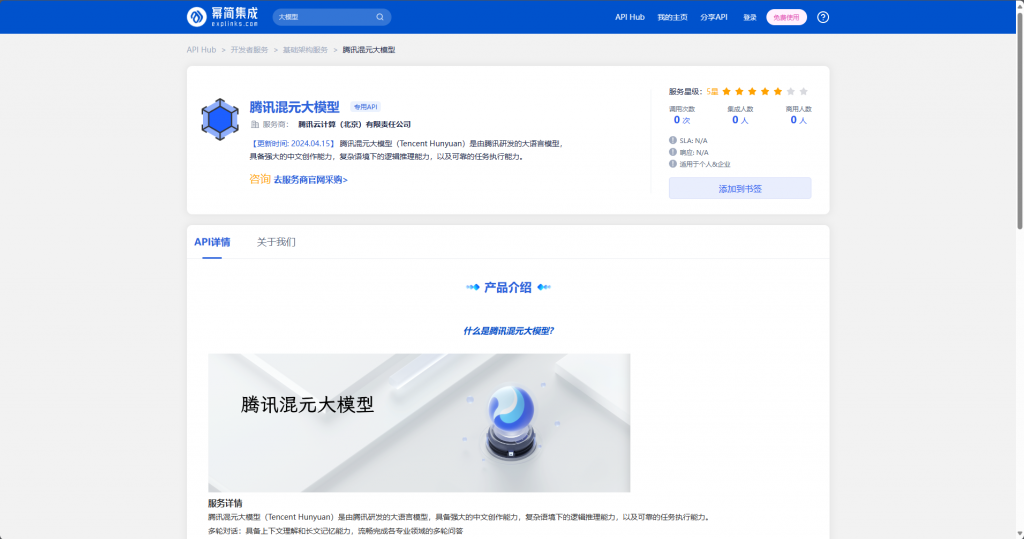
点击跳转 腾讯混元 官网,点击“腾讯元器”,输入手机号码并通过验证吗登录。
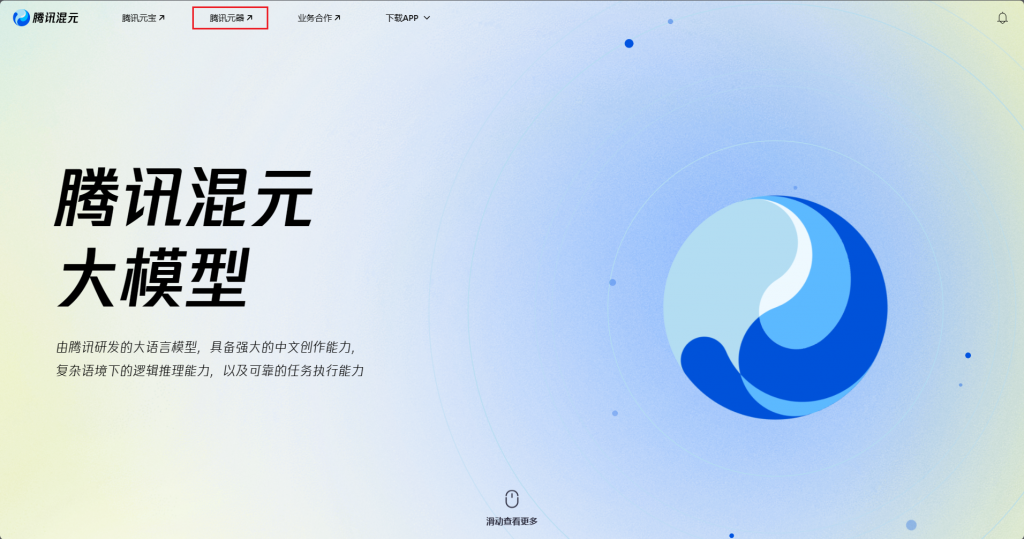
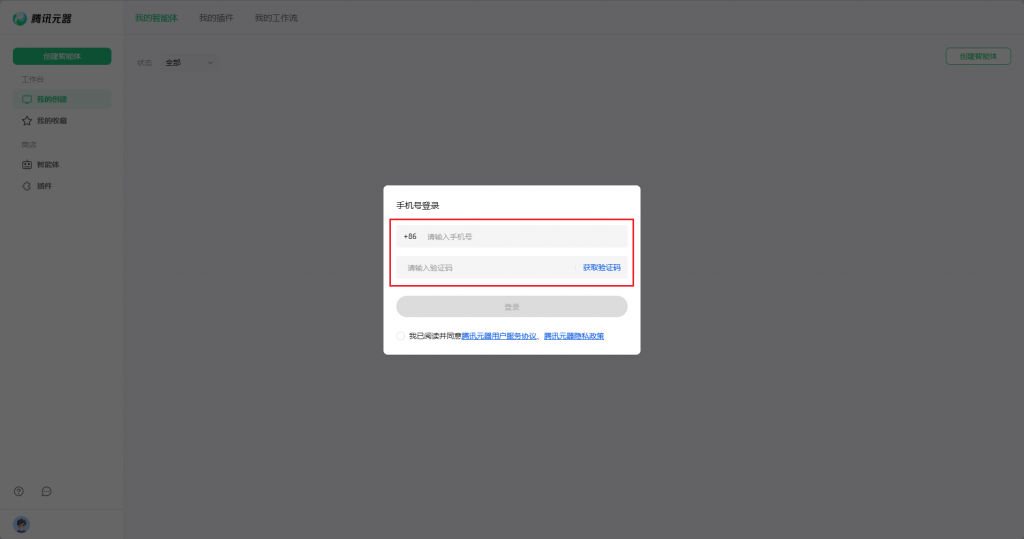
创建智能体
登录成功后,点击“创建智能体”
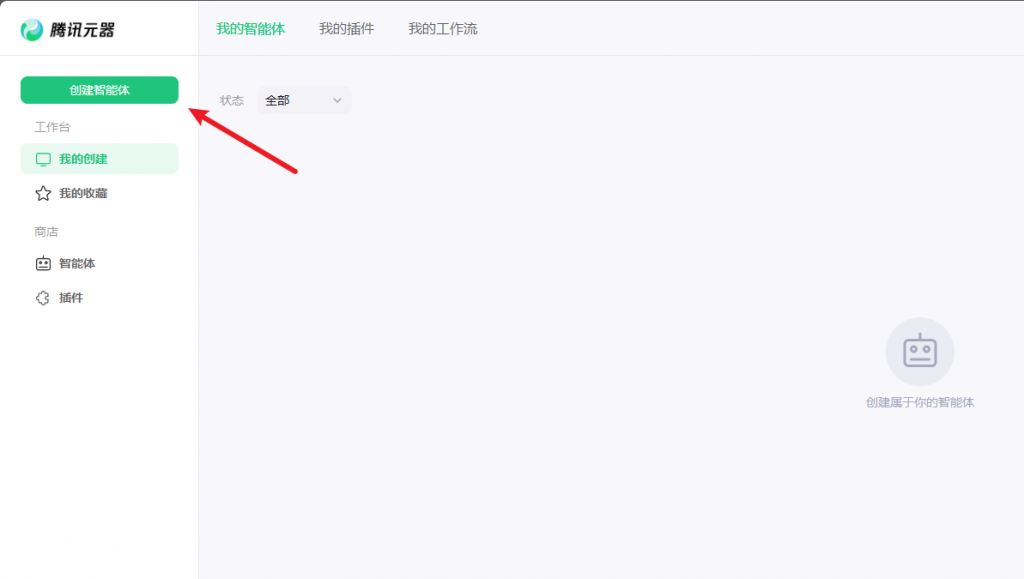
输入要创建智能体的名称、简介、头像(可以 AI 生成)、详细设定等相关信息~
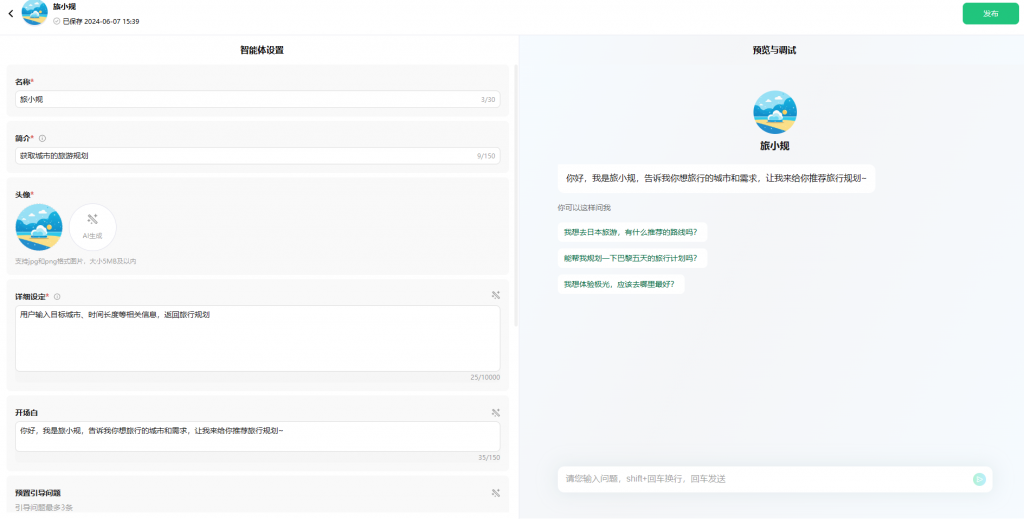
还可以添加丰富的插件、知识库、工作流等~
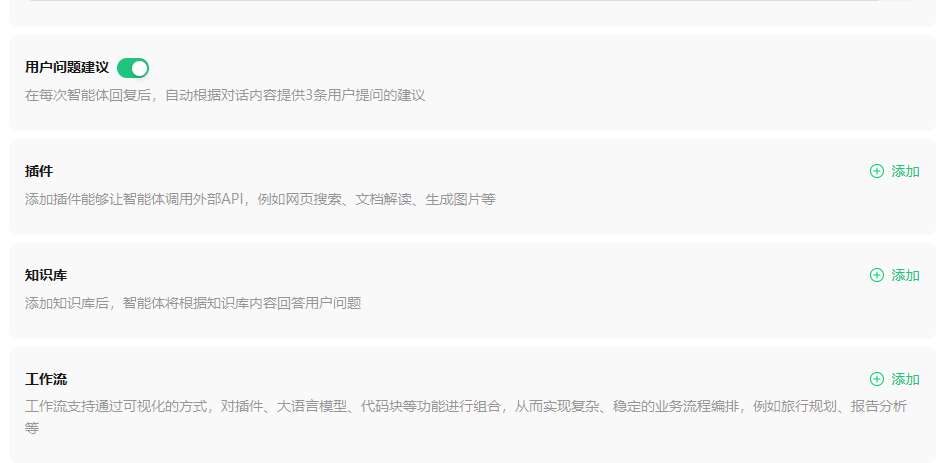
每一项表单的编辑后,右边会自动更新智能体的配置(预计有 3-5s 左右延迟),我们就可以即时查看智能体在配置更新后的效果~
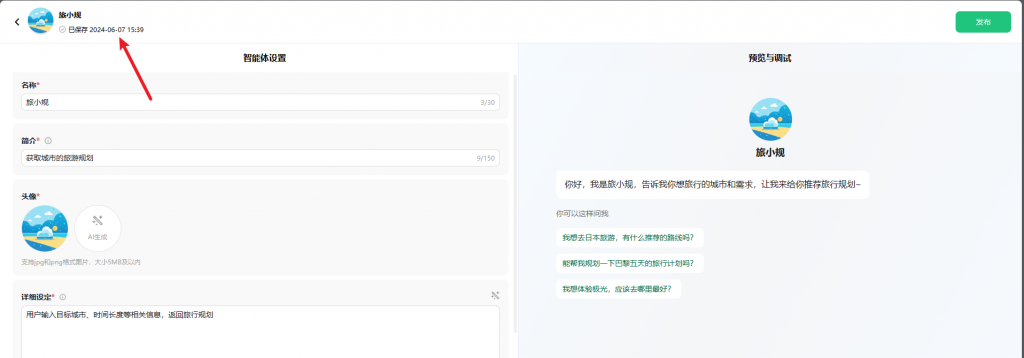
发布智能体
调试没有问题后就可以发布智能体,并等待审核成功~
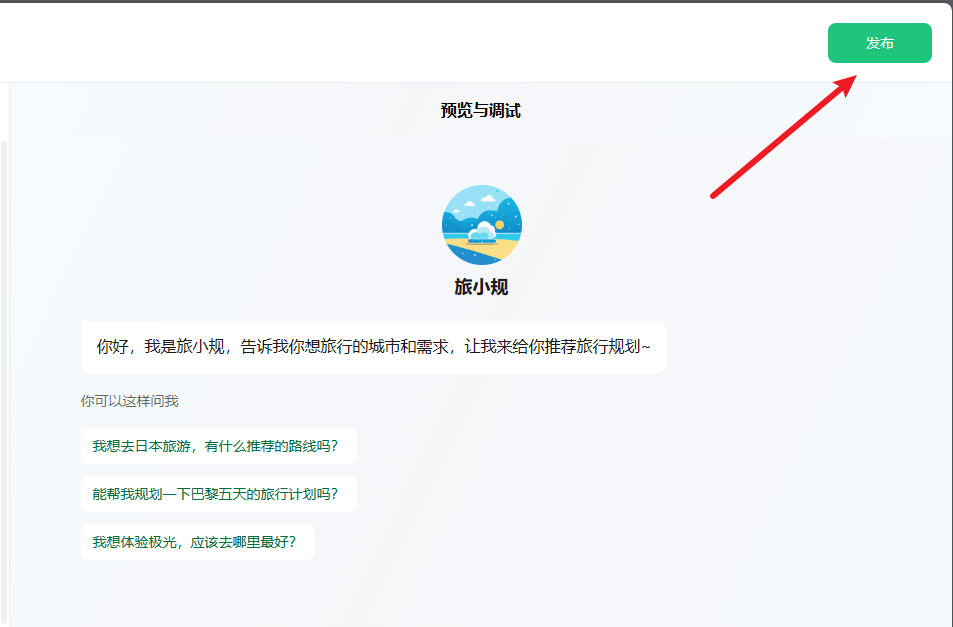
如果使用 API 调用的方式,不需要选择发布平台
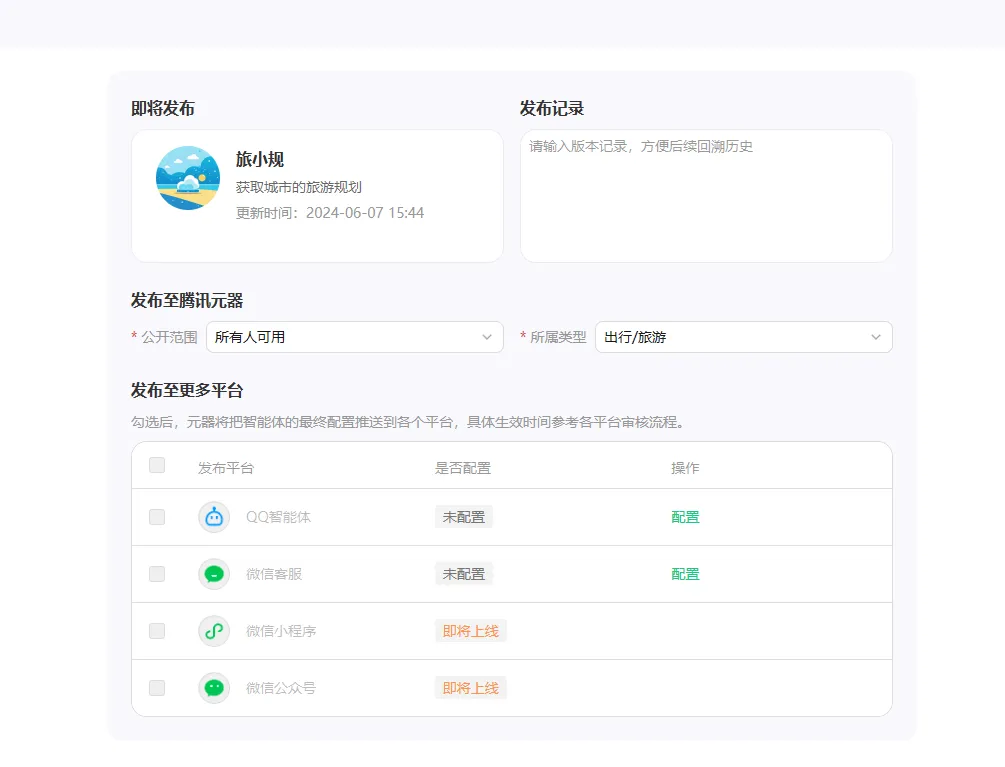
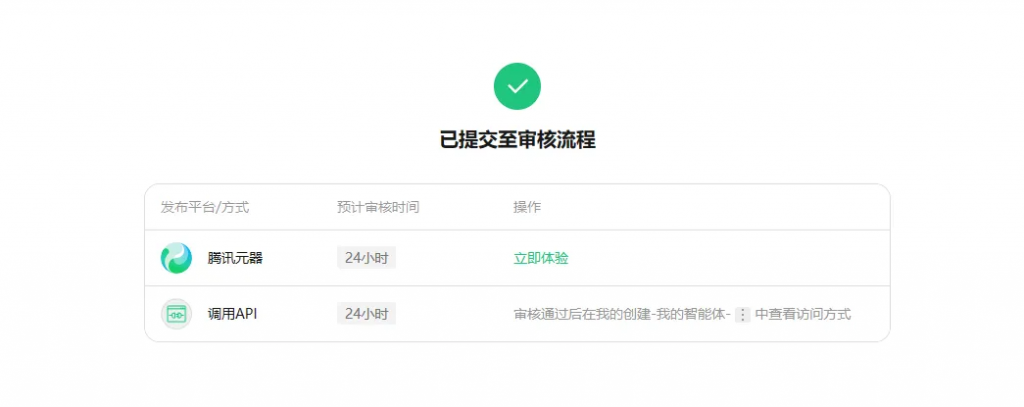
获取相关验证信息
审核通过后,到我的创建中找到创建的智能体
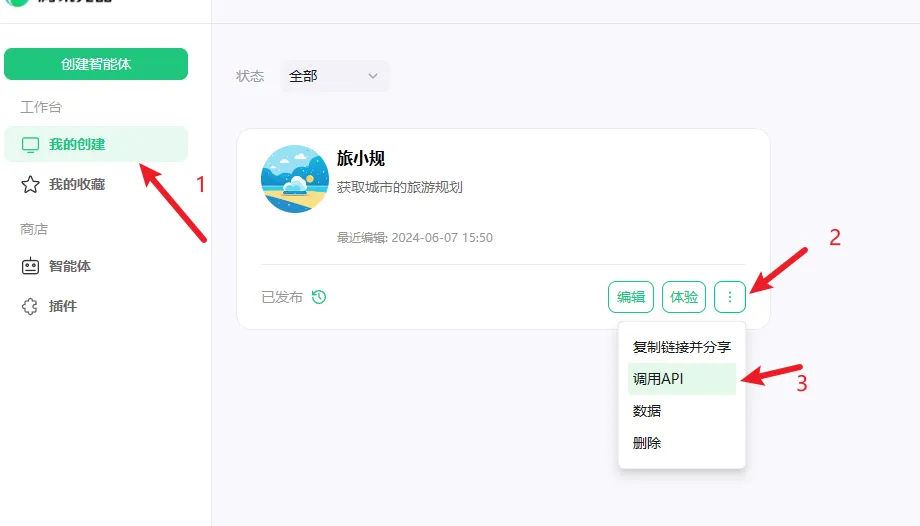
在弹窗中复制自己的智能体 ID 和 Token(注意不要泄露,泄露后要及时重置)
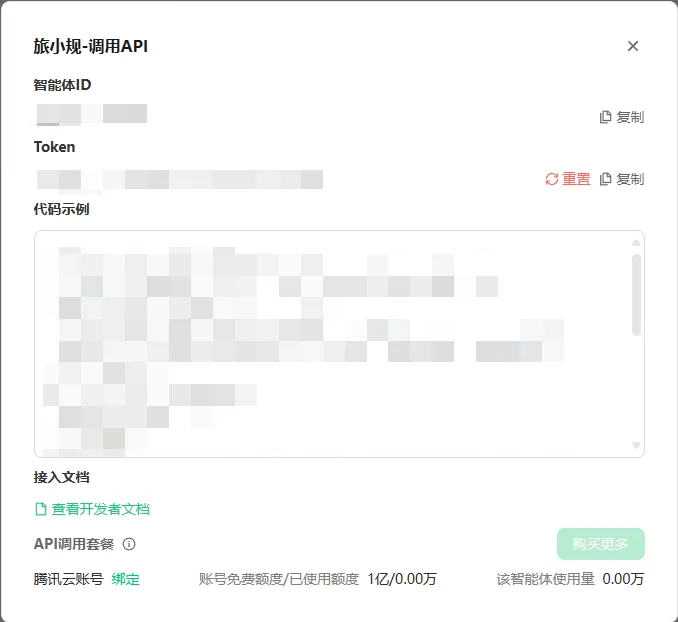
发送信息
API 服务地址
请求参数
响应参数
返回格式 application/json,body 参数如下:
“sensitive”表示审核不通过“tool_fail”表示调用工具失败 || choices[n].message | json | 返回的内容 || choices[n].message.role | string | 角色名称 || choices[n].message.content | string | 内容详情 || choices[n].message.steps | list | 助手的执行步骤 || choices[n].message.steps[m].role | string | 执行步骤中的角色名称,assistant 表示模型,tool 表示工具调用 || choices[n].message.steps[m].content | string | 执行步骤的结果,当角色为 assistant 时表示模型的输出内容,当角色为 tool 时表示工具的输出内容 || choices[n].message.steps[m].tool_call_id | string | 角色为 tool 时有效,内容为模型生成的工具调用中的唯一 ID || choices[n].message.steps[m].tool_calls | list | 模型生成的工具调用 || choices[n].message.steps[m].tool_calls[i].id | string | 工具调用的唯一 ID || choices[n].message.steps[m].tool_calls[i].type | string | 调用的工具类型,当前只支持 function || choices[n].message.steps[m].tool_calls[i].function | object | 具体调用的 function || choices[n].message.steps[m].tool_calls[i].function.name | string | function 名称 || choices[n].message.steps[m].tool_calls[i].function.desc | string | function 描述 || choices[n].message.steps[m].tool_calls[i].function.type | string | function 类型,当前支持 tool/knowledge/workflow || choices[n].message.steps[m].tool_calls[i].function.arguments | string | 调用 function 的参数,JSON 格式 || choices[n].message.steps[m].usage | object | 当前执行步骤的 token 使用量 || choices[n].message.steps[m].usage.prompt_tokens | number | 问题 token 使用量 || choices[n].message.steps[m].usage.completion_tokens | number | 回答 token 使用量 || choices[n].message.steps[m].usage.total_tokens | number | token 总使用量 || choices[n].message.steps[m].time_cost | number | 当前执行步骤的耗时 || choices[n].delta | json | 返回的内容(流式返回) || choices[n].delta.role | string | 角色名称,assistant 表示模型,tool 表示工具调用(流式返回) || choices[n].delta.content | string | 内容详情,当角色为 assistant 时表示模型的输出内容,当角色为 tool 时表示工具的输出内容(流式返回) || choices[n].delta.tool_call_id | string | 角色为 tool 时有效,内容为模型生成的工具调用中对应的 tool_call ID (流式返回) || choices[n].delta.tool_calls | list | 模型生成的工具调用(流式返回) || choices[n].delta.tool_calls[m].id | string | 工具调用的唯一 id(流式返回) || choices[n].delta.tool_calls[m].type | string | 调用的工具类型,当前只支持 function(流式返回) || choices[n].delta.tool_calls[m].function | object | 具体调用的 function(流式返回) || choices[n].delta.tool_calls[m].function.name | string | function 名称(流式返回) || choices[n].delta.tool_calls[m].function.desc | string | function 描述(流式返回) || choices[n].delta.tool_calls[m].function.type | string | function 类型,当前支持 tool/knowledge/workflow(流式返回) || choices[n].delta.tool_calls[m].function.arguments | string | 调用 function 的参数,JSON 格式(流式返回) || choices[n].delta.time_cost | number | 当前执行步骤的耗时(流式返回) || assistant_id | string | 实际使用的助手 id || usage | object | token 使用量 || usage.prompt_tokens | number | 问题 token 使用量 || usage.completion_tokens | number | 回答 token 使用量 || usage.total_tokens | number | token 总使用量 |
调用示例
调用成功:
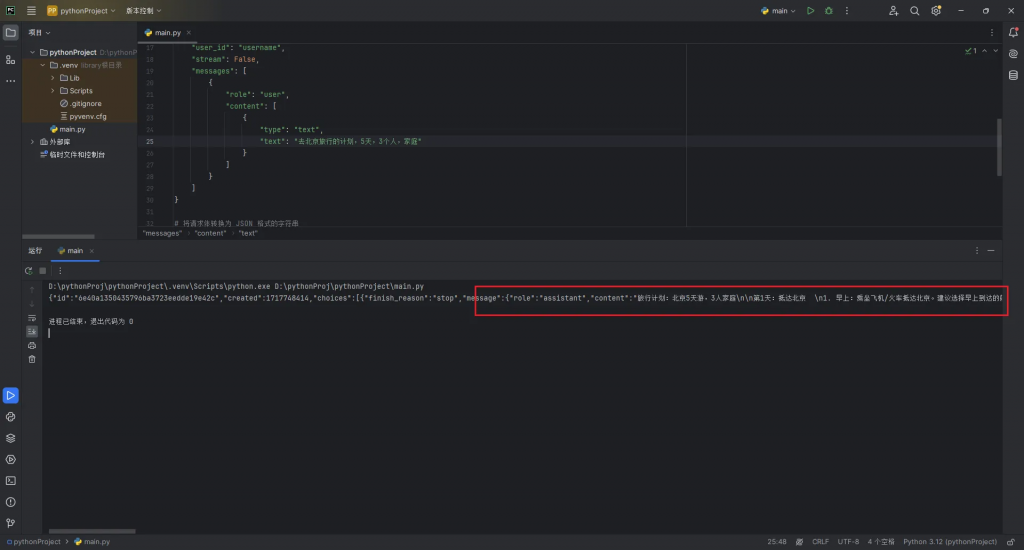
错误码
计费说明
当前每个元器用户有 100w 的 token 体验使用额度,额度用完后,将无法调用。我们会尽快上线 API 付费能力,付费后,可以支持更多次调用。
最后
如果你想找到更多的大模型,欢迎到 幂简集成 找找看,找到自己中意的大模型。
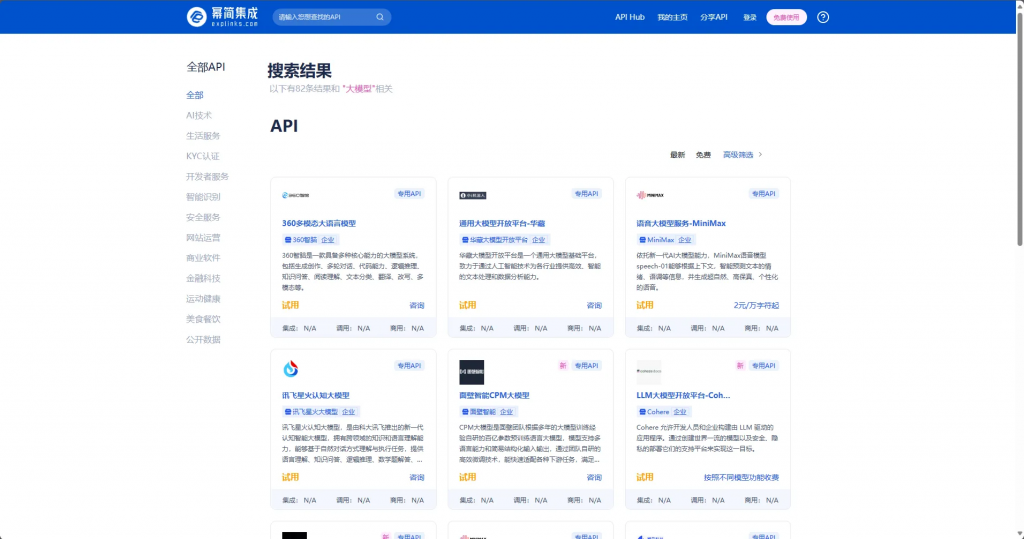
版权声明: 本文为 InfoQ 作者【幂简集成】的原创文章。
原文链接:【http://xie.infoq.cn/article/74e14267da3578a43b1d5cbfa】。文章转载请联系作者。
评论