Pytest 结合数据驱动的用法详解
全面解析软件测试开发:人工智能测试、自动化测试、性能测试、测试左移、测试右移到DevOps如何驱动持续交付
在软件测试中,数据驱动测试是一种重要的方法,可以通过多组测试数据验证功能的正确性,提高测试覆盖率,同时减少冗余的代码。Pytest 是一个灵活而强大的测试框架,其内置支持数据驱动测试功能,使得实现和管理数据驱动测试变得轻松高效。
本文将从 数据驱动测试的基本概念 入手,结合 Pytest 的实际应用,全面讲解如何在测试中使用数据驱动的方法。
一、什么是数据驱动测试?
数据驱动测试(Data-Driven Testing,DDT)是一种通过参数化实现的测试方法。它的核心思想是将测试逻辑与测试数据分离。测试逻辑只需实现一次,通过多组不同的数据进行重复执行,从而验证代码在不同场景下的表现。
数据驱动的优势
代码复用性高:一个测试函数可覆盖多组测试数据。
可维护性强:测试逻辑和测试数据分离,数据的更新不会影响测试逻辑。
易扩展:新增测试场景时,只需添加测试数据,无需修改测试逻辑。
二、Pytest 中的数据驱动方法
1. 使用 @pytest.mark.parametrize
Pytest 提供了 @pytest.mark.parametrize
装饰器,允许测试函数以不同的数据集运行。
单参数数据驱动
在这里,测试函数 test_is_even
会分别以参数 1, 2, 3, 4
运行,每次验证参数是否是偶数。
多参数数据驱动
可以为测试函数传入多组参数:
每一组
(a, b, expected)
是一个测试用例。Pytest 会自动为每一组数据执行一次测试。
2. 数据驱动结合外部数据源
在实际项目中,测试数据通常来自外部文件,例如 JSON、CSV 或 Excel,这些数据可以通过 Pytest 参数化传入测试函数。
从 JSON 文件读取数据
文件内容示例 (test_data.json
):
从 CSV 文件读取数据
文件内容示例 (test_data.csv
):
从 Excel 文件读取数据
使用 openpyxl
库读取 Excel 数据:
文件内容示例 (
test_data.xlsx
): | a | b | expected | | --- | --- | -------- | | 1 | 2 | 3 | | 2 | 3 | 5 | | 3 | 5 | 8 |
3. 数据驱动与 Fixture 的结合
Fixture 是 Pytest 中的一大亮点,用于测试环境的初始化和清理。Fixture 可以与数据驱动结合,动态生成测试数据。
4. 结合数据驱动与条件跳过
在某些情况下,可以结合数据驱动和条件跳过测试,例如,跳过不适用的数据集:
三、运行与结果分析
运行所有测试用例:
运行特定标记的数据驱动测试:
四、数据驱动测试的最佳实践
测试数据与逻辑分离:
使用外部文件管理测试数据(JSON、CSV、Excel)。
数据更新无需修改测试代码。
数据覆盖面广:
针对不同场景设计全面的测试数据集,覆盖正常情况、异常情况和边界情况。
使用 Fixture 动态生成数据:
通过计算或外部接口生成实时测试数据。
与 CI/CD 集成:
确保数据驱动测试在持续集成流程中稳定运行。
日志与报告生成:
使用
pytest-html
等插件生成测试报告,记录每组数据的测试结果。
Pytest 强大的参数化和扩展功能使得数据驱动测试的实现简单而高效。通过合理组织数据源、灵活使用参数化功能,可以显著提高测试的覆盖率和效率,为项目的高质量交付保驾护航。
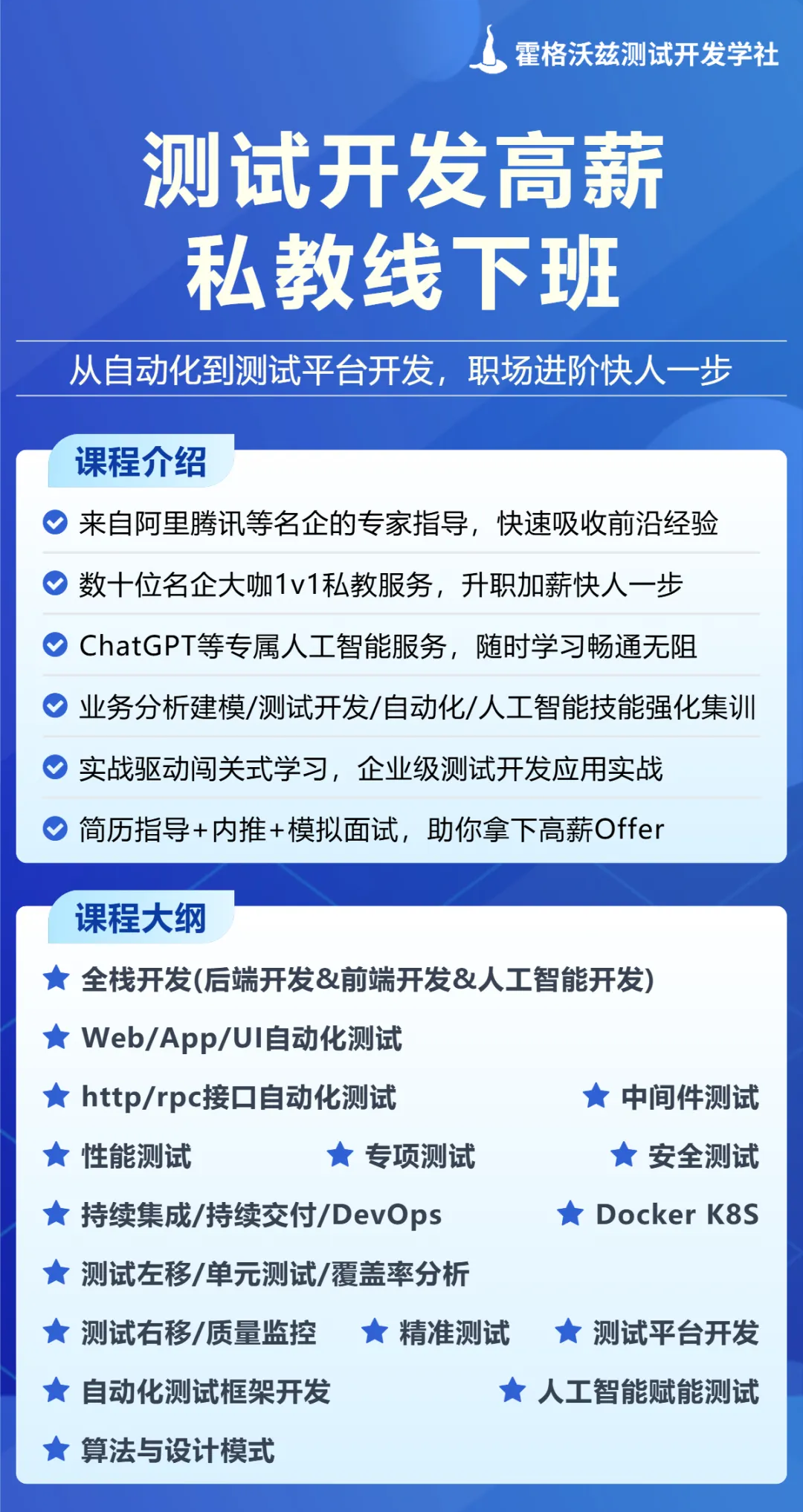
评论