Tritonserver 在得物的最佳实践
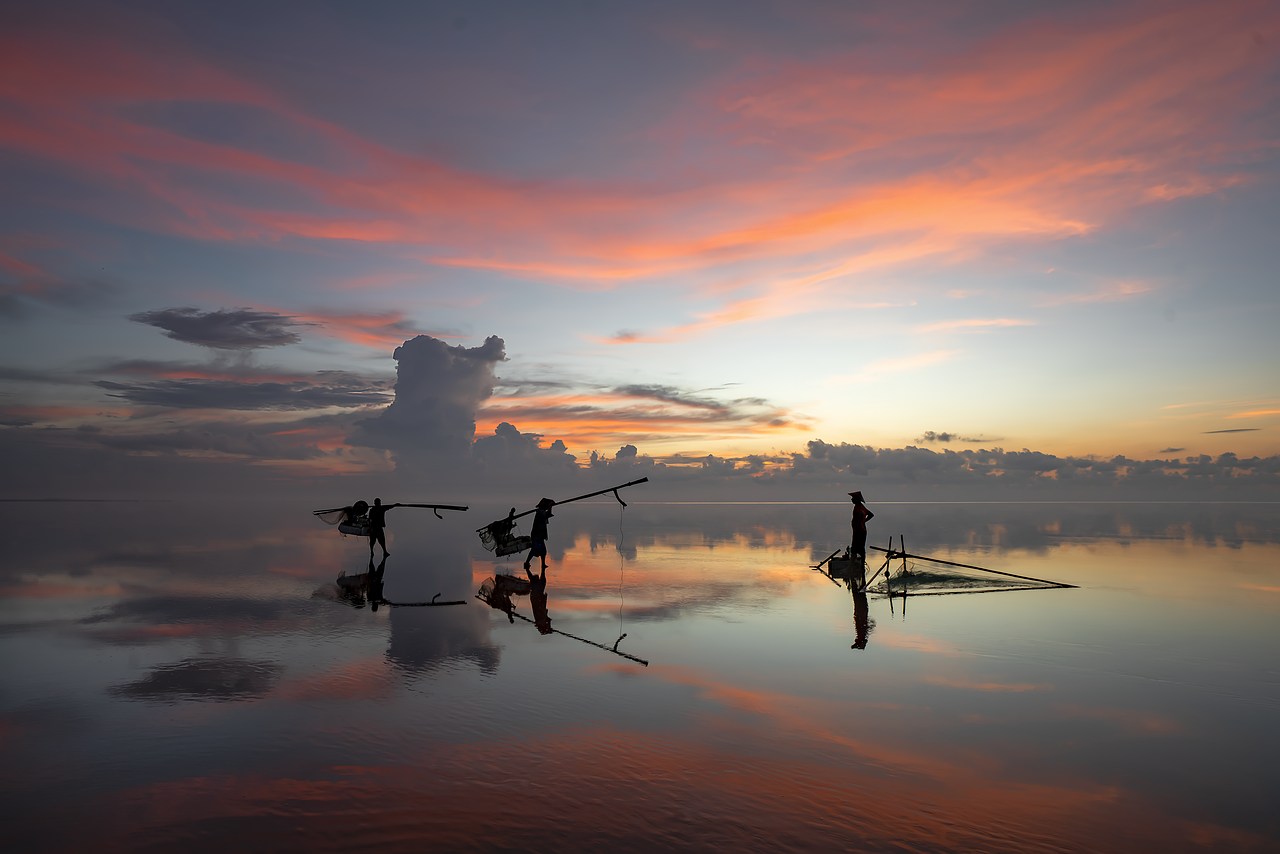
一、Tritonserver 介绍
Tritonserver 是 Nvidia 推出的基于 GPU 和 CPU 的在线推理服务解决方案,因其具有高性能的并发处理和支持几乎所有主流机器学习框架模型的特点,是目前云端的 GPU 服务高效部署的主流方案。
Tritonserver 的部署是以模型仓库(Model Repository)的形式体现的,即需要模型文件和配置文件,且按一定的格式放置如下,根目录下每个模型有各自的文件夹。
Tritonserver 有 auto-generate-config 功能,关于模型的输入(inputs)、输出(outputs)和最大 batch(max_batch_size)等可以根据对模型的分析自动生成,对 onnx, tensorrt, tf saved model 等带模型结构的模型极为方便,最简便的 config.pbtxt 可以只定义模型的 name 和 backend,例如针对上述模型:
二、部署 Features
Ensemble Pipeline
Pipeline 模式是实际生产中机器学习模型应用常见的场景,比如一个含有带 Python 代码前处理和后处理的模型,或者含有前后处理的多个模型串联成一个生产处理流程;比如下图左边的 OCR 流程,若单独部署了文字检测和文字识别模型,图像经过检测模型返回 boxes 块后,再经过 cropping 后,接着请求识别模型,最后返回识别的文字,这样在客户端和服务端之间需要交互多次;而像右图,将中间的 cropping 处理过程也视作一个 model 形式,部署在服务测,则单次请求中,服务端完成 Text Detection -> Image Cropping -> Text Recognition 处理后返回,减少了交互次数,极大降低了耗时。
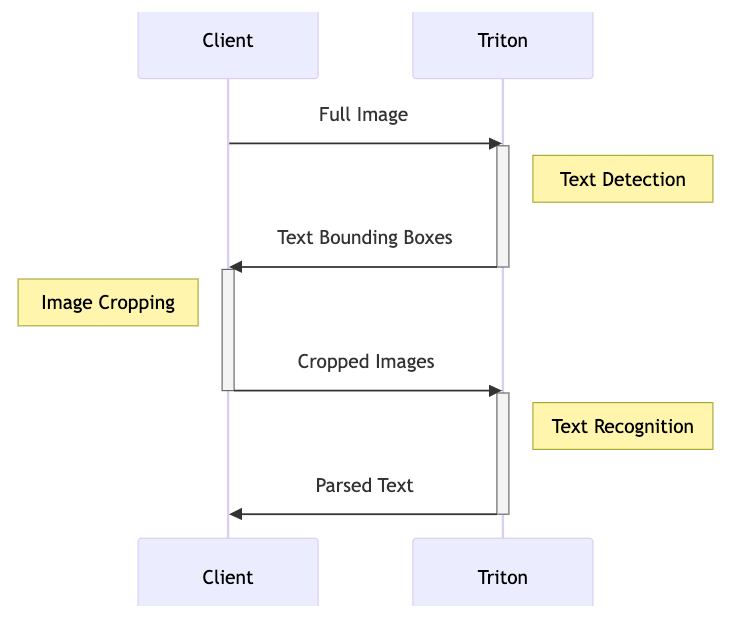
Ensemble Pipeline 需要额外定义一个 Model Repository, 里边的版本文件夹为空,config.pbtxt 中定义数据流的处理流程,指明服务端接收到数据后在各个模型之间处理的逻辑顺序,格式如下:
以上图的 OCR Pipeline 为例,除了本身的两个模型:text_detection 和 text_recognition, 我们额外定义三个 Model Repository:detection_preprocessing, detection_postprocessing, recognition_postprocessing, 分别表示检测模型的前处理,检测模型的后处理和识别模型的后处理, 按实际处理中的逻辑顺序将上述的所有模型串联如下:
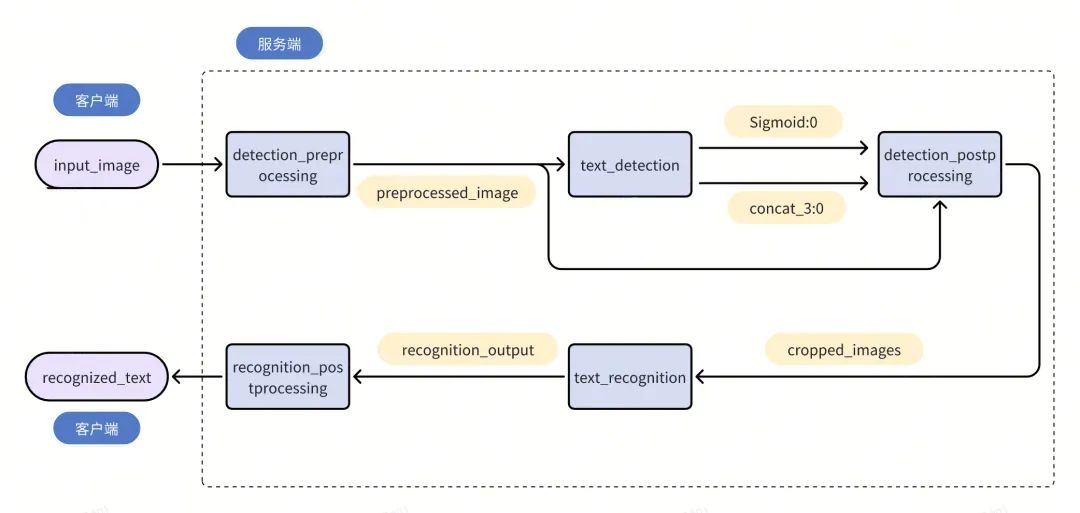
上图模型及各自输入输出之间的逻辑关系写到 ensemble_model 的配置文件里边如下。文件的信息由两部分组成,第一部分定义整个 Pipeline 的基础信息,第二部分中的 step 定义了推理时上图的逻辑关系。
Business Logic Scripting(BLS)
虽然 ensemble 特征已能支持大多数的推理 Pipeline, 但是诸如循环(loop)、条件(if...else...)或一些依赖数据的控制流逻辑还是无法实现。BLS 的本质是允许用户在定义的 python-backend 的模型的执行函数里请求其他的模型,而这样的请求可以完美实现这些自定义的逻辑。
以上述的 OCR 推理流程为例,如果 OCR 检测模型输出图片个含文字的 bounding boxes 的 patches, 经过一定的后处理,来到后边的 OCR Recoginition 模型进行识别;而识别模型的输入有一定的 batch 限制,假设为图片,那这些 patches 则需要经过图片次的推理才能完成处理,这里形成了一个 loop。
以下是具体的逻辑:
pb_utils.InferenceRequest 创建了一个对模型的请求
构建一个 for-loop,完成对模型的多次请求
收集多次请求的返回,并做一定的处理,作为当前 TritonPythonModel 的返回
Dynamic Batching
动态批次 指的是在服务处理请求时,相比于对接收的请求依次推理,允许将单个或多个请求自动组合成一个 batch 再做推理,以达到提升吞吐的目的。
以下示例对动态批次对服务提高处理请求能力,提升吞吐做了解释:
图左,按时间轴到达了 A, B, C, D, E 等不同 batch_size 的请求。
图右的第一行,无动态批次(No Dynamic Batching)设置的条件下,模型依次推理这些请求,需要 5X 的时间。
图右的第二行,设置了动态批次,但未设置等待时间(Without Delay),模型将一定时间内的批次合并,如将同时接收到的请求 A, C 合并成一个 batch_size=6 的服务做一次推理;随后将 B, D 合并成一个 batch_size=8 的服务再做一次推理(因为图中规定了模型的最大处理批次=8,所以虽然 E 和 D 是同时到达,但不能将 B, D, E 都合并处理);最后处理 E, 一共花费了 3X 的时间。
图右的第三行,为动态批次设置了等待时间,Delay=X/2,则在 X/2 的时间内,将接收到的 A, B, C 请求合成一个 batch_size=8 的请求处理;再将 D, E 合成处理,共花费了 2.5X 的时间。
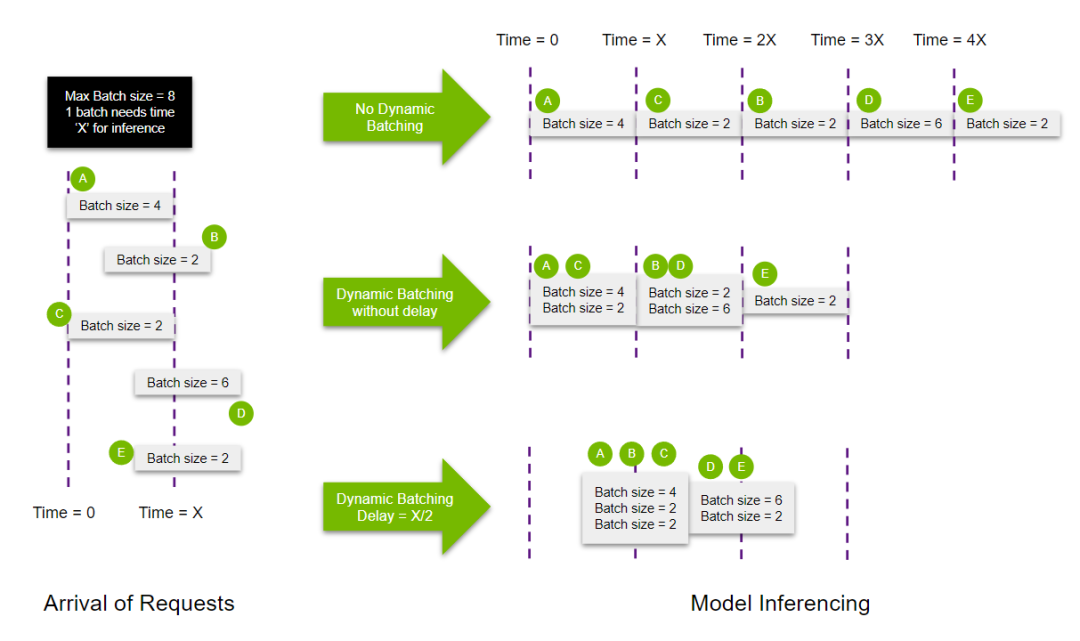
关于延时的设置,可以在 config.pbtxt 里定义如下:
而时间的值的大小设置,可以根据实际业务的吞吐量,一种方法是测试不同的取值,从而选取效果最佳的值;另一种方法是使用 Triton-client 的自带工具 model-analyzer,自动分析和检索模型配置的最佳参数。
其他详细的配置参数可以参考这里。
C/GPU 分离
在实际部署中,对服务做并发改造是提升服务吞吐的基本操作。而在 GPU 服务中,当模型训练者直接将 Pytorch 或 tensorflow 的模型直接写到服务的请求体后,随后采用 gunicorn 或 kserve 设置多个 workers 作并发时,则服务中的模型使用的显存也会成多倍的复制,造成 GPU 显存的 OOM;所以当我们做并发改造时,需要先将服务中的 GPU 推理部分与其他 CPU 处理分离,而 Tritonserver 是一个很好的选择。
首先将模型都改写成 Tritonserver 支持的部署格式(第一部分介绍的模型仓库格式),常用的手段有:
将 Pytorch 模型 export 并保存为 onnx 格式,或提取为 torchscript 格式
将 onnx、tensorflow 模型等用 tensorRT 编译加速,精度允许时可以编译成 fp16 或精度更低的格式。
当上述模型的提取较为复杂时,例如使用了 mmcv, mmdet 等复杂工具构建的 Pytorch 模型,可以直接将基于 Python 代码的模型改写成一个 TritonPythonModel 类, 写成 python-backend 的模型。
随后将模型用 Tritonserver 命令拉起服务,此处也可以用守护进程工具,例如 supervisord,启动 Tritonserver 命令,以保护 Tritonserver 进程因意外退出而中断服务。
接着,用高并发的服务工具,例如 gunicorn, kserve 改写代理服务,设置多个 CPU 的 workers, 这样整个服务的 GPU 利用率上限能大大提升,从而提升服务的吞吐上限。
三、模型的性能测试
参数优化
Model-analyzer 是一个针对模型配置的参数优化工具,其主要功能是对用户期望优化的参数通过搜索的方式得到在吞吐或延时上最优的方案。
参数搜索根据搜索的方式有以下三种:
Brute force Search: 暴力检索指定的参数及其取值范围,适用于单个模型或顺序型的 Pipeline 模型,指定命令参数 --run-config-search-mode brute
Quick Search:基于爬坡算法(hill-climbing)找到参数最优解,适用于所有简单或复杂的模型,指定命令参数 --run-config-search-mode quick
Optuna search: 使用带超参的优化算法找到最优解,适用于所有简单或复杂的模型,指定命令参数 --run-config-search-mode optuna
实际部署中的多数业务,brute 和 quick 两种方法基本能够快速解决参数配置。
使用 model-analyzer 的 profile 命令和相应的参数配置拉起参数搜索程序:
也可以将这些参数都配在一个 yaml 文件里,直接指定配置文件拉起更方便:
可以简单地配置一些基本的参数:
程序运行完成后会给出一个关于最优配置的报告,以及所有已尝试的配置的配置文件,用户可以直接使用给出的最优配置文件拉起模型的服务。
以 bert 模型为例,检索合适的 instances, delay 等参数,最后的报告中列出了几个较优解,再从其中选择,比如,最终根据 GPU 资源的用量和 p99 latency 选择了 “rel_cross_bert_l20_trt_config_11” 这个模型配置。
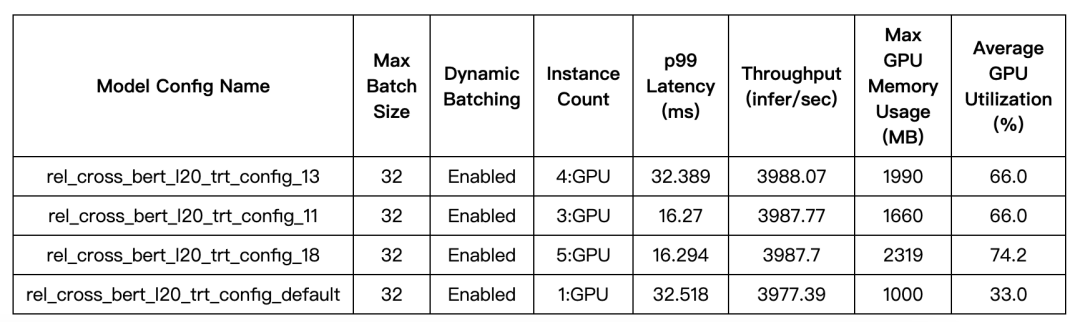
更详细的配置说明可以参考这两个官方文档:
https://github.com/triton-inference-server/model_analyzer/blob/main/docs/config.md
https://github.com/triton-inference-server/model_analyzer/blob/main/docs/config_search.md
性能压测
perf-analyzer 是 Tritonclient 携带的一个模型性能压测工具,可以按提供的输入数据格式压测模型的性能,既可以观察模型在不同的并发量的压测下的吞吐和延时的性能,也可以模拟在特定的吞吐下模型的延时性能。
以一个 bert 模型为例,其输入 input_id 为[1, 128]的 int64 类,则可以先编写一个用以提供输入示例的 json 文件。
随后输入一下命令进行压测:
bert_ensemble 是我们想压测的模型的名称
-b 指定以多大的 batch 作为输入
--input-data 指定了写有输入示例的 json 格式的文件
--measurement-interval 压测的时间间隔,单位为 ms
--concurrency-range 指定压测的并发数,可以是一个范围,如 1:10 表示压测并发分别为 1, 2, ..., 10 时的情况。
--percentile=90 返回 p90 的延时信息。
压测完毕, 除了会返回相应的并发量下模型的 QPS,平均 RT 这些基础信息,还会返回各类 P50, P90, P99 等延时,Pipeline 中每个子模块的延时,每个子模块的延时中的排队延时、推理延时、输入处理延时、输出处理延时等这些极为详细的信息。
也可以指定模型的在特定的 QPS 下压测,如:
--request-rate-range 指定了模型在吞吐为 20 情况下压测
四、Tritonserver 在得物的最佳实践
模型管理
用户可以在 KubeAI 平台先上传模型,“模型列表” ->“新增模型”,填写相关信息以及 oss 地址。
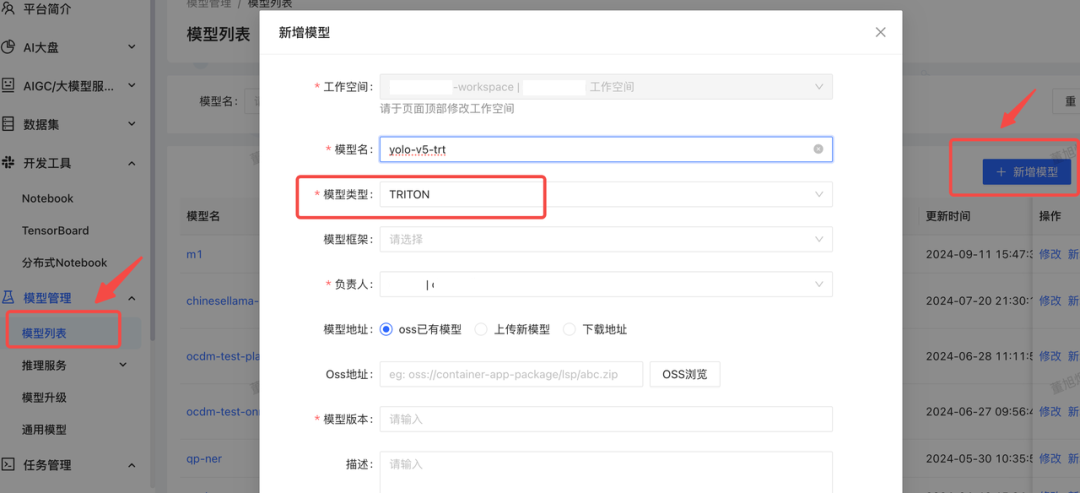
一键部署
对于简单的 single model 或已有 Triton 配置文件的模型仓库,可以直接在 KubeAI 的“Triton 服务”创建服务,简单选择环境、模型、模型版本和 GPU 配置等资源即可拉起。

Pytorch 和 Tensorflow
许多 python-backend 的模型,直接使用 Pytorch 或 TensorFlow 的 Python 接口加载模型或处理数据,我们也提供了自带安装 Pytorch 2.0 和 TensorFlow 2.9 的 Python 包的镜像,可以在部署服务时替换
repoin.shizhuang-inc.net/app-runtime/kubeai/triton-22.12-py3-deploy:v1.31-torch
repoin.shizhuang-inc.net/app-runtime/kubeai/triton-22.12-py3-deploy:v1.32-tf2
基于代码的普通服务部署
如果用户需要安装额外的 Python 库,也可以直接使用上述基于 Triton 的镜像在 KubeAI 的“普通推理服务”基于 GitLab 代码部署对应的 Tritonserver 服务。

五、总结
总的来说,Tritonserver 是目前非常成熟的在线推理框架:
不管是利用 Tritonserver 直接提供推理服务,还是用代理服务+C/GPU 分离,或是结合 rayserve 等可自动弹性伸缩的框架,都能充分利用 GPU 的算力,体现在线服务的高效并发性。
Tritonserver 提供的 Pipeline 模式加上 BLS、以及对 python-backend 的支持,基本上能满足算法开发者所有的逻辑功能的设计,支持绝大多数离线开发的模型服务移植到线上。
Tritonserver 支持目前绝大多数的模型类型作为 backend;甚至是目前深度学习最火热的大模型所支持的主流推理框架,Tritonserver 也能结合 vLLM、或者其原生的 Tensor-LLM-backend 提供优秀的并发服务。
*文/xujiong
本文属得物技术原创,更多精彩文章请看:得物技术
未经得物技术许可严禁转载,否则依法追究法律责任!
评论