机器人 SLAM 建图与自主导航:从基础到实践
- 2024-11-23 四川
本文字数:10803 字
阅读完需:约 35 分钟
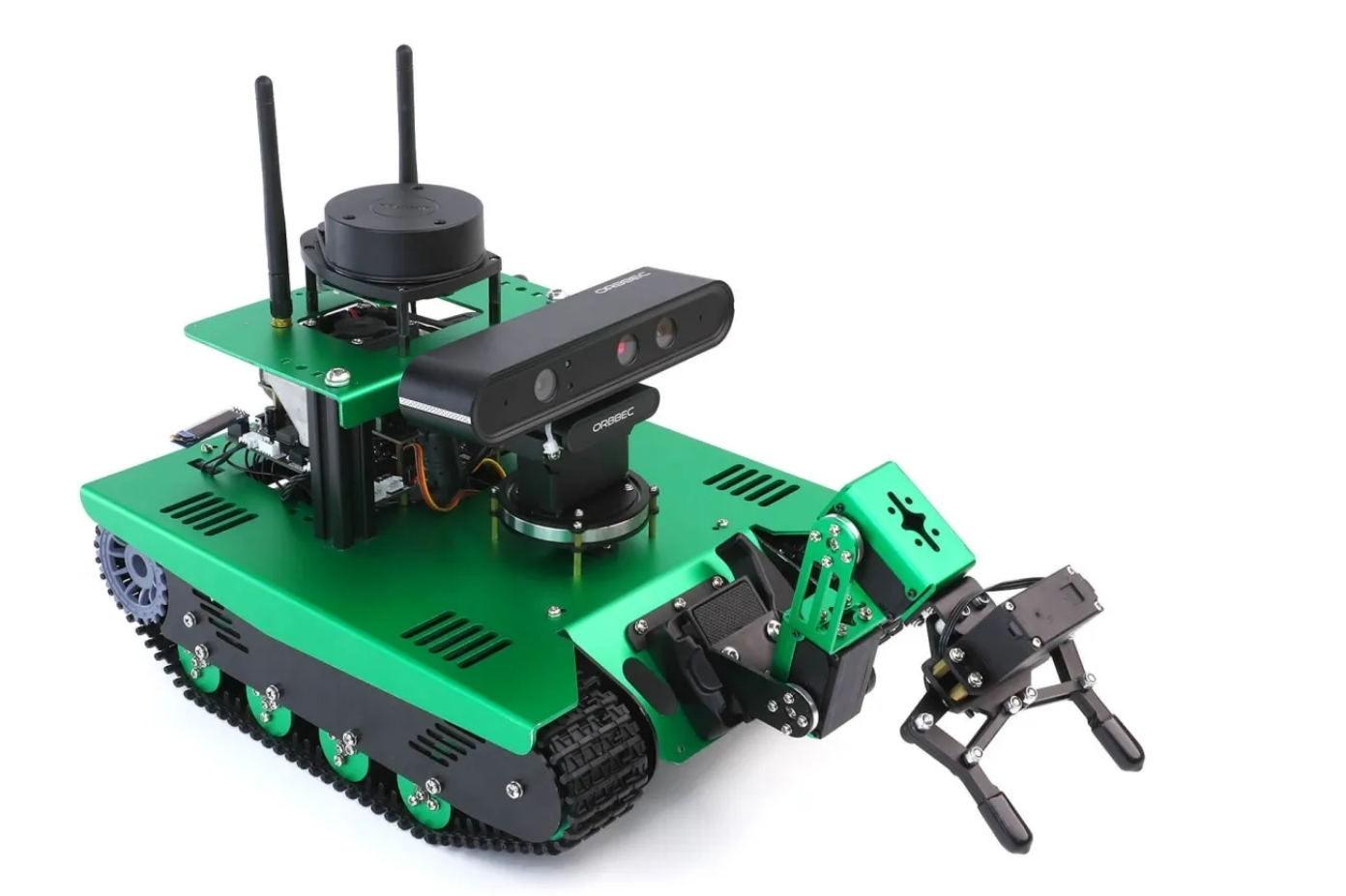
前言
这篇文章我开始和大家一起探讨机器人 SLAM 建图与自主导航 ,在前面的内容中,我们介绍了差速轮式机器人的概念及应用,谈到了使用 Gazebo 平台搭建仿真环境的教程,主要是利用 gmapping slam 算法,生成一张二维的仿真环境地图 。我们也会在这篇文章中继续介绍并使用这片二维的仿真环境地图,用于我们的演示。
教程
SLAM 算法的引入
(1)SLAM:Simultaneous Localization and Mapping,中文是即时定位与地图构建,所谓的 SLAM 算法准确说是能实现 SLAM 功能的算法,而不是某一个具体算法。
(2)现在各种机器人研发和商用化非常火 ,所有的自主机器人都绕不开一个问题,即在陌生环境中,需要知道周边是啥样(建图),需要知道我在哪(定位),于是有了 SLAM 课题的研究。SLAM 在室内机器人,自动驾驶汽车建图,VR/AR 穿戴等领域都有广泛的应用。
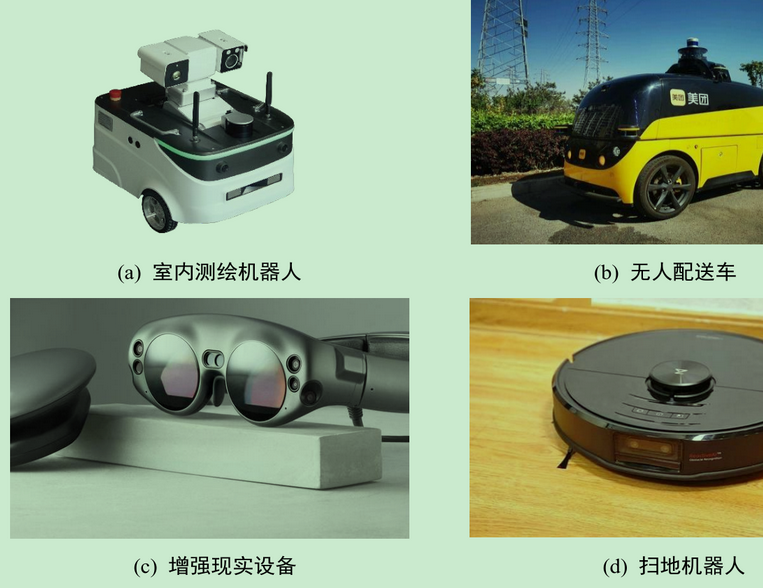
(3)SLAM 算法根据依赖的传感器不同,可以分为激光 SLAM 和视觉 SLAM,前者是激光雷达,后者是能提供深度信息的摄像头,如双目摄像头,红外摄像头等。除此之外,SLAM 算法通常还依赖里程计提供距离信息,否则地图很难无缝的拼接起来,很容易跑飞。一个经典的 SLAM 流程框架如下,其中回环检测时为了判断机器人有没有来过之前的位置。
整体视觉 SLAM 的流程图
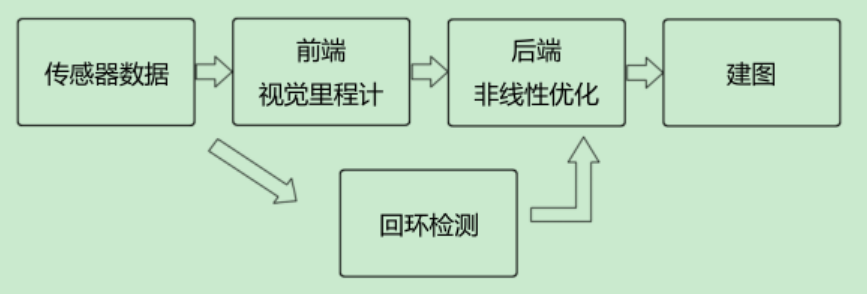
gmapping 算法的基本原理
(1)现在 ROS 里有一系列 SLAM 算法包,如:gmapping ,hector(不需要里程计,比较特别),谷歌开源的 cartographer(效率高),rtabmap(前面是二维的,这是三维建图)等。
(2)gmapping 是基于激光雷达的,需要里程计信息,创建二维格栅地图。其中 IMU 信息可以没有 。
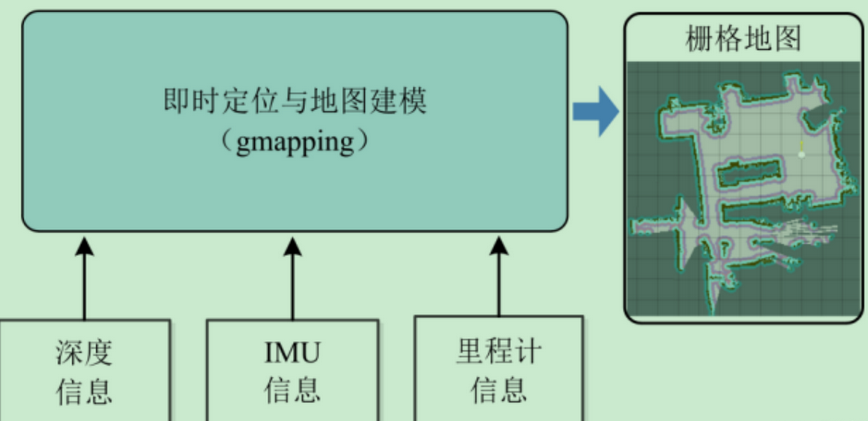
(3)ros 中激光雷达数据消息是 sensor_msgs/LaserScan ,内容如下:
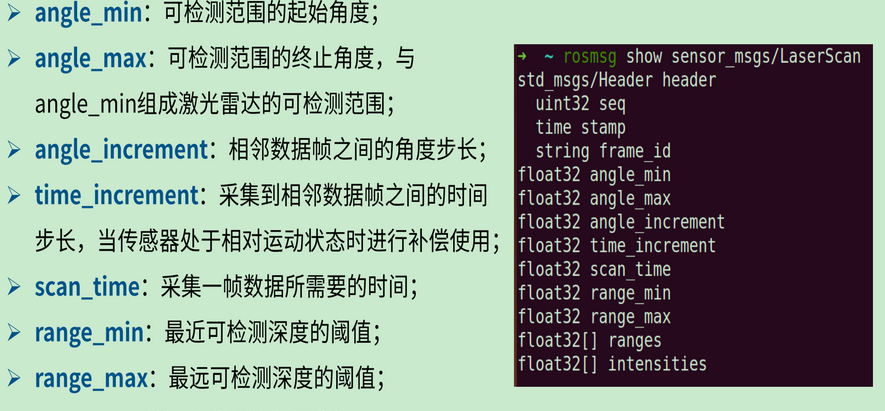
(4)ros 中里程计数据消息是 nav_msgs/Odometry 。
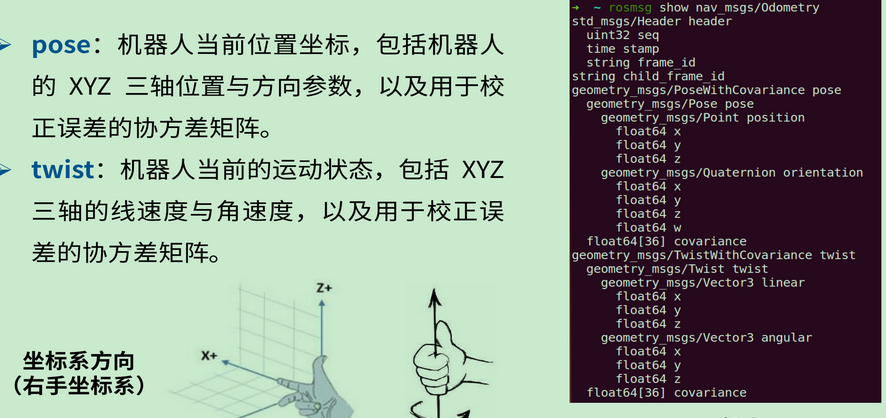
(5)gmapping 发布的地图 meta 数据:
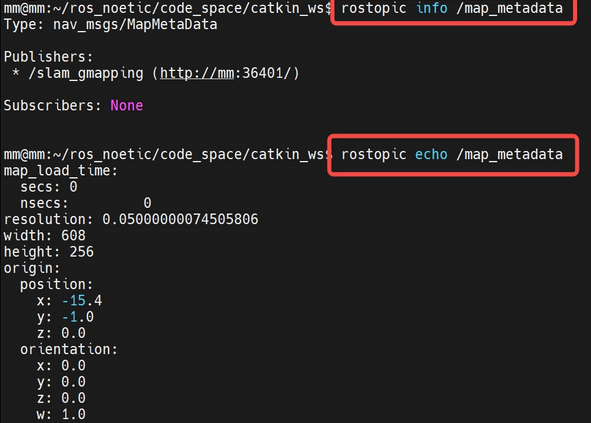
(6)gmapping 发布的地图栅格数据
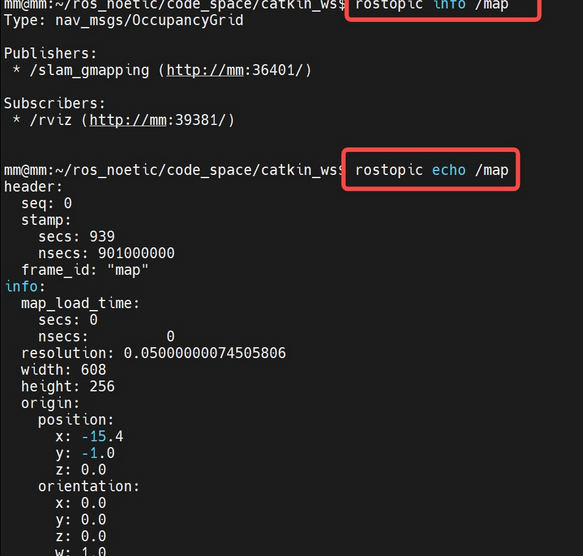
mbot_navigation
(1)ubuntu20.04 + ros noetic 下,安装 gmapping 和保存地图文件的 map_server
sudo apt-get install ros-noetic-gmapping
sudo apt-get install ros-noetic-map-server
// 补充:这是安装hector
sudo apt-get install ros-noetic-hector-slam
(2)创建 mbot_navigation 和相关文件
cd ~/catkin_ws/src
catkin_create_pkg mbot_navigation geometry_msgs move_base_msgs actionlib roscpp rospy
cd mbot_navigation
mkdir launch maps rviz
touch launch/gmapping.launch
(3)调用 gmapping 算法,只需要写 launch 文件就行了,不用编码。gmapping.launch
<launch>
// mbot_gazebo 会通过发/scan topic,传出lidar数据
<arg name="scan_topic" default="scan" />
// gammping一大堆参数,这里都是从他的demo里扣出来的,不用改。
// 如果想用的好,可以尝试修改,甚至改一些代码,这就是算法(调参)工程师!
<node pkg="gmapping" type="slam_gmapping" name="slam_gmapping" output="screen" clear_params="true">
// mbot_gazebo 会通过发/odom topic,传出里程计数据
<param name="odom_frame" value="odom"/>
<param name="map_update_interval" value="5.0"/>
<!-- Set maxUrange < actual maximum range of the Laser -->
<param name="maxRange" value="5.0"/>
<param name="maxUrange" value="4.5"/>
<param name="sigma" value="0.05"/>
<param name="kernelSize" value="1"/>
<param name="lstep" value="0.05"/>
<param name="astep" value="0.05"/>
<param name="iterations" value="5"/>
<param name="lsigma" value="0.075"/>
<param name="ogain" value="3.0"/>
<param name="lskip" value="0"/>
<param name="srr" value="0.01"/>
<param name="srt" value="0.02"/>
<param name="str" value="0.01"/>
<param name="stt" value="0.02"/>
<param name="linearUpdate" value="0.5"/>
<param name="angularUpdate" value="0.436"/>
<param name="temporalUpdate" value="-1.0"/>
<param name="resampleThreshold" value="0.5"/>
<param name="particles" value="80"/>
<param name="xmin" value="-1.0"/>
<param name="ymin" value="-1.0"/>
<param name="xmax" value="1.0"/>
<param name="ymax" value="1.0"/>
<param name="delta" value="0.05"/>
<param name="llsamplerange" value="0.01"/>
<param name="llsamplestep" value="0.01"/>
<param name="lasamplerange" value="0.005"/>
<param name="lasamplestep" value="0.005"/>
<remap from="scan" to="$(arg scan_topic)"/>
</node>
// 保存的rviz配置文件
<node pkg="rviz" type="rviz" name="rviz" args="-d $(find mbot_navigation)/rviz/map.rviz"/>
</launch>
(4)连同 mbot_gazebo,一起编译运行
cd ~/catkin_ws
catkin_make -DCATKIN_WHITELIST_PACKAGES="mbot_navigation;mbot_gazebo"
source devel/setup.bash
// 打开仿真环境
roslaunch mbot_gazebo mbot_gazebo.launch
//再开一个窗口,打开gmapping
roslaunch mbot_navigation gmapping.launch
// 控制机器人行动,进行建图
roslaunch mbot_gazebo mbot_teletop.launch
// 建图完成后,新开窗口,执行map_server,保存生成的地图
cd ~/catkin_ws/src/mbot_navigation/maps
rosrun map_server map_saver -f gmapping_save
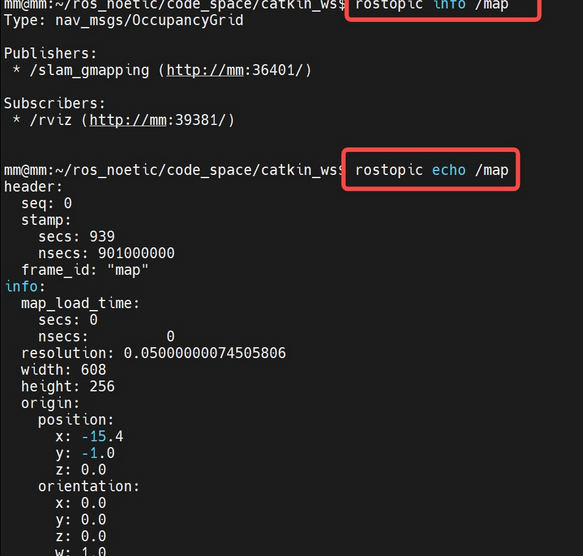
最终保存下来的地图
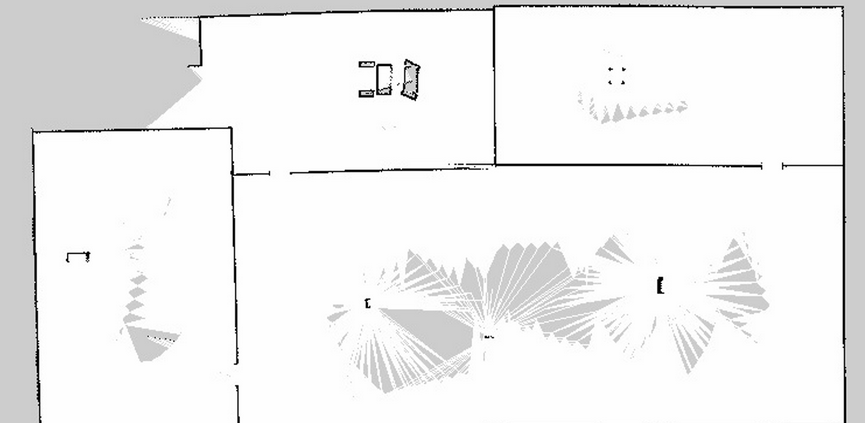
总结
在 github 上面的访问地址:https://github.com/Jieshoudaxue/ros_senior/tree/main/mbot_navigation/config/move_base
代码示例:
#include <ros/ros.h>
#include <list>
#include <geometry_msgs/Pose.h>
#include <move_base_msgs/MoveBaseAction.h>
#include <actionlib/client/simple_action_client.h>
geometry_msgs::Pose createPose(double px, double py, double pz, double ox, double oy, double oz, double ow) {
geometry_msgs::Pose pose;
pose.position.x = px;
pose.position.y = py;
pose.position.z = pz;
pose.orientation.x = ox;
pose.orientation.y = oy;
pose.orientation.z = oz;
pose.orientation.w = ow;
return pose;
}
int main(int argc, char** argv) {
ros::init(argc, argv, "move_test");
actionlib::SimpleActionClient<move_base_msgs::MoveBaseAction> move_base_client("move_base", true);
ROS_INFO("Waiting for move_base action server...");
move_base_client.waitForServer();
ROS_INFO("connected to move base server");
std::vector<geometry_msgs::Pose> target_list;
target_list.push_back(createPose(6.543, 4.779, 0.000, 0.000, 0.000, 0.645, 0.764));
target_list.push_back(createPose(5.543, -4.779, 0.000, 0.000, 0.000, 0.645, 0.764));
target_list.push_back(createPose(-5.543, 4.779, 0.000, 0.000, 0.000, 0.645, 0.764));
target_list.push_back(createPose(-5.543, -4.779, 0.000, 0.000, 0.000, 0.645, 0.764));
for (uint8_t i = 0; i < target_list.size(); i ++) {
ros::Time start_time = ros::Time::now();
ROS_INFO("going to %u goal, position: (%f, %f)", i, target_list[i].position.x, target_list[i].position.y);
move_base_msgs::MoveBaseGoal goal;
goal.target_pose.header.frame_id = "map";
goal.target_pose.header.stamp = ros::Time::now();
goal.target_pose.pose = target_list[i];
move_base_client.sendGoal(goal);
move_base_client.waitForResult();
if (move_base_client.getState() == actionlib::SimpleClientGoalState::SUCCEEDED) {
ros::Duration running_time = ros::Time::now() - start_time;
ROS_INFO("go to %u goal succeeded, running time %f sec", i, running_time.toSec());
} else {
ROS_INFO("goal failed");
}
}
return 0;
}
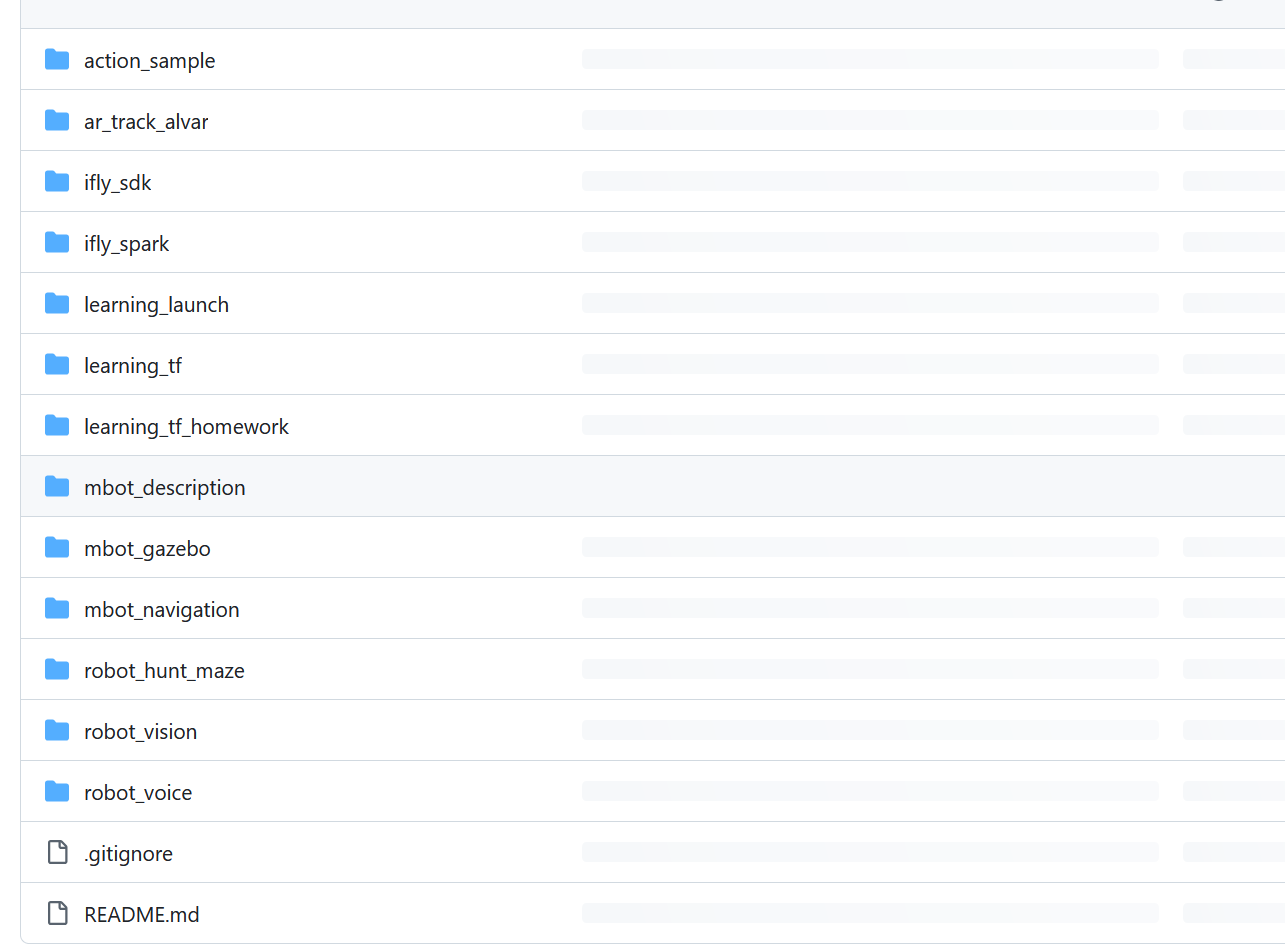
# YOLOv5 🚀 by Ultralytics, GPL-3.0 license
"""
YOLO-specific modules
Usage:
$ python path/to/models/yolo.py --cfg yolov5s.yaml
"""
import argparse
import sys
from copy import deepcopy
from pathlib import Path
FILE = Path(__file__).resolve()
ROOT = FILE.parents[1] # YOLOv5 root directory
if str(ROOT) not in sys.path:
sys.path.append(str(ROOT)) # add ROOT to PATH
# ROOT = ROOT.relative_to(Path.cwd()) # relative
from models.common import *
from models.experimental import *
from utils.autoanchor import check_anchor_order
from utils.general import LOGGER, check_version, check_yaml, make_divisible, print_args
from utils.plots import feature_visualization
from utils.torch_utils import fuse_conv_and_bn, initialize_weights, model_info, scale_img, select_device, time_sync
try:
import thop # for FLOPs computation
except ImportError:
thop = None
class Detect(nn.Module):
stride = None # strides computed during build
onnx_dynamic = False # ONNX export parameter
def __init__(self, nc=80, anchors=(), ch=(), inplace=True): # detection layer
super().__init__()
self.nc = nc # number of classes
self.no = nc + 5 # number of outputs per anchor
self.nl = len(anchors) # number of detection layers
self.na = len(anchors[0]) // 2 # number of anchors
self.grid = [torch.zeros(1)] * self.nl # init grid
self.anchor_grid = [torch.zeros(1)] * self.nl # init anchor grid
self.register_buffer('anchors', torch.tensor(anchors).float().view(self.nl, -1, 2)) # shape(nl,na,2)
self.m = nn.ModuleList(nn.Conv2d(x, self.no * self.na, 1) for x in ch) # output conv
self.inplace = inplace # use in-place ops (e.g. slice assignment)
def forward(self, x):
z = [] # inference output
for i in range(self.nl):
x[i] = self.m[i](x[i]) # conv
bs, _, ny, nx = x[i].shape # x(bs,255,20,20) to x(bs,3,20,20,85)
x[i] = x[i].view(bs, self.na, self.no, ny, nx).permute(0, 1, 3, 4, 2).contiguous()
if not self.training: # inference
if self.onnx_dynamic or self.grid[i].shape[2:4] != x[i].shape[2:4]:
self.grid[i], self.anchor_grid[i] = self._make_grid(nx, ny, i)
y = x[i].sigmoid()
if self.inplace:
y[..., 0:2] = (y[..., 0:2] * 2 - 0.5 + self.grid[i]) * self.stride[i] # xy
y[..., 2:4] = (y[..., 2:4] * 2) ** 2 * self.anchor_grid[i] # wh
else: # for YOLOv5 on AWS Inferentia https://github.com/ultralytics/yolov5/pull/2953
xy = (y[..., 0:2] * 2 - 0.5 + self.grid[i]) * self.stride[i] # xy
wh = (y[..., 2:4] * 2) ** 2 * self.anchor_grid[i] # wh
y = torch.cat((xy, wh, y[..., 4:]), -1)
z.append(y.view(bs, -1, self.no))
return x if self.training else (torch.cat(z, 1), x)
def _make_grid(self, nx=20, ny=20, i=0):
d = self.anchors[i].device
if check_version(torch.__version__, '1.10.0'): # torch>=1.10.0 meshgrid workaround for torch>=0.7 compatibility
yv, xv = torch.meshgrid([torch.arange(ny, device=d), torch.arange(nx, device=d)], indexing='ij')
else:
yv, xv = torch.meshgrid([torch.arange(ny, device=d), torch.arange(nx, device=d)])
grid = torch.stack((xv, yv), 2).expand((1, self.na, ny, nx, 2)).float()
anchor_grid = (self.anchors[i].clone() * self.stride[i]) \
.view((1, self.na, 1, 1, 2)).expand((1, self.na, ny, nx, 2)).float()
return grid, anchor_grid
class Model(nn.Module):
def __init__(self, cfg='yolov5s.yaml', ch=3, nc=None, anchors=None): # model, input channels, number of classes
super().__init__()
if isinstance(cfg, dict):
self.yaml = cfg # model dict
else: # is *.yaml
import yaml # for torch hub
self.yaml_file = Path(cfg).name
with open(cfg, encoding='ascii', errors='ignore') as f:
self.yaml = yaml.safe_load(f) # model dict
# Define model
ch = self.yaml['ch'] = self.yaml.get('ch', ch) # input channels
if nc and nc != self.yaml['nc']:
LOGGER.info(f"Overriding model.yaml nc={self.yaml['nc']} with nc={nc}")
self.yaml['nc'] = nc # override yaml value
if anchors:
LOGGER.info(f'Overriding model.yaml anchors with anchors={anchors}')
self.yaml['anchors'] = round(anchors) # override yaml value
self.model, self.save = parse_model(deepcopy(self.yaml), ch=[ch]) # model, savelist
self.names = [str(i) for i in range(self.yaml['nc'])] # default names
self.inplace = self.yaml.get('inplace', True)
# Build strides, anchors
m = self.model[-1] # Detect()
if isinstance(m, Detect):
s = 256 # 2x min stride
m.inplace = self.inplace
m.stride = torch.tensor([s / x.shape[-2] for x in self.forward(torch.zeros(1, ch, s, s))]) # forward
m.anchors /= m.stride.view(-1, 1, 1)
check_anchor_order(m)
self.stride = m.stride
self._initialize_biases() # only run once
# Init weights, biases
initialize_weights(self)
self.info()
LOGGER.info('')
def forward(self, x, augment=False, profile=False, visualize=False):
if augment:
return self._forward_augment(x) # augmented inference, None
return self._forward_once(x, profile, visualize) # single-scale inference, train
def _forward_augment(self, x):
img_size = x.shape[-2:] # height, width
s = [1, 0.83, 0.67] # scales
f = [None, 3, None] # flips (2-ud, 3-lr)
y = [] # outputs
for si, fi in zip(s, f):
xi = scale_img(x.flip(fi) if fi else x, si, gs=int(self.stride.max()))
yi = self._forward_once(xi)[0] # forward
# cv2.imwrite(f'img_{si}.jpg', 255 * xi[0].cpu().numpy().transpose((1, 2, 0))[:, :, ::-1]) # save
yi = self._descale_pred(yi, fi, si, img_size)
y.append(yi)
y = self._clip_augmented(y) # clip augmented tails
return torch.cat(y, 1), None # augmented inference, train
def _forward_once(self, x, profile=False, visualize=False):
y, dt = [], [] # outputs
for m in self.model:
if m.f != -1: # if not from previous layer
x = y[m.f] if isinstance(m.f, int) else [x if j == -1 else y[j] for j in m.f] # from earlier layers
if profile:
self._profile_one_layer(m, x, dt)
x = m(x) # run
y.append(x if m.i in self.save else None) # save output
if visualize:
feature_visualization(x, m.type, m.i, save_dir=visualize)
return x
def _descale_pred(self, p, flips, scale, img_size):
# de-scale predictions following augmented inference (inverse operation)
if self.inplace:
p[..., :4] /= scale # de-scale
if flips == 2:
p[..., 1] = img_size[0] - p[..., 1] # de-flip ud
elif flips == 3:
p[..., 0] = img_size[1] - p[..., 0] # de-flip lr
else:
x, y, wh = p[..., 0:1] / scale, p[..., 1:2] / scale, p[..., 2:4] / scale # de-scale
if flips == 2:
y = img_size[0] - y # de-flip ud
elif flips == 3:
x = img_size[1] - x # de-flip lr
p = torch.cat((x, y, wh, p[..., 4:]), -1)
return p
def _clip_augmented(self, y):
# Clip YOLOv5 augmented inference tails
nl = self.model[-1].nl # number of detection layers (P3-P5)
g = sum(4 ** x for x in range(nl)) # grid points
e = 1 # exclude layer count
i = (y[0].shape[1] // g) * sum(4 ** x for x in range(e)) # indices
y[0] = y[0][:, :-i] # large
i = (y[-1].shape[1] // g) * sum(4 ** (nl - 1 - x) for x in range(e)) # indices
y[-1] = y[-1][:, i:] # small
return y
def _profile_one_layer(self, m, x, dt):
c = isinstance(m, Detect) # is final layer, copy input as inplace fix
o = thop.profile(m, inputs=(x.copy() if c else x,), verbose=False)[0] / 1E9 * 2 if thop else 0 # FLOPs
t = time_sync()
for _ in range(10):
m(x.copy() if c else x)
dt.append((time_sync() - t) * 100)
if m == self.model[0]:
LOGGER.info(f"{'time (ms)':>10s} {'GFLOPs':>10s} {'params':>10s} {'module'}")
LOGGER.info(f'{dt[-1]:10.2f} {o:10.2f} {m.np:10.0f} {m.type}')
if c:
LOGGER.info(f"{sum(dt):10.2f} {'-':>10s} {'-':>10s} Total")
def _initialize_biases(self, cf=None): # initialize biases into Detect(), cf is class frequency
# https://arxiv.org/abs/1708.02002 section 3.3
# cf = torch.bincount(torch.tensor(np.concatenate(dataset.labels, 0)[:, 0]).long(), minlength=nc) + 1.
m = self.model[-1] # Detect() module
for mi, s in zip(m.m, m.stride): # from
b = mi.bias.view(m.na, -1) # conv.bias(255) to (3,85)
b.data[:, 4] += math.log(8 / (640 / s) ** 2) # obj (8 objects per 640 image)
b.data[:, 5:] += math.log(0.6 / (m.nc - 0.999999)) if cf is None else torch.log(cf / cf.sum()) # cls
mi.bias = torch.nn.Parameter(b.view(-1), requires_grad=True)
def _print_biases(self):
m = self.model[-1] # Detect() module
for mi in m.m: # from
b = mi.bias.detach().view(m.na, -1).T # conv.bias(255) to (3,85)
LOGGER.info(
('%6g Conv2d.bias:' + '%10.3g' * 6) % (mi.weight.shape[1], *b[:5].mean(1).tolist(), b[5:].mean()))
# def _print_weights(self):
# for m in self.model.modules():
# if type(m) is Bottleneck:
# LOGGER.info('%10.3g' % (m.w.detach().sigmoid() * 2)) # shortcut weights
def fuse(self): # fuse model Conv2d() + BatchNorm2d() layers
LOGGER.info('Fusing layers... ')
for m in self.model.modules():
if isinstance(m, (Conv, DWConv)) and hasattr(m, 'bn'):
m.conv = fuse_conv_and_bn(m.conv, m.bn) # update conv
delattr(m, 'bn') # remove batchnorm
m.forward = m.forward_fuse # update forward
self.info()
return self
def info(self, verbose=False, img_size=640): # print model information
model_info(self, verbose, img_size)
def _apply(self, fn):
# Apply to(), cpu(), cuda(), half() to model tensors that are not parameters or registered buffers
self = super()._apply(fn)
m = self.model[-1] # Detect()
if isinstance(m, Detect):
m.stride = fn(m.stride)
m.grid = list(map(fn, m.grid))
if isinstance(m.anchor_grid, list):
m.anchor_grid = list(map(fn, m.anchor_grid))
return self
def parse_model(d, ch): # model_dict, input_channels(3)
LOGGER.info(f"\n{'':>3}{'from':>18}{'n':>3}{'params':>10} {'module':<40}{'arguments':<30}")
anchors, nc, gd, gw = d['anchors'], d['nc'], d['depth_multiple'], d['width_multiple']
na = (len(anchors[0]) // 2) if isinstance(anchors, list) else anchors # number of anchors
no = na * (nc + 5) # number of outputs = anchors * (classes + 5)
layers, save, c2 = [], [], ch[-1] # layers, savelist, ch out
for i, (f, n, m, args) in enumerate(d['backbone'] + d['head']): # from, number, module, args
m = eval(m) if isinstance(m, str) else m # eval strings
for j, a in enumerate(args):
try:
args[j] = eval(a) if isinstance(a, str) else a # eval strings
except NameError:
pass
n = n_ = max(round(n * gd), 1) if n > 1 else n # depth gain
if m in [Conv, GhostConv, Bottleneck, GhostBottleneck, SPP, SPPF, DWConv, MixConv2d, Focus, CrossConv,
BottleneckCSP, C3, C3TR, C3SPP, C3Ghost]:
c1, c2 = ch[f], args[0]
if c2 != no: # if not output
c2 = make_divisible(c2 * gw, 8)
args = [c1, c2, *args[1:]]
if m in [BottleneckCSP, C3, C3TR, C3Ghost]:
args.insert(2, n) # number of repeats
n = 1
elif m is nn.BatchNorm2d:
args = [ch[f]]
elif m is Concat:
c2 = sum(ch[x] for x in f)
elif m is Detect:
args.append([ch[x] for x in f])
if isinstance(args[1], int): # number of anchors
args[1] = [list(range(args[1] * 2))] * len(f)
elif m is Contract:
c2 = ch[f] * args[0] ** 2
elif m is Expand:
c2 = ch[f] // args[0] ** 2
else:
c2 = ch[f]
m_ = nn.Sequential(*(m(*args) for _ in range(n))) if n > 1 else m(*args) # module
t = str(m)[8:-2].replace('__main__.', '') # module type
np = sum(x.numel() for x in m_.parameters()) # number params
m_.i, m_.f, m_.type, m_.np = i, f, t, np # attach index, 'from' index, type, number params
LOGGER.info(f'{i:>3}{str(f):>18}{n_:>3}{np:10.0f} {t:<40}{str(args):<30}') # print
save.extend(x % i for x in ([f] if isinstance(f, int) else f) if x != -1) # append to savelist
layers.append(m_)
if i == 0:
ch = []
ch.append(c2)
return nn.Sequential(*layers), sorted(save)
if __name__ == '__main__':
parser = argparse.ArgumentParser()
parser.add_argument('--cfg', type=str, default='yolov5s.yaml', help='model.yaml')
parser.add_argument('--device', default='', help='cuda device, i.e. 0 or 0,1,2,3 or cpu')
parser.add_argument('--profile', action='store_true', help='profile model speed')
parser.add_argument('--test', action='store_true', help='test all yolo*.yaml')
opt = parser.parse_args()
opt.cfg = check_yaml(opt.cfg) # check YAML
print_args(FILE.stem, opt)
device = select_device(opt.device)
# Create model
model = Model(opt.cfg).to(device)
model.train()
# Profile
if opt.profile:
img = torch.rand(8 if torch.cuda.is_available() else 1, 3, 640, 640).to(device)
y = model(img, profile=True)
# Test all models
if opt.test:
for cfg in Path(ROOT / 'models').rglob('yolo*.yaml'):
try:
_ = Model(cfg)
except Exception as e:
print(f'Error in {cfg}: {e}')
# Tensorboard (not working https://github.com/ultralytics/yolov5/issues/2898)
# from torch.utils.tensorboard import SummaryWriter
# tb_writer = SummaryWriter('.')
# LOGGER.info("Run 'tensorboard --logdir=models' to view tensorboard at http://localhost:6006/")
# tb_writer.add_graph(torch.jit.trace(model, img, strict=False), []) # add model graph
版权声明: 本文为 InfoQ 作者【梦笔生花】的原创文章。
原文链接:【http://xie.infoq.cn/article/1e864671ce876cbe4295da1d8】。文章转载请联系作者。
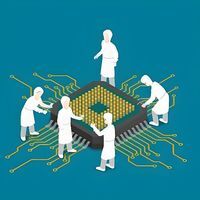
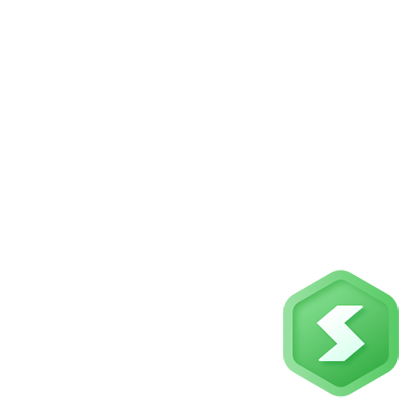
梦笔生花
凡事预则立,不预则废! 2022-06-01 加入
某公司芯片AE工程师,嵌入式开发工程师,InfoQ签约作者,阿里云专家博主,华为云·云享专家,51CTO专家博主,腾讯云社区优秀共创官。
评论