【GreatSQL 优化器 -15】index merge
- 2025-02-21 福建
本文字数:21966 字
阅读完需:约 72 分钟
【GreatSQL 优化器-15】index merge
一、index merge 介绍
GreatSQL 的优化器的Index Merge Optimization
是查询优化器在处理复杂查询时使用的一种高级技术。当查询的 WHERE 子句中有多个独立的条件,且每个条件都可以使用不同的索引时,优化器会尝试将这些索引合并起来,以提高查询效率。这种优化策略允许数据库在一个查询中同时使用多个索引,从而避免全表扫描或减少需要扫描的数据量。
在某些情况下,单独使用任何一个索引都无法高效地获取到完整的结果集。而通过合并多个索引的扫描结果,我们可以更精确地定位到满足所有条件的记录,从而提高查询效率。当优化器生成 mm tree 的时候会保存不同索引的 tree 信息,生成 mm tree 之后会基于 OR 或者 AND 条件进行索引并集合并或者交集合并,从而实现 index merge。
下面用一个简单的例子来说明索引合并是什么。
greatsql> CREATE TABLE t1 (c1 INT PRIMARY KEY, c2 INT,date1 DATETIME);
greatsql> INSERT INTO t1 VALUES (1,10,'2021-03-25 16:44:00.123456'),(2,1,'2022-03-26 16:44:00.123456'),(3,4,'2023-03-27 16:44:00.123456'),(5,5,'2024-03-25 16:44:00.123456'),(7,null,'2020-03-25 16:44:00.123456'),(8,10,'2020-10-25 16:44:00.123456'),(11,16,'2023-03-25 16:44:00.123456');
greatsql> CREATE TABLE t2 (cc1 INT, cc2 INT,cc3 int);
greatsql> INSERT INTO t2 VALUES (1,3,1),(2,1,4),(3,2,10),(4,3,4),(5,15,10),(1,10,3),(4,4,1),(6,4,9),(11,110,1);
greatsql> CREATE INDEX idx1 ON t1(c2);
greatsql> CREATE INDEX idx2 ON t1(c2,date1);
greatsql> CREATE INDEX idx2_1 ON t2(cc1);
greatsql> CREATE INDEX idx2_2 ON t2(cc2);
greatsql> CREATE INDEX idx2_3 ON t2(cc3);
greatsql> explain SELECT * FROM t2 where cc2=3 and cc1=1 and cc3=1;
+----+-------------+-------+------------+-------------+----------------------+---------------+---------+------+------+----------+---------------------------------------------+
| id | select_type | table | partitions | type | possible_keys | key | key_len | ref | rows | filtered | Extra |
+----+-------------+-------+------------+-------------+----------------------+---------------+---------+------+------+----------+---------------------------------------------+
| 1 | SIMPLE | t2 | NULL | index_merge | idx2_1,idx2_2,idx2_3 | idx2_1,idx2_2 | 5,5 | NULL | 1 | 33.33 | Using intersect(idx2_1,idx2_2); Using where |
这里用到了索引交集合并
+----+-------------+-------+------------+-------------+----------------------+---------------+---------+------+------+----------+---------------------------------------------+
二、test_quick_select 代码解释
首先了解一下 ROR 的定义,ROR 的含义是 Rowid-Ordered Retrieval,表示单个索引返回的结果集是按照主键有序排列的。索引交集和并集是对 ROR 的索引进行操作,而如果有非 ROR 索引的话就要执行排序并集操作。
不是所有涉及不同 mm tree 的查询最后都会走索引合并,还是要取决于 cost 大小,有时候全表扫描反而 cost 更小。例子见下面第三节。
int test_quick_select() {
// 先判断skip_scan是否满足条件,不满足的话执行索引合并。skip_scan下一期介绍
AccessPath *skip_scan_path = get_best_skip_scan();
// 不满足skip_scan执行索引合并
if (tree && (best_path == nullptr || !get_forced_by_hint(best_path))) {
// 获取is_ror_scan值与table->quick_rows[keynr]区间范围行数,is_ror_scan为true才能执行索引合并,取值见表二
get_key_scans_params();
// 执行索引交集合并,计算cost并跟全表扫描对比,选取cost低的path
AccessPath *rori_path = get_best_ror_intersect(
thd, ¶m, table, index_merge_intersect_allowed, tree,
&needed_fields, best_cost,
/*force_index_merge_result=*/true, /*reuse_handler=*/true);
// tree->merges在tree_or()的时候赋值,赋值条件见表二
if (!tree->merges.is_empty()) {
// 按照不同索引组执行索引并集合并,计算cost并跟全表扫描对比,选取cost低的path
for (SEL_IMERGE &imerge : tree->merges) {
new_conj_path = get_best_disjunct_quick(
thd, ¶m, table, index_merge_union_allowed,
index_merge_sort_union_allowed, index_merge_intersect_allowed,
skip_records_in_range, &needed_fields, &imerge, best_cost,
needed_reg);
}
}
}
}
// 执行索引交集合并
AccessPath *get_best_ror_intersect() {
// 遍历mm tree的索引数组,给tree->ror_scans数组和cpk_scan赋值,cpk_scan专门存放主键索引信息
for (idx = 0, cur_ror_scan = tree->ror_scans; idx < param->keys; idx++) {
tree->ror_scans = make_ror_scan();
}
// 对tree->ror_scans数组根据覆盖列个数和范围包含的行数进行排序,范围包含的行数越少排序越前。这个步骤排除了主键索引,因为主键在函数最后单独处理
// 排序函数见下面is_better_intersect_match函数
find_intersect_order(tree->ror_scans, tree->ror_scans_end, param,
needed_fields);
// 按照上面的索引排序顺序进行交集操作,可以减少cost的索引进行交集操作,不能减少的排除
while (cur_ror_scan != tree->ror_scans_end && !intersect->is_covering) {
// 计算除了主键以外的所有索引的过滤系数、行数、cost并且累加到intersect变量
ror_intersect_add(intersect);
}
// 计算主键列的过滤系数、行数、cost并与之前别的索引结果累加到intersect变量
if (cpk_scan) ror_intersect_add(intersect);
// 最后生成AccessPath
AccessPath *path = new (param->return_mem_root) AccessPath;
}
// 对索引进行排序,可以看到覆盖的列越少,包含的行数越少,排序越靠前
static bool is_better_intersect_match(const ROR_SCAN_INFO *scan1,
const ROR_SCAN_INFO *scan2) {
if (scan1 == scan2) return false;
if (scan1->num_covered_fields_remaining > scan2->num_covered_fields_remaining)
return false;
if (scan1->num_covered_fields_remaining < scan2->num_covered_fields_remaining)
return true;
return (scan1->records > scan2->records);
}
// 执行索引并集合并
static AccessPath *get_best_disjunct_quick() {
// 按照索引遍历所有tree
for (auto tree_it = imerge->trees.begin(); tree_it != imerge->trees.end();
++tree_it, cur_child++) {
// 获取is_ror_scan值与table->quick_rows[keynr]区间范围行数,is_ror_scan为true才能执行索引合并,取值见表二
get_key_scans_params();
}
// 如果所有索引都是ROR的,那么直接返回结果
if (all_scans_rors && (index_merge_union_allowed || force_index_merge))
return get_ror_union_path();
// 如果不是所有索引都是ROR的,那么需要执行Sort-Union
// 首先计算磁盘扫描的cost
get_sweep_read_cost();
// 如果扫描磁盘cost太大,那么继续执行Sort-Union
// 索引去重cost估计
dup_removal_cost = Unique::get_use_cost(
(uint)non_cpk_scan_records, table->file->ref_length,
// 这个系统变量见表七
thd->variables.sortbuff_size, cost_model);
// 执行索引Sort-Union
get_ror_union_path();
}
static AccessPath *get_ror_union_path() {
// 遍历所有tree元素,对每个元素执行Intersection Merge
for (auto tree_it = imerge->trees.begin(); tree_it != imerge->trees.end();
tree_it++, cur_child++, cur_roru_plan++) {
get_best_ror_intersect();
}
// 计算磁盘扫描cost
get_sweep_read_cost();
// 生成AccessPath,这个AccessPath带有child即Intersection Merge的索引子集
AccessPath *path = new (param->return_mem_root) AccessPath;
path->rowid_union().children = children;
}
表一:索引合并类型
表二:is_ror_scan 取值情况
注:is_ror_scan 原则就是通过条件可以确定唯一的位置,这就是 ROR 有序的含义
表三:tree->merges 数组赋值条件
表四:不同索引合并方法行数计算方法
表五:跟索引合并相关的 OPTIMIZER_SWITCH
表六:索引扫描类型
表七:涉及的系统变量
三、实际例子说明
接下来看几个例子来说明上面的代码。
交集合并
greatsql> EXPLAIN SELECT * FROM t1 where c2=10 and c1<10;
+----+-------------+-------+------------+-------------+-------------------+--------------+---------+------+------+----------+--------------------------------------------+
| id | select_type | table | partitions | type | possible_keys | key | key_len | ref | rows | filtered | Extra |
+----+-------------+-------+------------+-------------+-------------------+--------------+---------+------+------+----------+--------------------------------------------+
| 1 | SIMPLE | t1 | NULL | index_merge | PRIMARY,idx1,idx2 | idx1,PRIMARY | 9,4 | NULL | 1 | 58.33 | Using intersect(idx1,PRIMARY); Using where |
+----+-------------+-------+------------+-------------+-------------------+--------------+---------+------+------+----------+--------------------------------------------+
"analyzing_range_alternatives": {
"range_scan_alternatives": [
{
"index": "PRIMARY",
"ranges": [
"c1 < 10"
],
"index_dives_for_eq_ranges": true,
"rowid_ordered": true, 这里为true说明这个索引是ROR的
"using_mrr": false,
"index_only": false,
"in_memory": 1,
"rows": 6,
"cost": 0.861465,
"chosen": true
},
{
"index": "idx1",
"ranges": [
"c2 = 10 AND c1 < 10"
],
"index_dives_for_eq_ranges": true,
"rowid_ordered": true, 这里为true说明这个索引是ROR的
"using_mrr": false,
"index_only": false,
"in_memory": 1,
"rows": 2,
"cost": 0.96,
"chosen": false,
"cause": "cost"
},
{
"index": "idx2",
"ranges": [
"c2 = 10"
],
"index_dives_for_eq_ranges": true,
"rowid_ordered": false, 这里为false说明这个索引不可以被合并
"using_mrr": false,
"index_only": true,
"in_memory": 0,
"rows": 2,
"cost": 1.21183,
"chosen": false,
"cause": "cost"
}
],
"analyzing_roworder_intersect": {
"intersecting_indexes": [ 上面"rowid_ordered"数量加起来为2,因此可以执行索引交集
{
"index": "idx1",
"index_scan_cost": 0.250274, 满足c2 = 10行数2条,这个是对应2条的cost
"cumulated_index_scan_cost": 0.250274,
"disk_sweep_cost": 0.4375, 从硬盘读取数据的cost
"cumulated_total_cost": 0.687774, 这个值=index_scan_cost+disk_sweep_cost
"usable": true,
"matching_rows_now": 2,
"isect_covering_with_this_index": false,
"chosen": true
}
],
"clustered_pk": {
"index_scan_cost": 0.1,
"cumulated_index_scan_cost": 0.350274, 这个值=index_scan_cost+上一个cumulated_index_scan_cost
"disk_sweep_cost": 0.25,
"clustered_pk_scan_added_to_intersect": true,
"cumulated_cost": 0.600274 这个值=disk_sweep_cost+cumulated_index_scan_cost,这里取两个索引交集以后的cost小于单独用idx1索引的cost 0.687774,说明索引交集确实提高了效率
},
"rows": 1.71429, 这个值=索引行数 * 过滤系数 =7 * 2/7 * 6/7,其中2是满足idx1条件c2=10的行数,6是满足主键列c1<10的行数
"cost": 0.600274,
"covering": false,
"chosen": true
}
},
"chosen_range_access_summary": {
"range_access_plan": {
"type": "index_roworder_intersect",
"rows": 1.71429,
"cost": 0.600274,
"covering": false,
"clustered_pk_scan": true,
"intersect_of": [
{
"type": "range_scan",
"index": "idx1",
"rows": 2,
"ranges": [
"c2 = 10 AND c1 < 10"
]
}
]
},
"rows_for_plan": 1.71429,
"cost_for_plan": 0.600274,
"chosen": true
}
}
}
{
"considered_execution_plans": [
{
"plan_prefix": [
],
"table": "`t1`",
"best_access_path": {
"considered_access_paths": [
{
"access_type": "ref",
"index": "idx1",
"chosen": false,
"cause": "range_uses_more_keyparts"
},
{
"access_type": "ref", 这个是因为keyuse_array数组有值,所以根据索引单独计算
"index": "idx2",
"rows": 2,
"cost": 1.20183,
"chosen": true
},
{
"rows_to_scan": 1, 这个值等于上面索引交集算出来的"rows_for_plan": 1.71429
"filtering_effect": [
],
"final_filtering_effect": 1,
"access_type": "range",
"range_details": {
"used_index": "intersect(idx1,PRIMARY)"
},
"resulting_rows": 1, 这个值等于"rows_to_scan"
"cost": 0.700274,
"chosen": true
}
]
},
"condition_filtering_pct": 100,
"rows_for_plan": 1,
"cost_for_plan": 0.700274, 最后结果:在上面ref和range两种方法中选择了cost低的range方法即索引交集方法
"chosen": true
}
]
},
并集合并
greatsql> CREATE TABLE t4 (d1 INT, d2 int, d3 varchar(100));
greatsql> INSERT INTO t4 VALUES (1,2,'aa1'),(2,1,'bb1'),(2,3,'cc1'),(3,3,'cc1'),(4,2,'ff1'),(4,4,'ert'),(4,2,'f5fg'),(null,2,'ee'),(5,30,'cc1'),(5,4,'fcc1'),(4,10,'cc1'),(6,4,'ccd1'),(null,1,'fee'),(1,2,'aa1'),(2,1,'bb1'),(2,3,'cc1'),(3,3,'cc1'),(4,2,'ff1'),(4,4,'ert'),(4,2,'f5fg'),(null,2,'ee'),(5,30,'cc1'),(5,4,'fcc1'),(4,10,'cc1'),(6,4,'ccd1'),(null,1,'fee'),(1,2,'aa1'),(2,1,'bb1'),(2,3,'cc1'),(3,3,'cc1'),(4,2,'ff1'),(4,4,'ert'),(4,2,'f5fg'),(null,2,'ee'),(5,30,'cc1'),(5,4,'fcc1'),(4,10,'cc1'),(6,4,'ccd1'),(null,1,'fee');
greatsql> CREATE INDEX idx4_1 ON t4(d1);
greatsql> CREATE INDEX idx4_2 ON t4(d2);
greatsql> EXPLAIN SELECT * FROM t4 where d2=4 or d1=10;
+----+-------------+-------+------------+-------------+---------------+---------------+---------+------+------+----------+-----------------------------------------+
| id | select_type | table | partitions | type | possible_keys | key | key_len | ref | rows | filtered | Extra |
+----+-------------+-------+------------+-------------+---------------+---------------+---------+------+------+----------+-----------------------------------------+
| 1 | SIMPLE | t4 | NULL | index_merge | idx4_2,idx4_1 | idx4_2,idx4_1 | 5,5 | NULL | 10 | 100.00 | Using union(idx4_2,idx4_1); Using where |
+----+-------------+-------+------------+-------------+---------------+---------------+---------+------+------+----------+-----------------------------------------+
"analyzing_range_alternatives": {
"range_scan_alternatives": [
],
"analyzing_roworder_intersect": {
"usable": false,
"cause": "too_few_roworder_scans"
}
},
"analyzing_index_merge_union": [
{
"indexes_to_merge": [
{
"range_scan_alternatives": [
{
"index": "idx4_2",
"ranges": [
"d2 = 4"
],
"index_dives_for_eq_ranges": true,
"rowid_ordered": true, 这里为true说明这个索引是ROR的
"using_mrr": false,
"index_only": true,
"in_memory": 0,
"rows": 9,
"cost": 1.92074,
"chosen": true
}
],
"index_to_merge": "idx4_2",
"cumulated_cost": 1.92074
},
{
"range_scan_alternatives": [
{
"index": "idx4_1",
"ranges": [
"d1 = 10"
],
"index_dives_for_eq_ranges": true,
"rowid_ordered": true, 这里为true说明这个索引是ROR的
"using_mrr": false,
"index_only": true,
"in_memory": 0,
"rows": 1,
"cost": 1.11,
"chosen": true
}
],
"index_to_merge": "idx4_1",
"cumulated_cost": 3.03074 这个值=idx4_1索引对应的cost+idx4_2索引对应的cost=1.11+1.92074
}
],
"cost_of_reading_ranges": 3.03074,
"use_roworder_union": true,
"cause": "always_cheaper_than_not_roworder_retrieval",
"analyzing_roworder_scans": [
{
"type": "range_scan",
"index": "idx4_2",
"rows": 9,
"ranges": [
"d2 = 4"
],
"analyzing_roworder_intersect": {
"usable": false,
"cause": "too_few_roworder_scans"
}
},
{
"type": "range_scan",
"index": "idx4_1",
"rows": 1,
"ranges": [
"d1 = 10"
],
"analyzing_roworder_intersect": {
"usable": false,
"cause": "too_few_roworder_scans"
}
}
],
"index_roworder_union_cost": 4.47442,
"members": 2, 涉及2个索引
"chosen": true
}
],
"chosen_range_access_summary": {
"range_access_plan": {
"type": "index_roworder_union",
"union_of": [
{
"type": "range_scan",
"index": "idx4_2",
"rows": 9,
"ranges": [
"d2 = 4"
]
},
{
"type": "range_scan",
"index": "idx4_1",
"rows": 1,
"ranges": [
"d1 = 10"
]
}
]
},
"rows_for_plan": 10,
"cost_for_plan": 4.47442,
"chosen": true
}
}
}
]
},
排序合并
greatsql> EXPLAIN SELECT * FROM t4 WHERE d1 < 2 OR d2 > 20;
+----+-------------+-------+------------+-------------+---------------+---------------+---------+------+------+----------+----------------------------------------------+
| id | select_type | table | partitions | type | possible_keys | key | key_len | ref | rows | filtered | Extra |
+----+-------------+-------+------------+-------------+---------------+---------------+---------+------+------+----------+----------------------------------------------+
| 1 | SIMPLE | t4 | NULL | index_merge | idx4_2,idx4_1 | idx4_1,idx4_2 | 5,5 | NULL | 6 | 100.00 | Using sort_union(idx4_1,idx4_2); Using where |
+----+-------------+-------+------------+-------------+---------------+---------------+---------+------+------+----------+----------------------------------------------+
"analyzing_range_alternatives": {
"range_scan_alternatives": [
],
"analyzing_roworder_intersect": {
"usable": false,
"cause": "too_few_roworder_scans"
}
},
"analyzing_index_merge_union": [
{
"indexes_to_merge": [
{
"range_scan_alternatives": [
{
"index": "idx4_1",
"ranges": [
"NULL < d1 < 2"
],
"index_dives_for_eq_ranges": true,
"rowid_ordered": false, 这里说明这个不是ROR索引,因为条件是一个范围不是等于
"using_mrr": false,
"index_only": true,
"in_memory": 1,
"rows": 3,
"cost": 0.560671,
"chosen": true
}
],
"index_to_merge": "idx4_1",
"cumulated_cost": 0.560671
},
{
"range_scan_alternatives": [
{
"index": "idx4_2",
"ranges": [
"20 < d2"
],
"index_dives_for_eq_ranges": true,
"rowid_ordered": false, 这里说明这个不是ROR索引,因为条件是一个范围不是等于
"using_mrr": false,
"index_only": true,
"in_memory": 1,
"rows": 3,
"cost": 0.560671,
"chosen": true
}
],
"index_to_merge": "idx4_2",
"cumulated_cost": 1.12134 这个值=两个索引累加=0.560671+0.560671
}
],
"cost_of_reading_ranges": 1.12134,
"cost_sort_rowid_and_read_disk": 0.822021, 磁盘扫描的cost
"cost_duplicate_removal": 1.2282, 索引排序去重cost
"total_cost": 3.17157
}
],
"chosen_range_access_summary": {
"range_access_plan": {
"type": "index_merge",
"index_merge_of": [
{
"type": "range_scan",
"index": "idx4_1",
"rows": 3,
"ranges": [
"NULL < d1 < 2"
]
},
{
"type": "range_scan",
"index": "idx4_2",
"rows": 3,
"ranges": [
"20 < d2"
]
}
]
},
"rows_for_plan": 6,
"cost_for_plan": 3.17157,
"chosen": true
}
}
}
]
},
上面的例子改一个条件范围,结果变成不选择排序合并而选择全表扫描的情况。下面 trace 对比上面发现,少了"chosen_range_access_summary"
,对比源码发现是imerge_cost > read_cost
,因此被放弃。这里主要原因是索引排序 cost 太高所以放弃了。
greatsql> EXPLAIN SELECT * FROM t4 WHERE d1 < 4 OR d2 > 20;
+----+-------------+-------+------------+------+---------------+------+---------+------+------+----------+-------------+
| id | select_type | table | partitions | type | possible_keys | key | key_len | ref | rows | filtered | Extra |
+----+-------------+-------+------------+------+---------------+------+---------+------+------+----------+-------------+
| 1 | SIMPLE | t4 | NULL | ALL | idx4_2,idx4_1 | NULL | NULL | NULL | 39 | 53.84 | Using where |
+----+-------------+-------+------------+------+---------------+------+---------+------+------+----------+-------------+
{
"rows_estimation": [
{
"table": "`t4`",
"range_analysis": {
"table_scan": {
"rows": 39,
"cost": 6.25 这个是全表扫描的cost
},
"analyzing_index_merge_union": [
{
"indexes_to_merge": [
{
"range_scan_alternatives": [
{
"index": "idx4_1",
"ranges": [
"NULL < d1 < 4"
],
"index_dives_for_eq_ranges": true,
"rowid_ordered": false,
"using_mrr": false,
"index_only": true,
"in_memory": 1,
"rows": 12,
"cost": 1.46369,
"chosen": true
}
],
"index_to_merge": "idx4_1",
"cumulated_cost": 1.46369
},
{
"range_scan_alternatives": [
{
"index": "idx4_2",
"ranges": [
"20 < d2"
],
"index_dives_for_eq_ranges": true,
"rowid_ordered": false,
"using_mrr": false,
"index_only": true,
"in_memory": 1,
"rows": 3,
"cost": 0.560671,
"chosen": true
}
],
"index_to_merge": "idx4_2",
"cumulated_cost": 2.02436
}
],
"cost_of_reading_ranges": 2.02436,
"cost_sort_rowid_and_read_disk": 0.986637,
"cost_duplicate_removal": 4.42426,
"total_cost": 7.43526 索引合并cost大于全表扫描cost的6.25,因此不选择用索引合并
}
]
}
}
]
},
无法使用index merge
交集合并的情况
列的第二个以上条件有右节点
greatsql> EXPLAIN SELECT * FROM t4 WHERE d2>=2 AND (d2>=6 or d2=4);
+----+-------------+-------+------------+-------+---------------+--------+---------+------+------+----------+-----------------------+
| id | select_type | table | partitions | type | possible_keys | key | key_len | ref | rows | filtered | Extra |
+----+-------------+-------+------------+-------+---------------+--------+---------+------+------+----------+-----------------------+
| 1 | SIMPLE | t4 | NULL | range | idx4_2 | idx4_2 | 5 | NULL | 15 | 100.00 | Using index condition |
+----+-------------+-------+------------+-------+---------------+--------+---------+------+------+----------+-----------------------+
tree叶节点范围最大值和最小值不相等
greatsql> EXPLAIN SELECT * FROM t1 WHERE c1>=2 AND c2>=2 AND c2<=11;
+----+-------------+-------+------------+-------+-------------------+------+---------+------+------+----------+--------------------------+
| id | select_type | table | partitions | type | possible_keys | key | key_len | ref | rows | filtered | Extra |
+----+-------------+-------+------------+-------+-------------------+------+---------+------+------+----------+--------------------------+
| 1 | SIMPLE | t1 | NULL | range | PRIMARY,idx1,idx2 | idx2 | 5 | NULL | 4 | 85.71 | Using where; Using index |
+----+-------------+-------+------------+-------+-------------------+------+---------+------+------+----------+--------------------------+
多个索引与索引交集的选择
greatsql> CREATE TABLE t5 (d1 INT, d2 int, d3 int, d4 int, d5 int);
greatsql> INSERT INTO t5 VALUES (1,2,1,2,4),(2,1,1,2,1),(2,3,6,9,10),(3,3,2,1,5),(4,2,9,22,4),(4,4,2,1,3),(4,2,7,3,5);
greatsql> create index idx4_1 on t5(d1);
greatsql> CREATE INDEX idx4_2 ON t5(d2);
greatsql> CREATE INDEX idx4_3 ON t5(d3);
greatsql> CREATE INDEX idx4_4 ON t5(d4);
greatsql> CREATE INDEX idx4_5 ON t5(d5);
greatsql> EXPLAIN SELECT * FROM t5 where d2=2 and d4=2 and d3=1 ;
+----+-------------+-------+------------+-------------+----------------------+---------------+---------+------+------+----------+---------------------------------------------+
| id | select_type | table | partitions | type | possible_keys | key | key_len | ref | rows | filtered | Extra |
+----+-------------+-------+------------+-------------+----------------------+---------------+---------+------+------+----------+---------------------------------------------+
| 1 | SIMPLE | t5 | NULL | index_merge | idx4_2,idx4_3,idx4_4 | idx4_3,idx4_4 | 5,5 | NULL | 1 | 42.86 | Using intersect(idx4_3,idx4_4); Using where |
+----+-------------+-------+------------+-------------+----------------------+---------------+---------+------+------+----------+---------------------------------------------+
这个sql通过find_intersect_order函数排序结果为idx4_3、idx4_4、idx4_2,因为idx4_3和idx4_4的rows都是2,但是因为idx4_3在sql位置靠前因此排序靠前。而idx4_2包含的rows是3,因此位置在最后。
"analyzing_range_alternatives": {
"range_scan_alternatives": [
{
"index": "idx4_2",
"ranges": [
"d2 = 2"
],
"index_dives_for_eq_ranges": true,
"rowid_ordered": true,
"using_mrr": false,
"index_only": false,
"in_memory": 1,
"rows": 3,
"cost": 1.31,
"chosen": true
},
{
"index": "idx4_3",
"ranges": [
"d3 = 1"
],
"index_dives_for_eq_ranges": true,
"rowid_ordered": true,
"using_mrr": false,
"index_only": false,
"in_memory": 1,
"rows": 2,
"cost": 0.96,
"chosen": true
},
{
"index": "idx4_4",
"ranges": [
"d4 = 2"
],
"index_dives_for_eq_ranges": true,
"rowid_ordered": true,
"using_mrr": false,
"index_only": false,
"in_memory": 1,
"rows": 2,
"cost": 0.96,
"chosen": false,
"cause": "cost"
}
],
"analyzing_roworder_intersect": {
"intersecting_indexes": [
{
"index": "idx4_3",
"index_scan_cost": 0.250336,
"cumulated_index_scan_cost": 0.250336,
"disk_sweep_cost": 0.4375,
"cumulated_total_cost": 0.687836,
"usable": true,
"matching_rows_now": 2,
"isect_covering_with_this_index": false,
"chosen": true
},
{
"index": "idx4_4",
"index_scan_cost": 0.250336,
"cumulated_index_scan_cost": 0.500671,
"disk_sweep_cost": 0,
"cumulated_total_cost": 0.500671,
"usable": true,
"matching_rows_now": 0.571429,
"isect_covering_with_this_index": false,
"chosen": true 合并完idx4_3和idx4_4以后发现cost变小,因此可以选择
},
{
"index": "idx4_2",
"index_scan_cost": 0.250671,
"cumulated_index_scan_cost": 0.751342,
"disk_sweep_cost": 0,
"cumulated_total_cost": 0.751342,
"usable": true,
"matching_rows_now": 0.244898,
"isect_covering_with_this_index": false,
"chosen": false,
"cause": "does_not_reduce_cost" 这里合并到idx4_2就发现cost大于单独用前两个索引,因此不继续进行交集操作了
}
],
"clustered_pk": {
"clustered_pk_added_to_intersect": false,
"cause": "no_clustered_pk_index"
},
"rows": 1,
"cost": 0.500671,
"covering": false,
"chosen": true
}
索引并集合并的子集是索引交集的情况
greatsql> EXPLAIN SELECT * FROM t5 WHERE (d2=4 AND d1=4 ) OR (d3=1 AND d4=2);
+----+-------------+-------+------------+-------------+-----------------------------+----------------------+---------+------+------+----------+-----------------------------------------------------------+
| id | select_type | table | partitions | type | possible_keys | key | key_len | ref | rows | filtered | Extra |
+----+-------------+-------+------------+-------------+-----------------------------+----------------------+---------+------+------+----------+-----------------------------------------------------------+
| 1 | SIMPLE | t5 | NULL | index_merge | idx4_1,idx4_2,idx4_3,idx4_4 | idx4_2,idx4_3,idx4_4 | 5,5,5 | NULL | 2 | 100.00 | Using union(idx4_2,intersect(idx4_3,idx4_4)); Using where |
这里的子集没用idx4_1和idx4_2的交集,因为加上idx4_1以后的cost比单独用idx4_2还要大,因此idx4_1和idx4_2不使用索引交集
+----+-------------+-------+------------+-------------+-----------------------------+----------------------+---------+------+------+----------+-----------------------------------------------------------+
OPTIMIZER_SWITCH_FAVOR_RANGE_SCAN
使用举例
greatsql> EXPLAIN SELECT * FROM t5 WHERE d2=2 AND d4=2 AND d3=1;
+----+-------------+-------+------------+-------------+----------------------+---------------+---------+------+------+----------+---------------------------------------------+
| id | select_type | table | partitions | type | possible_keys | key | key_len | ref | rows | filtered | Extra |
+----+-------------+-------+------------+-------------+----------------------+---------------+---------+------+------+----------+---------------------------------------------+
| 1 | SIMPLE | t5 | NULL | index_merge | idx4_2,idx4_3,idx4_4 | idx4_3,idx4_4 | 5,5 | NULL | 1 | 42.86 | Using intersect(idx4_3,idx4_4); Using where |
+----+-------------+-------+------------+-------------+----------------------+---------------+---------+------+------+----------+---------------------------------------------+
加上FAVOR_RANGE_SCAN以后看到结果变为走单个索引而不走索引合并了。
greatsql> EXPLAIN SELECT /*+ set_var(optimizer_switch='FAVOR_RANGE_SCAN=ON') */ * FROM t5 WHERE d2=2 AND d4=2 AND d3=1;
+----+-------------+-------+------------+------+----------------------+--------+---------+-------+------+----------+-------------+
| id | select_type | table | partitions | type | possible_keys | key | key_len | ref | rows | filtered | Extra |
+----+-------------+-------+------------+------+----------------------+--------+---------+-------+------+----------+-------------+
| 1 | SIMPLE | t5 | NULL | ref | idx4_2,idx4_3,idx4_4 | idx4_3 | 5 | const | 2 | 14.29 | Using where |
+----+-------------+-------+------------+------+----------------------+--------+---------+-------+------+----------+-------------+
下面的trace可以看到每个单独的索引的cost都被改小十倍,这样后面执行索引合并的时候对比这个cost就会偏大,导致最后走单个索引而不是索引合并。
"analyzing_range_alternatives": {
"range_scan_alternatives": [
{
"index": "idx4_2",
"ranges": [
"d2 = 2"
],
"index_dives_for_eq_ranges": true,
"rowid_ordered": true,
"using_mrr": false,
"index_only": false,
"in_memory": 1,
"rows": 3,
"cost": 1.31,
"revised_cost": 0.131, 这个地方修改了cost值,变为cost * 0.1 = 1.31 * 0.1
"chosen": true
},
{
"index": "idx4_3",
"ranges": [
"d3 = 1"
],
"index_dives_for_eq_ranges": true,
"rowid_ordered": true,
"using_mrr": false,
"index_only": false,
"in_memory": 1,
"rows": 2,
"cost": 0.96,
"revised_cost": 0.096, 这个地方修改了cost值,变为cost * 0.1 = 1.31 * 0.1
"chosen": true
},
{
"index": "idx4_4",
"ranges": [
"d4 = 2"
],
"index_dives_for_eq_ranges": true,
"rowid_ordered": true,
"using_mrr": false,
"index_only": false,
"in_memory": 1,
"rows": 2,
"cost": 0.96,
"revised_cost": 0.096, 这个地方修改了cost值,变为cost * 0.1 = 1.31 * 0.1
"chosen": false,
"cause": "cost"
}
],
"analyzing_roworder_intersect": {
"intersecting_indexes": [
{
"index": "idx4_3",
"index_scan_cost": 0.250336,
"cumulated_index_scan_cost": 0.250336,
"disk_sweep_cost": 0.4375,
"cumulated_total_cost": 0.687836,
"usable": true,
"matching_rows_now": 2,
"isect_covering_with_this_index": false,
"chosen": true
},
{
"index": "idx4_4",
"index_scan_cost": 0.250336,
"cumulated_index_scan_cost": 0.500671,
"disk_sweep_cost": 0,
"cumulated_total_cost": 0.500671,
"usable": true,
"matching_rows_now": 0.571429,
"isect_covering_with_this_index": false,
"chosen": true
},
{
"index": "idx4_2",
"index_scan_cost": 0.250671,
"cumulated_index_scan_cost": 0.751342,
"disk_sweep_cost": 0,
"cumulated_total_cost": 0.751342,
"usable": true,
"matching_rows_now": 0.244898,
"isect_covering_with_this_index": false,
"chosen": false,
"cause": "does_not_reduce_cost"
}
],
"clustered_pk": {
"clustered_pk_added_to_intersect": false,
"cause": "no_clustered_pk_index"
},
"chosen": false, 索引合并cost大于idx4_3,因此没有选择索引合并
"cause": "cost"
}
},
"chosen_range_access_summary": { 最后结果选了cost最小的idx4_3,因为cost最小
"range_access_plan": {
"type": "range_scan",
"index": "idx4_3",
"rows": 2,
"ranges": [
"d3 = 1"
]
},
"rows_for_plan": 2,
"cost_for_plan": 0.096,
"chosen": true
}
}
}
四、总结
从上面索引合并的应用例子我们认识了索引合并的使用场合和好处,也知道了索引合并的三种类型以及分别使用的条件,认识了 ROR 条件的判定和使用,还有相关系统变量,以上选择都是系统自动选择的,如果要改变结果只能添加OPTIMIZER_SWITCH
强制改变索引的使用。
版权声明: 本文为 InfoQ 作者【GreatSQL】的原创文章。
原文链接:【http://xie.infoq.cn/article/b95350b282a73cc98f3316756】。文章转载请联系作者。
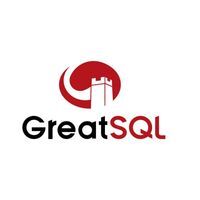
GreatSQL
GreatSQL社区 2023-01-31 加入
GreatSQL是由万里数据库维护的MySQL分支,专注于提升MGR可靠性及性能,支持InnoDB并行查询特性,是适用于金融级应用的MySQL分支版本。 社区:https://greatsql.cn/ Gitee: https://gitee.com/GreatSQL/GreatSQL
评论