目标检测之 RetinaNet
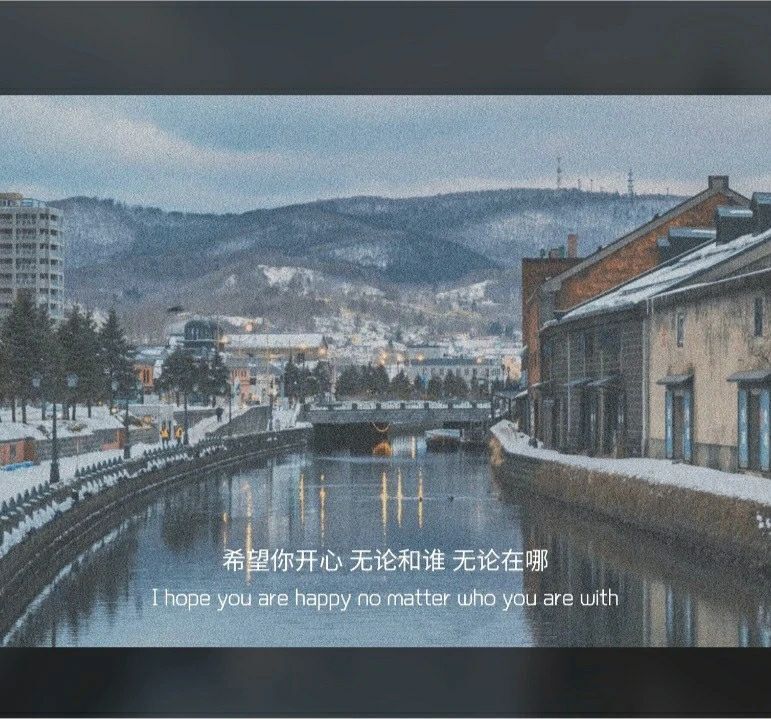
FocalLoss
author: dreamer
date: 2020-0916
对于one stage的正负样本不均衡的情况下提出的计算损失的方式,提出了RetinaNet同时满足快速和准确的预测。可以达到双阶段目标检测效果的单阶段预测网络。
作者发现对比双阶段的网络,单阶段网络由于没有RPN来提出出更有可能出现目标的ROI,网络会计算大量的ROI区域,导致训练的时候出现大量的easy example。因此本文提出一个新的loss function,可以自动的减少easy example对loss 的影响。需要注意的是,focal loss的具体形式并不重要,可以用别的形式实现相同的效果。
Relate work
class Imblance
训练中出现大量的easy example,导致网络学习不到有用的信息
减弱网络的性能
3.1 Balanced Cross Entropy

自定义权重的loss function, 平衡正负样本不均衡的权重
3.2 Focal Loss Function

这是加入权重是为了改变难易样本的权重
实际中使用的公式:

3.4 Class Imbalance and Two-stage Detectors
two stage中不使用loss权重参数的原因:
1. two stage cascade
2. biased minibatch sampling
RetinaNet
Feature Pyramid Network Backbone: based on Resnet
anchior: 每个level9个anchor
Classification Subnet:4 (3\3Conv + ReLu)+ 3\*3Conv
Box Regression Subnet:用上,区别是最终的输出不同
评论