极客时间架构师训练营 - week5 - 作业 1
发布于: 2020 年 07 月 08 日
用你熟悉的编程语言实现一致性 hash 算法。
编写测试用例测试这个算法,测试 100 万 KV 数据,10 个服务器节点的情况下,计算这些 KV 数据在服务器上分布数量的标准差,以评估算法的存储负载不均衡性。
本周作业对于我而言比较吃力,搜索了极客时间的多门课程,还有微信公众号的文章,最终才得以勉强实现,并在这么晚的时间提交作业。虽然算法的思路还算是清晰,整个哈希环上,增加或减少节点,之间的缓存按次序挪到下一个节点处即可。个人觉得陶老师的这个图示很直观。
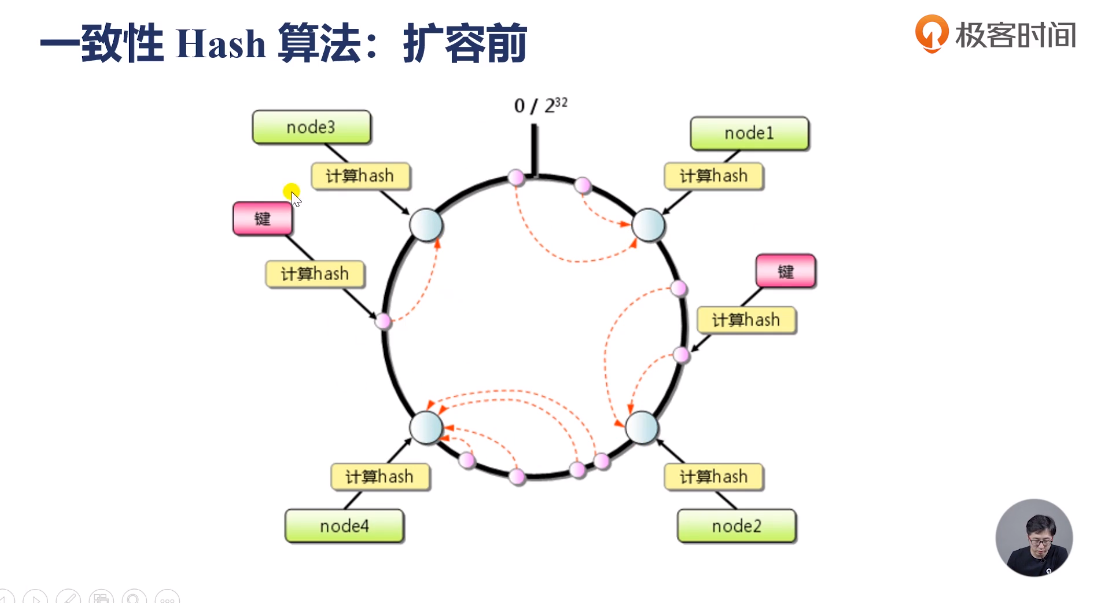
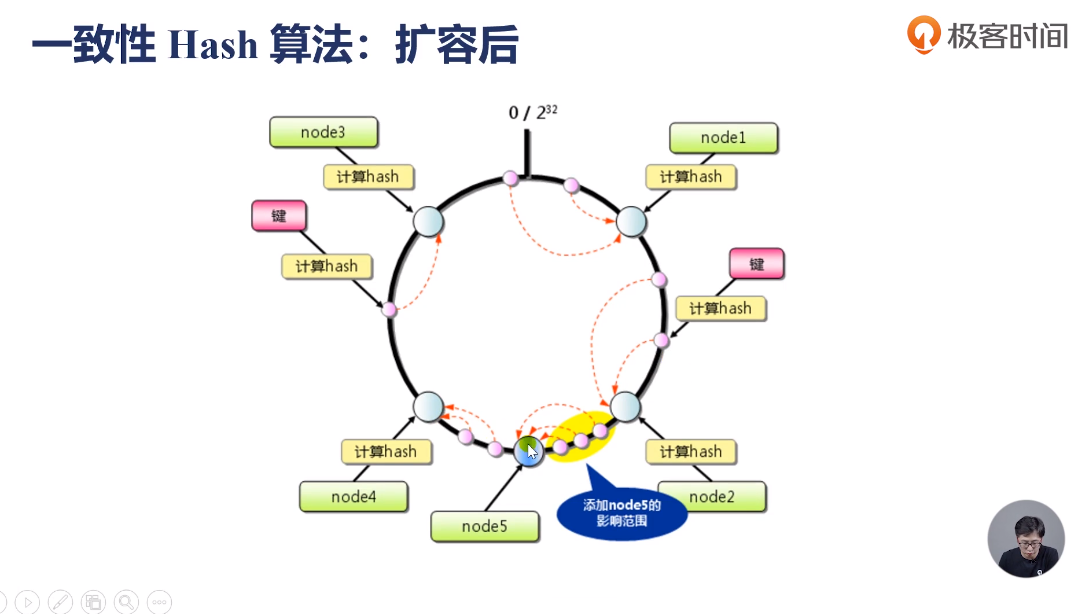
接下来是代码:
缓存服务器实体类:
public class CacheServerNode { private final String socketAddress; public CacheServerNode(String socketAddress) { this.socketAddress = socketAddress; } public String getSocketAddress() { return socketAddress; }}
哈希算法接口:
public interface HashAlgorithm { long hash(final String k);}
哈希算法:
import java.nio.ByteBuffer;import java.nio.ByteOrder;import java.security.MessageDigest;import java.security.NoSuchAlgorithmException;import java.util.zip.CRC32;public enum DefaultHashAlgorithm implements HashAlgorithm { /** * Native hash (String.hashCode()). */ NATIVE_HASH, /** * CRC_HASH as used by the perl API. This will be more consistent both * across multiple API users as well as java versions, but is mostly likely * significantly slower. */ CRC_HASH, /** * FNV hashes are designed to be fast while maintaining a low collision rate. * The FNV speed allows one to quickly hash lots of data while maintaining a * reasonable collision rate. * * @see <a href="http://www.isthe.com/chongo/tech/comp/fnv/">fnv * comparisons</a> * @see <a href="http://en.wikipedia.org/wiki/Fowler_Noll_Vo_hash">fnv at * wikipedia</a> */ FNV1_64_HASH, /** * Variation of FNV. */ FNV1A_64_HASH, /** * 32-bit FNV1. */ FNV1_32_HASH, /** * 32-bit FNV1a. */ FNV1A_32_HASH, /** * MD5-based hash algorithm used by ketama. */ KETAMA_HASH, MURMUR_HASH; private static final long FNV_64_INIT = 0xcbf29ce484222325L; private static final long FNV_64_PRIME = 0x100000001b3L; private static final long FNV_32_INIT = 2166136261L; private static final long FNV_32_PRIME = 16777619; private static MessageDigest md5Digest = null; static { try { md5Digest = MessageDigest.getInstance("MD5"); } catch (NoSuchAlgorithmException e) { throw new RuntimeException("MD5 not supported", e); } } /** * Compute the hash for the given key. * * @return a positive integer hash */ @Override public long hash(final String k) { long rv = 0; int len = k.length(); switch (this) { case NATIVE_HASH: rv = k.hashCode(); break; case CRC_HASH: // return (crc32(shift) >> 16) & 0x7fff; CRC32 crc32 = new CRC32(); crc32.update(KeyUtil.getKeyBytes(k)); rv = (crc32.getValue() >> 16) & 0x7fff; break; case FNV1_64_HASH: // Thanks to pierre@demartines.com for the pointer rv = FNV_64_INIT; for (int i = 0; i < len; i++) { rv *= FNV_64_PRIME; rv ^= k.charAt(i); } break; case FNV1A_64_HASH: rv = FNV_64_INIT; for (int i = 0; i < len; i++) { rv ^= k.charAt(i); rv *= FNV_64_PRIME; } break; case FNV1_32_HASH: rv = FNV_32_INIT; for (int i = 0; i < len; i++) { rv *= FNV_32_PRIME; rv ^= k.charAt(i); } break; case FNV1A_32_HASH: rv = FNV_32_INIT; for (int i = 0; i < len; i++) { rv ^= k.charAt(i); rv *= FNV_32_PRIME; } break; case KETAMA_HASH: byte[] bKey = computeMd5(k); rv = ((long) (bKey[3] & 0xFF) << 24) | ((long) (bKey[2] & 0xFF) << 16) | ((long) (bKey[1] & 0xFF) << 8) | (bKey[0] & 0xFF); break; case MURMUR_HASH: rv = murmurHash(k); break; default: assert false; } return rv & 0xffffffffL; /* Truncate to 32-bits */ } private long murmurHash(String key) { ByteBuffer buf = ByteBuffer.wrap(KeyUtil.getKeyBytes(key)); int seed = 0x1234ABCD; ByteOrder byteOrder = buf.order(); buf.order(ByteOrder.LITTLE_ENDIAN); long m = 0xc6a4a7935bd1e995L; int r = 47; long h = seed ^ (buf.remaining() * m); long k; while (buf.remaining() >= 8) { k = buf.getLong(); k *= m; k ^= k >>> r; k *= m; h ^= k; h *= m; } if (buf.remaining() > 0) { ByteBuffer finish = ByteBuffer.allocate(8).order( ByteOrder.LITTLE_ENDIAN); // for big-endian version, do this first: // finish.position(8-buf.remaining()); finish.put(buf).rewind(); h ^= finish.getLong(); h *= m; } h ^= h >>> r; h *= m; h ^= h >>> r; buf.order(byteOrder); return h; } /** * Get the md5 of the given key. */ public static byte[] computeMd5(String k) { MessageDigest md5; try { md5 = (MessageDigest) md5Digest.clone(); } catch (CloneNotSupportedException e) { throw new RuntimeException("clone of MD5 not supported", e); } md5.update(KeyUtil.getKeyBytes(k)); return md5.digest(); }}
哈希环
import java.util.List;import java.util.Map;import java.util.TreeMap;public class ConsistentHashNodeLocator implements NodeLocator { private final static int VIRTUAL_NODE_SIZE = 12; private final static String VIRTUAL_NODE_SUFFIX = "-"; private volatile TreeMap<Long, CacheServerNode> hashRing; private final HashAlgorithm hashAlg; public ConsistentHashNodeLocator(List<CacheServerNode> nodes, HashAlgorithm hashAlg) { this.hashAlg = hashAlg; this.hashRing = buildConsistentHashRing(hashAlg, nodes); } @Override public CacheServerNode getPrimary(String k) { long hash = hashAlg.hash(k); return getNodeForKey(hashRing, hash); } private CacheServerNode getNodeForKey(TreeMap<Long, CacheServerNode> hashRing, long hash) { /* 向右找到第一个key */ Map.Entry<Long, CacheServerNode> locatedNode = hashRing.ceilingEntry(hash); /* 想象成为一个环,超出尾部取出第一个 */ if (locatedNode == null) { locatedNode = hashRing.firstEntry(); } return locatedNode.getValue(); } private TreeMap<Long, CacheServerNode> buildConsistentHashRing(HashAlgorithm hashAlgorithm, List<CacheServerNode> nodes) { TreeMap<Long, CacheServerNode> virtualNodeRing = new TreeMap<>(); for (CacheServerNode node : nodes) { for (int i = 0; i < VIRTUAL_NODE_SIZE; i++) { // 新增虚拟节点的方式如果有影响,也可以抽象出一个由物理节点扩展虚拟节点的类 virtualNodeRing.put(hashAlgorithm.hash(node.getSocketAddress().toString() + VIRTUAL_NODE_SUFFIX + i), node); } } return virtualNodeRing; }}
节点定位接口
public interface NodeLocator { /** * Get the primary location for the given key. * * @param k the object key * @return the QueueAttachment containing the primary storage for a key */ CacheServerNode getPrimary(String k);}
package cn.remcarpediem.consistenthash;public class StatisticsUtil { //方差s^2=[(x1-x)^2 +...(xn-x)^2]/n public static double variance(Long[] x) { int m = x.length; double sum = 0; for (int i = 0; i < m; i++) {//求和 sum += x[i]; } double dAve = sum / m;//求平均值 double dVar = 0; for (int i = 0; i < m; i++) {//求方差 dVar += (x[i] - dAve) * (x[i] - dAve); } return dVar / m; } //标准差σ=sqrt(s^2) public static double standardDeviation(Long[] x) { int m = x.length; double sum = 0; for (int i = 0; i < m; i++) {//求和 sum += x[i]; } double dAve = sum / m;//求平均值 double dVar = 0; for (int i = 0; i < m; i++) {//求方差 dVar += (x[i] - dAve) * (x[i] - dAve); } return Math.sqrt(dVar / m); }}
mport com.google.common.util.concurrent.AtomicLongMap;import org.junit.Test;import java.util.ArrayList;import java.util.List;import java.util.UUID;public class NodeLocatorTest { private static List<String> ips = new ArrayList<>(50); static { for (int i = 1; i <= 50; i++) { ips.add(String.format("192.168.0.%s", i)); } } /** * 测试节点新增删除后的变化程度 */ @Test public void testNodeAddAndRemove() { List<CacheServerNode> servers = new ArrayList<>(); for (String ip : ips) { servers.add(new CacheServerNode(ip)); } // 构造 100_0000 缓存 List<String> keys = new ArrayList<>(); for (int i = 0; i < 100_0000; i++) { keys.add(UUID.randomUUID().toString()); } for (int index = 10; index <= servers.size(); index++) { AtomicLongMap<CacheServerNode> atomicLongMap = AtomicLongMap.create(); List<CacheServerNode> serverChanged = servers.subList(0, index); NodeLocator nodeLocator = new ConsistentHashNodeLocator(serverChanged, DefaultHashAlgorithm.KETAMA_HASH); for (String key : keys) { CacheServerNode node = nodeLocator.getPrimary(key); atomicLongMap.getAndIncrement(node); } System.out.println(String.format("服务器数目:%d, 标准差:%s", serverChanged.size(), StatisticsUtil.standardDeviation(atomicLongMap.asMap().values().toArray(new Long[]{})))); } }}
实验结果:
服务器数目:10, 标准差:20281.492706406007服务器数目:11, 标准差:18614.617684421835服务器数目:12, 标准差:17859.70210806689服务器数目:13, 标准差:16765.78199539645服务器数目:14, 标准差:14417.187791532944服务器数目:15, 标准差:14167.77614008478服务器数目:16, 标准差:14855.251743911982服务器数目:17, 标准差:13433.657892278708服务器数目:18, 标准差:13288.260571823965服务器数目:19, 标准差:14300.142293424507服务器数目:20, 标准差:14261.868727484487服务器数目:21, 标准差:14661.00541110326服务器数目:22, 标准差:14967.524383044274服务器数目:23, 标准差:13407.09552638774服务器数目:24, 标准差:11908.900724761384服务器数目:25, 标准差:11754.436530944391服务器数目:26, 标准差:11354.83654060706服务器数目:27, 标准差:11155.564944047006服务器数目:28, 标准差:11088.63582636728服务器数目:29, 标准差:10772.03044176388服务器数目:30, 标准差:10567.32368619836服务器数目:31, 标准差:9979.726791578381服务器数目:32, 标准差:9159.913959748748服务器数目:33, 标准差:9299.08377853936服务器数目:34, 标准差:7982.205795167233服务器数目:35, 标准差:7840.779142354648服务器数目:36, 标准差:7079.957525418383服务器数目:37, 标准差:7079.6900431712165服务器数目:38, 标准差:6900.269774123231服务器数目:39, 标准差:6467.352466227541服务器数目:40, 标准差:6516.695938126928服务器数目:41, 标准差:6407.049942438552服务器数目:42, 标准差:6037.666114201412服务器数目:43, 标准差:6315.702547069574服务器数目:44, 标准差:6390.455142289932服务器数目:45, 标准差:6372.179916162962服务器数目:46, 标准差:6270.7003097174975服务器数目:47, 标准差:6003.942253324767服务器数目:48, 标准差:6208.585649235169服务器数目:49, 标准差:6185.0661103718985服务器数目:50, 标准差:5659.331229041113
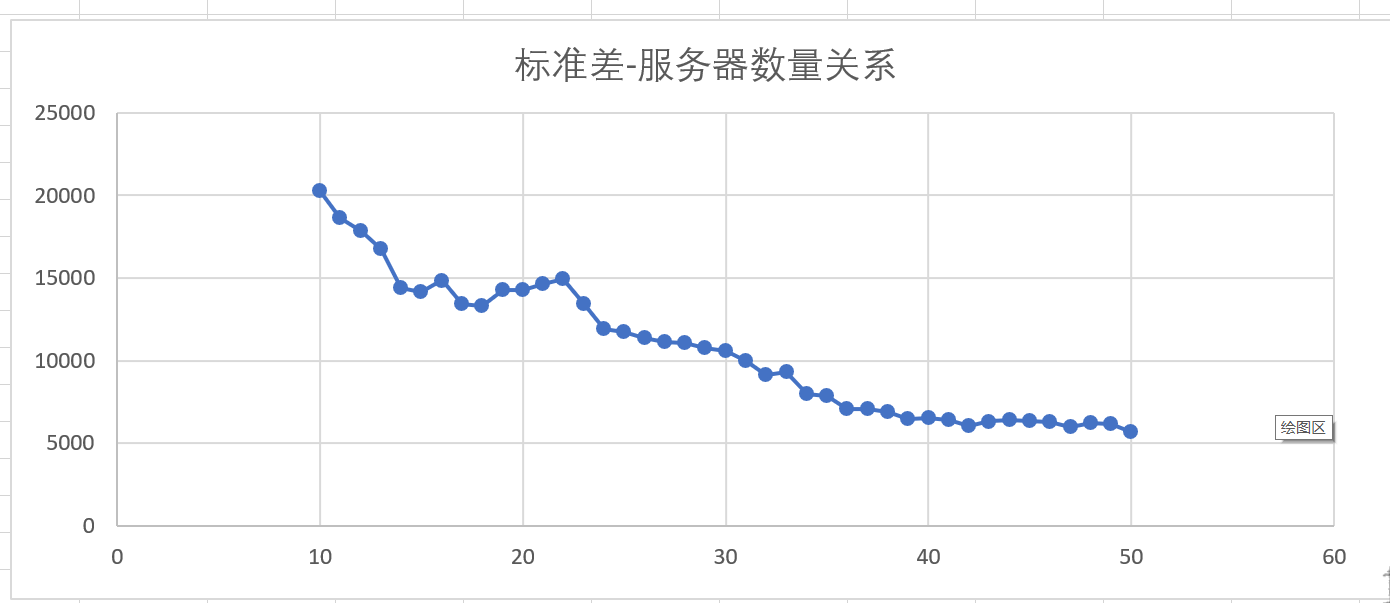
可见,在一段范围内,增加缓存服务器的数量,是有利于提升系统的稳定性能的,且缓存服务器的数量到达一定程度之后,标准差的大小并没有线性下降。所以我个人看来,在实际业务场景中,按业务情况,选用恰当数目的缓存服务器,才是明智之选。
划线
评论
复制
发布于: 2020 年 07 月 08 日 阅读数: 40
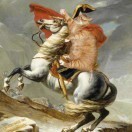
jjn0703
关注
Java工程师/终身学习者 2018.03.26 加入
USTC硕士/健身健美爱好者/Java工程师.
评论