6 月 14 日红杉最新报告:The New Language Model Stack
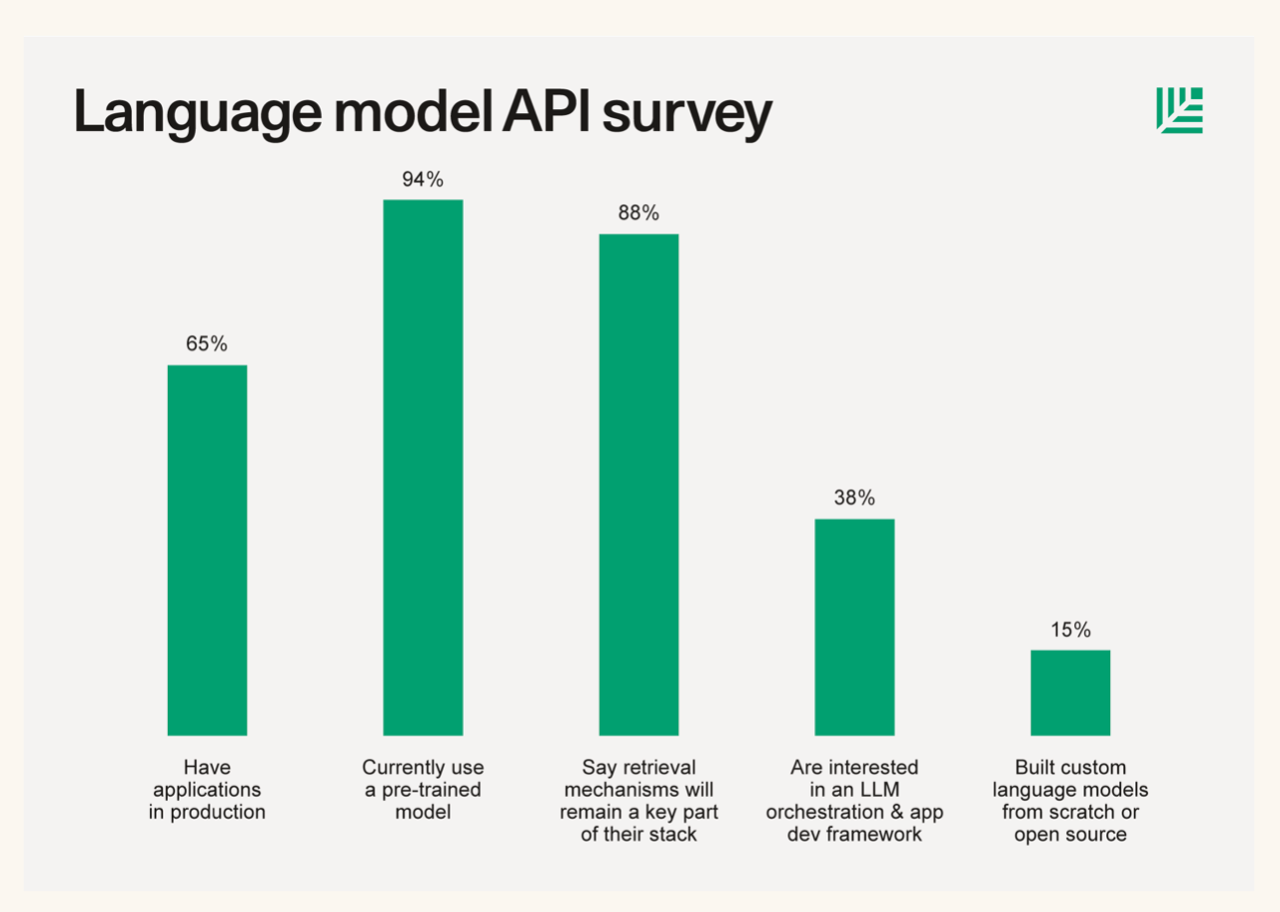
本文为知识星球内容推荐:
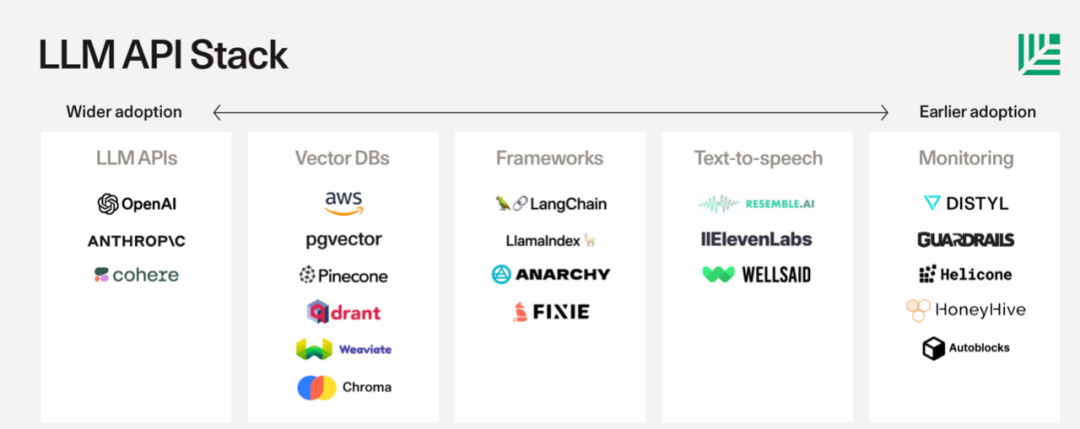
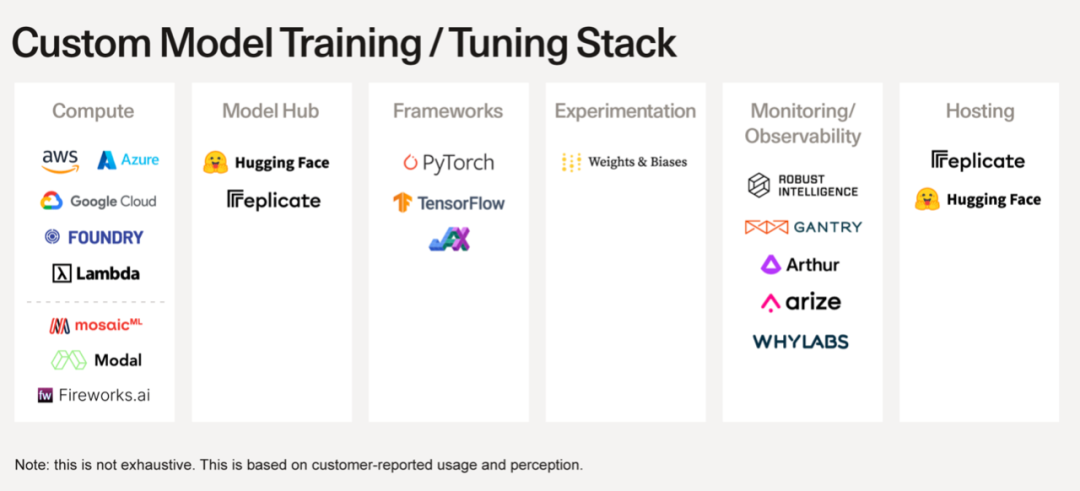
BloombergGPT 是在大型技术公司之外的自定义模型努力的一个很好的例子,它使用了 Hugging Face 和其他开源工具。随着开源工具的改进和越来越多的公司使用 LLM,我们预计会看到更多的自定义和预训练模型的使用。
语言模型需要变得更加可靠(输出质量、数据隐私、安全)才能得到全面采用。
从 Arc 到 Zoom,创始人都专注于同一件事情:用 AI 让用户感到愉悦。
ChatGPT 通过大型语言模型(LLMs)掀起了一股创新浪潮,比以往任何时候都有更多的公司将自然语言交互的能力带入其产品中。语言模型 API 的采用正在其后形成新的技术堆栈。为了更好地了解人们正在构建的应用程序和他们使用的堆栈,我们与 Sequoia 网络中的 33 家公司进行了交谈,从种子阶段的初创企业到大型上市企业不一而足。我们在两个月前和上周与他们交谈,以捕捉变化的速度。由于许多创始人和开发人员正在寻求其 AI 战略,我们希望分享我们的发现,即使这个领域正在迅速发展。
1. Sequoia 网络中的几乎每家公司都在将语言模型集成到其产品中。
我们看到了神奇的自动补全功能,用于从代码(Sourcegraph、Warp、Github)到数据科学(Hex)的各种用途。我们看到了更好的聊天机器人,用于从客户支持到员工支持,再到消费者娱乐的各个方面。其他人则通过 AI 优先的视角重新设计了整个工作流程:视觉艺术(Midjourney)、营销(Hubspot、Attentive、Drift、Jasper、Copy、Writer)、销售(Gong)、联系中心(Cresta)、法律(Ironclad、Harvey)、会计(Pilot)、生产力(Notion)、数据工程(dbt)、搜索(Glean、Neeva)、杂货购物(Instacart)、消费者支付(Klarna)和旅行计划(Airbnb)。这只是一些例子,而且这还只是个开始。
......
2. 这些应用程序的新堆栈集中在语言模型 API、检索和编排上,但开源使用也在增长。
65% 的应用程序已经投入生产,比两个月前的 50% 要高,其余的仍在试验阶段。
94% 使用基础模型 API。在我们的样本中,OpenAI 的 GPT 明显是最受欢迎的,达到了 91%,不过 Anthropic 的兴趣在上个季度增长了 15%。(一些公司使用多个模型)。
88% 认为检索机制(例如向量数据库)将继续是他们的堆栈的关键部分。为模型提供有关推理的相关上下文有助于提高结果的质量,减少“幻觉”(不准确),并解决数据新鲜度问题。有些公司使用专门构建的向量数据库(Pinecone、Weaviate、Chroma、Qdrant、Milvus 等),而其他公司则使用 pgvector 或 AWS 提供的工具。
38% 对类似 LangChain 的 LLM 协同和应用程序开发框架感兴趣。有些人用它进行原型设计,而有些人则在生产中使用它。采用率在过去几个月中有所增加。
不到 10% 的人正在寻找监视 LLM 输出、成本或性能以及 A/B 测试提示的工具。我们认为,随着更多大型企业和受监管的行业采用语言模型,对这些领域的兴趣可能会增加。
...
报告原文:
ChatGPT unleashed a tidal wave of innovation with large language models (LLMs). More companies than ever before are bringing the power of natural language interaction to their products. The adoption of language model APIs is creating a new stack in its wake. To better understand the applications people are building and the stacks they are using to do so, we spoke with 33 companies across the Sequoia network, from seed stage startups to large public enterprises. We spoke with them two months ago and last week to capture the pace of change. As many founders and builders are in the midst of figuring out their AI strategies themselves, we wanted to share our findings even as this space is rapidly evolving.
1. Nearly every company in the Sequoia network is building language models into their products. We’ve seen magical auto-complete features for everything from code (Sourcegraph, Warp, Github) to data science (Hex). We’ve seen better chatbots for everything from customer support to employee support to consumer entertainment. Others are reimagining entire workflows with an AI-first lens: visual art (Midjourney), marketing (Hubspot, Attentive, Drift, Jasper, Copy, Writer), sales (Gong), contact centers (Cresta), legal (Ironclad, Harvey), accounting (Pilot), productivity (Notion), data engineering (dbt), search (Glean, Neeva), grocery shopping (Instacart), consumer payments (Klarna), and travel planning (Airbnb). These are just a few examples and they’re only the beginning.
2. The new stack for these applications centers on language model APIs, retrieval, and orchestration, but open source usage is also growing.
65% had applications in production, up from 50% two months ago, while the remainder are still experimenting.
94% are using a foundation model API. OpenAI’s GPT was the clear favorite in our sample at 91%, however Anthropic interest grew over the last quarter to 15%. (Some companies are using multiple models).
88% believe a retrieval mechanism, such as a vector database, would remain a key part of their stack. Retrieving relevant context for a model to reason about helps increase the quality of results, reduce “hallucinations” (inaccuracies), and solve data freshness issues. Some use purpose-built vector databases (Pinecone, Weaviate, Chroma, Qdrant, Milvus, and many more), while others use pgvector or AWS offerings.
38% were interested in an LLM orchestration and application development framework like LangChain. Some use it for prototyping, while others use it in production. Adoption increased in the last few months.
Sub-10% were looking for tools to monitor LLM outputs, cost, or performance and A/B test prompts. We think interest in these areas may increase as more large companies and regulated industries adopt language models.
A handful of companies are looking into complementary generative technologies, such as combining generative text and voice. We also believe this is an exciting growth area.
15% built custom language models from scratch or open source, often in addition to using LLM APIs. Custom model training increased meaningfully from a few months ago. This requires its own stack of compute, model hub, hosting, training frameworks, experiment tracking and more from beloved companies like Hugging Face, Replicate, Foundry, Tecton, Weights & Biases, PyTorch, Scale, and more.
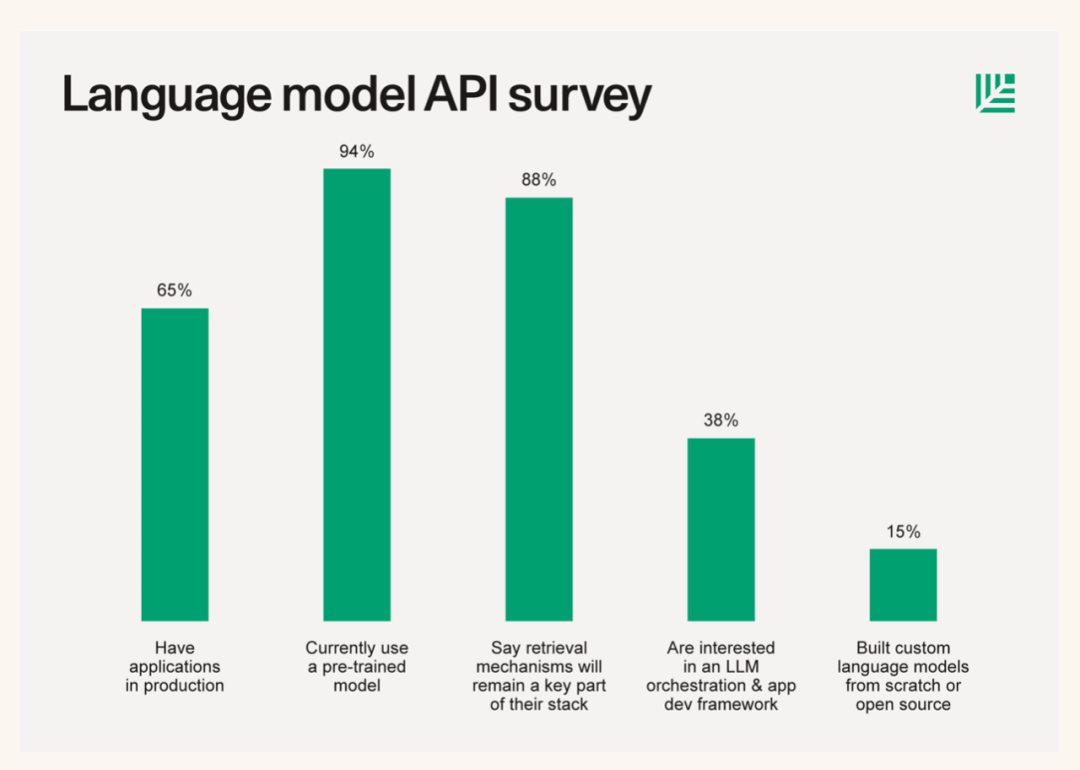
Every practitioner we spoke with said AI is moving too quickly to have high confidence in the end-state stack, but there was consensus that LLM APIs will remain a key pillar, followed in popularity by retrieval mechanisms and development frameworks like LangChain. Open source and custom model training and tuning also seem to be on the rise. Other areas of the stack are important, but earlier in maturity.
评论